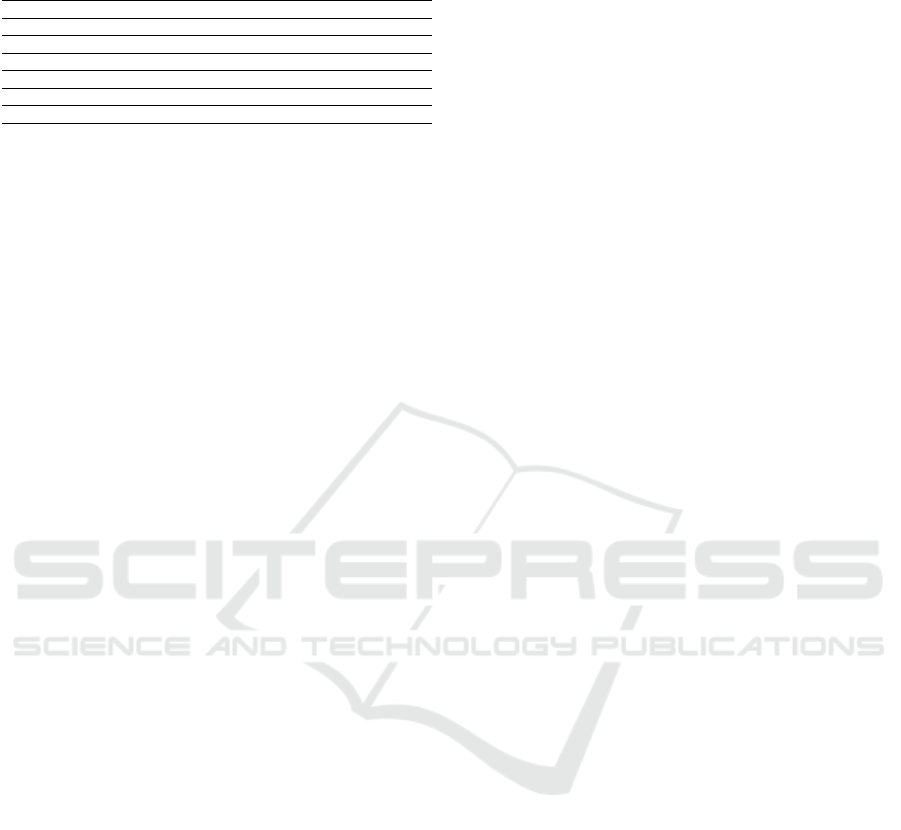
Table 3: Performance metrics of diverse ResNet architec-
tures and the proposed modified ResNet-101.
Model/Parameters Pre Rec F1-score Acc
ResNet-18 0.8921 0.9123 0.9014 0.8898
ResNet-34 0.9040 0.9154 0.9102 0.9001
ResNet-50 0.9308 94.02 0.9405 0.9274
ResNet-152 0.9621 0.9614 0.9652 0.9847
ResNet-101 0.9542 0.9548 0.9760 0.9511
Proposed Mod-ResNet-101 0.9855 0.9855 0.9756 0.9756
Incorporating the segmentation task into the eval-
uation, the proposed modified ResNet-101 demon-
strated its versatility, as depicted in Figure 4. Seg-
mentation demands a nuanced understanding of im-
age features, and the model’s modifications evidently
contributed to its efficacy in delineating regions of in-
terest. The precision and recall metrics in the seg-
mentation task aligned with those of the classification
task, highlighting the model’s consistent performance
across both domains. This dual-task capability proves
instrumental in providing a holistic solution for breast
cancer analysis, where accurate localization and clas-
sification of abnormalities are paramount. While op-
portunities for fine-tuning and further improvement
exist, this study firmly establishes the proposed mod-
ified ResNet-101 as a robust and versatile architec-
ture for comprehensive breast cancer analysis, effec-
tively addressing both classification and segmenta-
tion tasks. By offering a comprehensive solution for
accurate localization and classification of abnormali-
ties, our meticulously designed architecture stands as
a beacon of innovation, paving the way for heightened
precision in medical image analysis and holding im-
mense promise for improving diagnostic outcomes in
breast cancer research and clinical practice.
