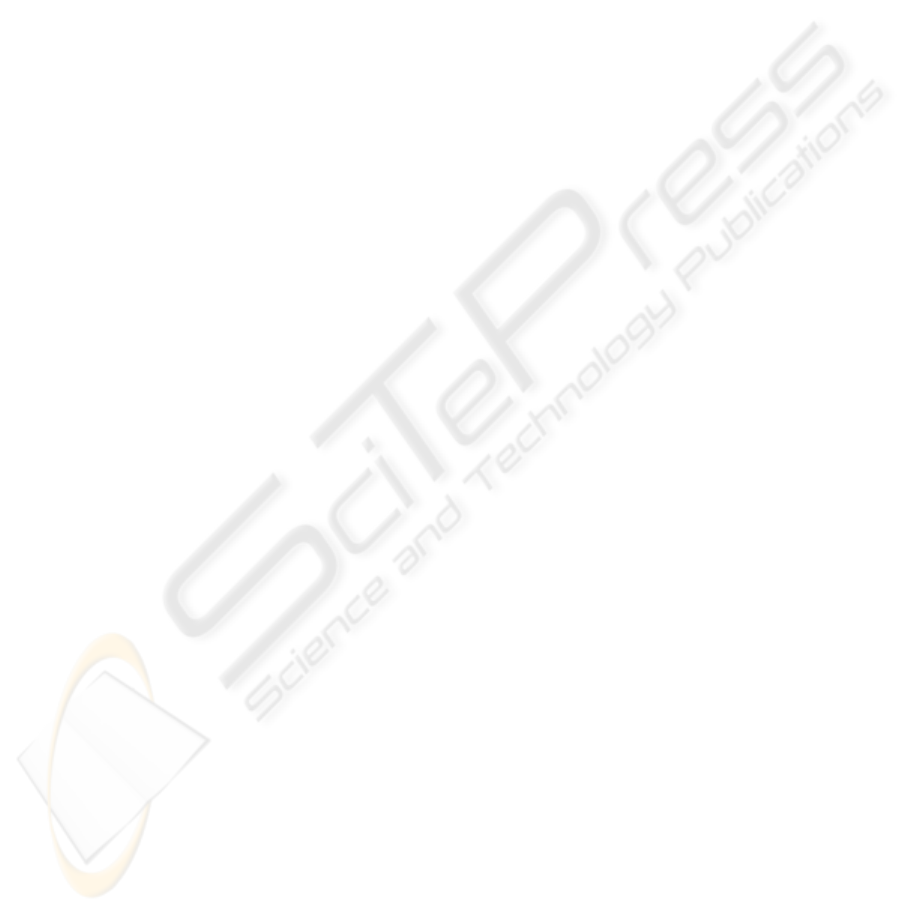
and Extrinsic Motivation. In this validating process,
all the five dimensions show content and construct
validities to some extent. The last two constructs
related to motivation should be deleted as factors if
Stevens’ (2002) guideline is followed since there is
only one loaded item for these two factors. We have
decided to retain these two factors since other
literatures indicate the importance of motivational
factors in learning (Venkatesh 1999, Venkatesh and
Davis, 2000, Venkatesh et al. 2002). The unreliable
items in constructs are eliminated and not considered
in the final solution of the factor analysis. Student
feedback on questions items and the factor analysis
provide
• validity of the dimensions that influence the
effectiveness of online learning
• controls to revalidate under different
experimental setups
• researchers with the valid questionnaire
items to test models or hypotheses under
• different contexts hence facilitating the
analysis of mediating effects on student
experiences and
• quantitative results that may help the
researcher/instructor understand the
dynamics of the online learning tool and
identify critical element to enhance the tool
in helping students perform better in their
learning process
REFERENCES
Agarwal, R., and Karahanna, E., 2000. Time Flies When
You’re Having Fun: Cognitive Absorption and
Beliefs, About Information Technology Usage. MIS
Quarterly, Vol. 24 No. 4, pp. 665-694.
