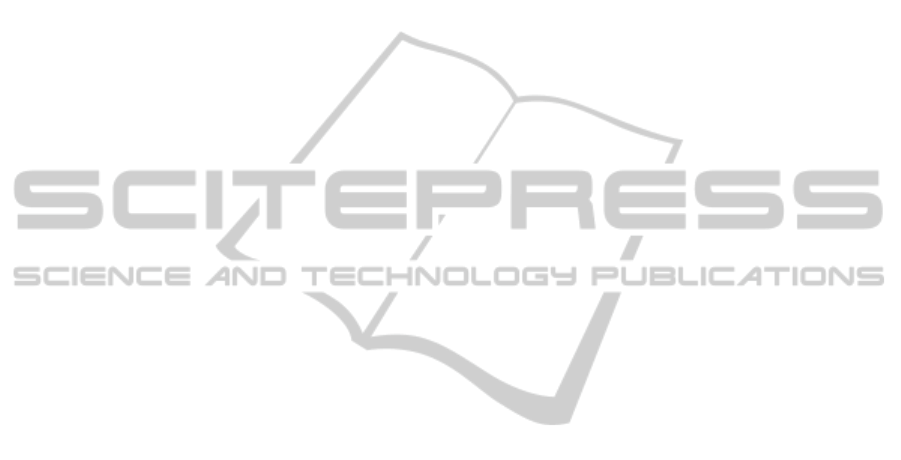
4 MAIN FINDINGS
AND RECOMMENDATIONS
From the point of view of this work intrinsic data
quality, data quality context, data quality
representation and data quality accessibility were
identified as major data quality characteristics. Data
availability, data format, data accuracy and data
accessibility arise as major problems identified,
relating to high-quality data collection on EHRs.
There are solutions to solve such problems like early
recognition of development of those problems and
direct physician entry or physician entry control.
Also, structured encounter forms and well structured
and designed EHRs that include anticipatory
prompts and that allow data linkage and aggregation
to data consumers are part of the solutions available.
A broad use of such systems for the most daily tasks
possible without compromising the goal of
compliant documentation and standard coding use
are also to consider. Other relevant issues are
periodic accuracy monitoring and feedback, better
research methods explanation, evidence-based
guidelines, automated data capture from patient
information systems and others. If attended they can
help reducing data quality problems in order to
improve EHRs suitability for general everyday use.
REFERENCES
Ahima. (2008). Quality Data and Documentation for
EHRs in Physician Practice. Journal of AHIMA, 79,
43-48. Retrieved from AHIMA Body of Knowledge.
Berner, E. S., Moss, J. (2005). Informatics Challenges for
the Impending Patient Information Explosion. J Am
Med Inform Assoc, 12, 614-617.
doi:10.1197/jamia.M1873.
Cruz-Correia, R., Rodrigues, P. P., Freitas, A., Almeida, F.
C., Chen, R., Costa-Pereira, A. (2009). Data Quality
and Integration Issues in Electronic Health Records.
In: HALL, H. V. C. A. (ed.) Information Discovery on
Electronic Health Records. CRC Data Mining and
Knowledge Discovery Series.
de Lusignan, S., Hague, N., Van Vlymen, J. &
Kumarapeli, P. (2006). Routinely-collected general
practice data are complex, but with systematic
processing can be used for quality improvement and
research. Informatics in Primary Care, 14, 59-66.
Retrieved from: http://www.ingentaconnect.com/con
tent/rmp/ipc/2006/00000014/00000001/art00008.
de Lusignan, S. & Van Weel, C. (2006). The use of
routinely collected computer data for research in
primary care: opportunities and challenges. Fam.
Pract., 23, 253-263. doi:10.1093/fampra/cmi106.
Häyrinen, K., Saranto, K., Nykänen, P. (2008). Definition,
structure, content, use and impacts of electronic health
records: A review of the research literature. Int J Med
Inform., 77, 291-304. doi:10.1016/j.ijmedinf.
2007.09.001.
Hersh, W. R. (2002). Medical Informatics: Improving
Health Care Through Information. JAMA, 288, 1955-
1958. doi:10.1001/jama.288.16.1955.
Kaplan, B. & Harris-Salamone, K. D. (2009). Health IT
Success and Failure: Recommendations from
Literature and an AMIA Workshop. Journal of the
American Medical Informatics Association, 16, 291-
299. doi:10.1197/jamia.M2997.
Mcdonald, C. J. (1997). The Barriers to Electronic
Medical Record Systems and How to Overcome
Them. J Am Med Inform Assoc, 4, 213-221. Retrieved
from: http://www.ncbi.nlm.nih.gov/pmc/articles/
PMC61236/
Orfanidis, L., Bamidis, P. D. & Eaglestone, B. (2004).
Data Quality Issues in Electronic Health Records: An
Adaptation Framework for the Greek Health System.
Health Informatics Journal, 10, 23-36.
doi:10.1177/146045804040665.
Pawlson, L. G. (2007). Health Information Technology:
Does It Facilitate Or Hinder Rapid Learning? Health
Aff, 26, w178-180. doi:10.1377/hlthaff.26.2.w178.
Stead, W. W. (2007). Rethinking Electronic Health
Records to Better Achieve Quality and Safety Goals.
Annual Review of Medicine, 58, 35-47.
doi:10.1146/annurev.med.58.061705.144942.
Strong, D. M., Lee, Y. W. & Wang, R. Y. (1997a). 10
Potholes in the Road to Information Quality.
Computer, 30, 38-46. doi:10.1109/2.607057.
Strong, D. M., Lee, Y. W. & Wang, R. Y. (1997b). Data
quality in context. Commun. ACM, 40, 103-110.
doi:http://doi.acm.org/10.1145/253769.253804.
Vaughan, C. (2009). Three Barriers to Effectively Using
Information Stored in EHRs. HealthLeaders Media.
Retrieved from: http://articles.icmcc.org/tag/seconda
ry-data-use/
Weiner, M. G., Lyman, J. A., Murphy, S., Weineer, M.
(2007). Electronic health records: high-quality
electronic data for higher-quality clinical research.
British Computer Society. Retrieved from
IngentaConnect.
HEALTHINF 2011 - International Conference on Health Informatics
454