4 CONCLUSION
Our proposed modified ResNet-101 architecture for
breast cancer classification via breast ultrasound im-
agery has demonstrated superior performance com-
pared to existing approaches. It exhibited height-
ened accuracy, recall, precision, and F1-score met-
rics, signifying its efficacy in precisely discerning
cases of breast cancer. These outcomes outperformed
random forest-based models and rivaled a fully con-
nected depth-wise separable CNN model. These re-
sults underscore the potential of deep learning archi-
tectures in breast cancer classification and provide a
solid groundwork for future investigations. Through
continued refinement and progression of these mod-
els, we can make significant strides in early and pre-
cise breast cancer identification, ultimately leading to
enhanced patient outcomes and reduced disease bur-
den.
Moving forward, we have outlined several areas
for potential improvement in breast cancer classifi-
cation models. A pivotal aspect of our agenda is to
leverage a distinct dataset sourced from our institution
(IGIMS Patna) to validate our model using authentic
clinical data. This endeavor will allow us to evalu-
ate the model’s performance and resilience in a real-
world healthcare setting, offering further substanti-
ation of its adeptness in accurately detecting breast
cancer.
REFERENCES
Aavula, R., Bhramaramba, R., and Ramula, U. S. (2019). A
comprehensive study on data mining techniques used
in bioinformatics for breast cancer prognosis. Journal
of Innovation in Computer Science and Engineering,
9(1):34–39.
Aboutalib, S. S., Mohamed, A. A., Berg, W. A., Zuley,
M. L., Sumkin, J. H., and Wu, S. (2018). Deep learn-
ing to distinguish recalled but benign mammography
images in breast cancer screening. Clinical Cancer
Research, 24(23):5902–5909.
Al-Dhabyani, W., Gomaa, M., Khaled, H., and Fahmy, A.
(2020). Dataset of breast ultrasound images. Data in
brief, 28:104863.
Althobaiti, M. M., Ashour, A. A., Alhindi, N. A., Al-
thobaiti, A., Mansour, R. F., Gupta, D., Khanna, A.,
et al. (2022). Deep transfer learning-based breast can-
cer detection and classification model using photoa-
coustic multimodal images. BioMed Research Inter-
national, 2022.
Chaurasia, V., Pal, S., and Tiwari, B. (2018). Prediction of
benign and malignant breast cancer using data mining
techniques. Journal of Algorithms & Computational
Technology, 12(2):119–126.
Fanizzi, A., Basile, T. M., Losurdo, L., Bellotti, R., Bottigli,
U., Dentamaro, R., Didonna, V., Fausto, A., Massafra,
R., Moschetta, M., et al. (2020). A machine learning
approach on multiscale texture analysis for breast mi-
crocalcification diagnosis. BMC bioinformatics, 21:1–
11.
Ferlay, J., Ervik, M., Lam, F., Colombet, M., Mery, L.,
Pi
˜
neros, M., Znaor, A., Soerjomataram, I., and Bray,
F. (2018). Global cancer observatory: cancer today.
Lyon, France: international agency for research on
cancer, 3(20):2019.
Hakim, A., Prajitno, P., and Soejoko, D. (2021). Micro-
calcification detection in mammography image using
computer-aided detection based on convolutional neu-
ral network. In AIP Conference Proceedings, volume
2346. AIP Publishing.
Jabeen, K., Khan, M. A., Alhaisoni, M., Tariq, U., Zhang,
Y.-D., Hamza, A., Mickus, A., and Dama
ˇ
sevi
ˇ
cius, R.
(2022). Breast cancer classification from ultrasound
images using probability-based optimal deep learning
feature fusion. Sensors, 22(3):807.
BIOSIGNALS 2024 - 17th International Conference on Bio-inspired Systems and Signal Processing
768