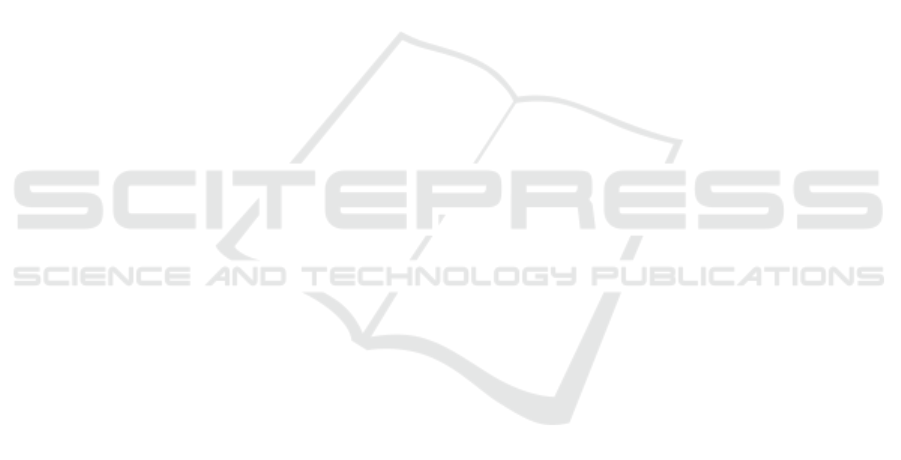
Kharrat, A., Drira, F., Lebourgeois, F., and Garcia, C.
(2023). Toward digits recognition using continual
learning. In IEEE 25th International Workshop on
Multimedia Signal Processing (MMSP).
Kim, C. D., Jeong, J., and Kim, G. (2020). Imbalanced con-
tinual learning with partitioning reservoir sampling. In
Computer Vision–ECCV 2020: 16th European Con-
ference, UK, Proceedings, Part XIII 16. Springer.
Kim, Y. and Rush, A. M. (2016). Sequence-level knowledge
distillation. In Proceedings of the 2016 Conference on
Empirical Methods in Natural Language Processing.
Association for Computational Linguistics.
Kirkpatrick, J., Pascanu, R., Rabinowitz, N., Veness, J.,
Desjardins, G., Rusu, A. A., Milan, K., Quan, J.,
Ramalho, T., Grabska-Barwinska, A., Hassabis, D.,
Clopath, C., Kumaran, D., and Hadsell, R. (2017).
Overcoming catastrophic forgetting in neural net-
works. Proceedings of the National Academy of Sci-
ences.
Lake, B. and Baroni, M. (2018). Generalization with-
out systematicity: On the compositional skills of
sequence-to-sequence recurrent networks. In Pro-
ceedings of the 35th International Conference on Ma-
chine Learning, Proceedings of Machine Learning
Research. PMLR.
Le, H., Vial, L., Frej, J., Segonne, V., Coavoux, M., Lecou-
teux, B., Allauzen, A., Crabb
´
e, B., Besacier, L., and
Schwab, D. (2019). Flaubert: Unsupervised language
model pre-training for french. CoRR.
Lee, S.-W., Kim, J.-H., Jun, J., Ha, J.-W., and Zhang, B.-T.
(2018). Overcoming catastrophic forgetting by incre-
mental moment matching.
Li, J., Monroe, W., Shi, T., Ritter, A., and Jurafsky, D.
(2017). Adversarial learning for neural dialogue gen-
eration. CoRR, abs/1701.06547.
Liu, T., Ungar, L., and Sedoc, J. (2019). Continual learning
for sentence representations using conceptors. In Pro-
ceedings of the 2019 Conference of the North Amer-
ican Chapter of the Association for Computational
Linguistics: Human Language Technologies). Asso-
ciation for Computational Linguistics.
Mai, Z., Li, R., Jeong, J., Quispe, D., Kim, H., and Sanner,
S. (2022). Online continual learning in image classifi-
cation: An empirical survey. Neurocomputing.
McCloskey, M. and Cohen, N. J. (1989). Catastrophic in-
terference in connectionist networks: The sequential
learning problem. In Psychology of learning and mo-
tivation. Elsevier.
Mittal, V., Gangodkar, D., and Pant, B. (2020). Exploring
the dimension of dnn techniques for text categoriza-
tion using nlp. In 2020 6th International Conference
on Advanced Computing and Communication Systems
(ICACCS). IEEE.
Mundt, M., Hong, Y., Pliushch, I., and Ramesh, V. (2023).
A wholistic view of continual learning with deep neu-
ral networks: Forgotten lessons and the bridge to ac-
tive and open world learning. Neural Networks.
Parisi, G. I., Kemker, R., Part, J. L., Kanan, C., and
Wermter, S. (2018). Continual lifelong learning with
neural networks: A review. CoRR.
Qu, H., Rahmani, H., Xu, L., Williams, B., and Liu, J.
(2021). Recent advances of continual learning in com-
puter vision: An overview.
Radford, A., Wu, J., Child, R., Luan, D., Amodei, D., and
Sutskever, I. (2019). Language models are unsuper-
vised multitask learners.
Raffel, C., Shazeer, N., Roberts, A., Lee, K., Narang, S.,
Matena, M., Zhou, Y., Li, W., and Liu, P. J. (2020).
Exploring the limits of transfer learning with a uni-
fied text-to-text transformer. The Journal of Machine
Learning Research.
Ruder, S., Peters, M. E., Swayamdipta, S., and Wolf, T.
(2019). Transfer learning in natural language process-
ing. In Proceedings of the 2019 Conference of the
North American Chapter of the Association for Com-
putational Linguistics, Minneapolis, Minnesota. As-
sociation for Computational Linguistics.
Santos, C. D. and Zadrozny, B. (2014). Learning character-
level representations for part-of-speech tagging. In
Proceedings of the 31st International Conference on
Machine Learning, Proceedings of Machine Learning
Research, Bejing, China. PMLR.
van de Ven, G. M. and Tolias, A. S. (2019). Three scenarios
for continual learning.
Vijay, S. and Priyanshu, A. (2022). Nerda-con: Extending
ner models for continual learning–integrating distinct
tasks and updating distribution shifts.
Wang, C., Luo, F., Li, Y., Xu, R., Huang, F., and Zhang, Y.
(2022). On effectively learning of knowledge in con-
tinual pre-training. arXiv preprint arXiv:2204.07994.
Wang, H., Xiong, W., Yu, M., Guo, X., Chang, S., and
Wang, W. Y. (2019). Sentence embedding alignment
for lifelong relation extraction. In Proceedings of the
2019 Conference of the North American Chapter of
the Association for Computational Linguistics: Hu-
man Language Technologies. Association for Compu-
tational Linguistics.
Wei, H.-R., Huang, S., Wang, R., Dai, X.-y., and Chen, J.
(2019). Online distilling from checkpoints for neural
machine translation. In Proceedings of the 2019 Con-
ference of the North American Chapter of the Associa-
tion for Computational Linguistics: Human Language
Technologies.
Yu, L., Zhang, W., Wang, J., and Yu, Y. (2016). Seqgan:
Sequence generative adversarial nets with policy gra-
dient. CoRR.
ICAART 2024 - 16th International Conference on Agents and Artificial Intelligence
1262