Arkkelin, D., 2003. Putting Prometheus' Feet to the Fire:
Student Evaluations of Prometheus in Relation to
Their Attitudes Towards and Experience With
Computers, Computer Self-Efficacy and Preferred
LearningStyle.
http://faculty.valpo.edu/darkkeli/papers/syllabus03.ht
m (Last accessed on April 17, 2004).
Bergeron, F., Raymond, L. Rivard, S. and Gara, S., 1995.
Determinants of EIS Use: Testing a Behavioral Model.
Decision Support System, Vol. 14, pp. 131– 146.
Daley, B. J., Watkins, K., Williams, W., Courtenay, B.
and Davis, M., 2001. Exploring Llearning in a
Technology-Enhanced Environment. Educational
Technology & Society, Vol. 4, No. 3.
Davies, R. S., 2003. Learner Intent and Online Courses.
The Journal of Interactive Online Learning, Vol. 2,
No. 1.
Davis, F.D., 1989. Perceived Usefulness, Perceived Ease
of Use, and User Acceptance of Information
Technology. MIS Quarterly, Vol. 13, pp. 319-340.
Davis, D. F., Bagozzi, R.P. Warshaw,P.R. 1989.User
Acceptance of Computer Technology: a Comparison
of Two Theoretical Models. Management science,
Vol. 35, No. 8, pp.982-1003.
Davis, F.D., Davis, G.B. and Warshaw, P.R., 1992. User
Acceptance of Computer Technology: A Comparison
of Two Theoretical Models. Management Science,
Vol. 35, No. 12, pp. 982-1003.
Deci, E L., and Ryan, R.M., 1985. Intrinsic Motivation
and Self-determination in Human Behavior. Plenum,
New York.
Eklund, J. and Eklund, P. (1996), “Integrating the Web
and The Teaching of Technology: Cases Across Two
Universities,” Proceedings of the AusWeb96, The
Second Australian WorldWideWeb Conference, Gold
Coast, Australia. Available online at:
http://wwwdev.scu.edu.au/sponsored/ausweb/ausweb9
6/educn/eklund2 (last accessed Feb, 2004).
Feenberg, A., 1987. Computer Conferencing and the
Humanities. Instructional Science, Vol. 16, pp. 169-
186.
Faigley, L. 1990. Subverting the electronic workbook:
Teaching writing using networked computers. In D.
Baker & M. Monenberg (Eds.), The writing teacher as
researcher: Essays in the theory and practice of class-
based research. Portsmouth, NH: Heinemann.
Field, A. (2000). Discovering Statistics using SPSS for
Windows. London – Thousand Oaks –New Delhi:
Sage publications.
Hair, J.F. Jr. , Anderson, R.E., Tatham, R.L., & Black,
W.C. (1998). Multivariate Data Analysis,
(5thEdition). Upper Saddle River, NJ: Prentice Hall.
Irani, T., 1998. Communication Potential, Information
Richness and Attitude: A Study of Computer Mediated
Communication in the ALN Classroom. ALM
Magazine, Vol. 2, No. 1.
Krendl, K. A., and Lieberman, D. A., 1988. Computers
and Learning: A Review of Recent Research. Journal
of Educational Computing Research, Vol. 4, No. 4,
pp. 367-389.
Kum, L. C., 1999. A Study Into Students’ Perceptions of
Web-Based Learning Environment. HERDSA
ANNUAL International Conference, Melbourne, pp.
12-15.
Lowell, R., 2001. The Pew Learning and Technology
Program Newsletter.
http://www.math.hawaii.edu/~dale/pew.html (last
accessed Feb, 2004).
Marzano, R. J. & Pickering, D. J., 1997.
Dimensions of
learning (2
nd
ed.), Alexandria, VA: Association for
Supervision and Curriculum Development.
Picciano, G. A., 2002. Beyond Student Perceptions: Issues
of Interaction, Presence and Performance in an Online
Course. JALN, Vol. 6, No. 1, pp. 21-40.
WEBIST 2005 - E-LEARNING
464