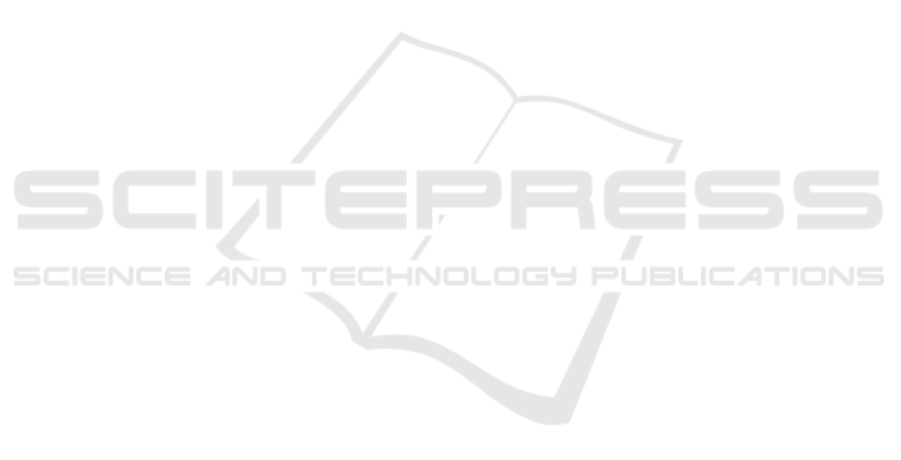
4 CONCLUSION
This article presents an energy minimization-based
framework to improve the depth map for the SFF
method. The framework has been formulated with
AD as a smoothness constraint and a fidelity term,
which incorporates the focus value of the initial depth
to improve the overall structure of the scene. Experi-
ments are conducted with real and synthetic datasets.
For synthetic dataset, both z
o
and z are also compared
with ground truth by measuring RMSE and correla-
tion. The results indicate that the proposed method
can significantly improve the accuracy of the depth
map by removing noise and preserving the structural
details of the scene. The proposed method is also
compared with the L2 regularizer, demonstrating a
substantial 10% improvement in terms of RMSE over
it.
ACKNOWLEDGEMENTS
This work was supported by the Research Council of
Norway through the project CAPSULE under Grant
300031.
REFERENCES
Ahmad, B., Farup, I., and Floor, P. A. (2023a). 3D recon-
struction of gastrointestinal regions using shape-from-
focus. In Fifteenth International Conference on Ma-
chine Vision (ICMV 2022), volume 12701, pages 463–
470. SPIE.
Ahmad, B., Floor, P. A., and Farup, I. (2022). A compar-
ison of regularization methods for near-light-source
perspective shape-from-shading. In 2022 IEEE In-
ternational Conference on Image Processing (ICIP),
pages 3146–3150. IEEE.
Ahmad, B., Floor, P. A., Farup, I., and Hovde, Ø. (2023b).
3D reconstruction of gastrointestinal regions using
single-view methods. IEEE Access.
Ali, U. and Mahmood, M. T. (2021). Robust focus volume
regularization in shape from focus. IEEE Transactions
on Image Processing, 30:7215–7227.
Ali, U. and Mahmood, M. T. (2022). Energy minimization
for image focus volume in shape from focus. Pattern
Recognition, 126:108559.
Di Zenzo, S. (1986). A note on the gradient of a multi-
image. Computer vision, graphics, and image pro-
cessing, 33(1):116–125.
Dogan, H. (2023). A higher performance shape from focus
strategy based on unsupervised deep learning for 3d
shape reconstruction. Multimedia Tools and Applica-
tions, pages 1–24.
He, M., Zhang, J., Shan, S., and Chen, X. (2022). En-
hancing face recognition with self-supervised 3d re-
construction. In Proceedings of the IEEE/CVF Con-
ference on Computer Vision and Pattern Recognition,
pages 4062–4071.
˙
Incetan, K., Celik, I. O., Obeid, A., Gokceler, G. I., Ozy-
oruk, K. B., Almalioglu, Y., Chen, R. J., Mahmood,
F., Gilbert, H., Durr, N. J., et al. (2021). VR-Caps: A
virtual environment for capsule endoscopy. Medical
image analysis, 70:101990.
Moeller, M., Benning, M., Sch
¨
onlieb, C., and Cremers,
D. (2015). Variational depth from focus recon-
struction. IEEE Transactions on Image Processing,
24(12):5369–5378.
Mutahira, H., Ahmad, B., Muhammad, M. S., and Shin,
D. R. (2021a). Focus measurement in color space for
shape from focus systems. IEEE Access, 9:103291–
103310.
Mutahira, H., Muhammad, M. S., Li, M., and Shin, D.-
R. (2021b). A simplified approach using deep neural
network for fast and accurate shape from focus. Mi-
croscopy Research and Technique, 84(4):656–667.
Nayar, S. K. and Nakagawa, Y. (1994). Shape from focus.
IEEE Transactions on Pattern analysis and machine
intelligence, 16(8):824–831.
¨
Ozyes¸il, O., Voroninski, V., Basri, R., and Singer, A.
(2017). A survey of structure from motion*. Acta
Numerica, 26:305–364.
Perona, P. and Malik, J. (1990). Scale-space and edge de-
tection using anisotropic diffusion. IEEE Transac-
tions on pattern analysis and machine intelligence,
12(7):629–639.
Pertuz, S., Puig, D., and Garcia, M. A. (2013). Analysis of
focus measure operators for shape-from-focus. Pat-
tern Recognition, 46(5):1415–1432.
PureMoCo (2017). PureMoCo. Focus-zoom-unit (mo-
torized follow focus) 1:15–2:23. YouTube. https:
//www.youtube.com/watch?v=KFryXjYbTJc. Ac-
cessed: 2020-11-06.
Sapiro, G. and Ringach, D. L. (1996). Anisotropic diffu-
sion of multivalued images with applications to color
filtering. IEEE transactions on image processing,
5(11):1582–1586.
Tschumperl
´
e, D. and Deriche, R. (2005). Vector-valued
image regularization with pdes: A common frame-
work for different applications. IEEE transactions on
pattern analysis and machine intelligence, 27(4):506–
517.
Tseng, C.-Y. and Wang, S.-J. (2014). Shape-from-focus
depth reconstruction with a spatial consistency model.
IEEE Transactions on Circuits and Systems for Video
Technology, 24(12):2063–2076.
Verbin, D. and Zickler, T. (2020). Toward a universal
model for shape from texture. In Proceedings of the
IEEE/CVF Conference on Computer Vision and Pat-
tern Recognition, pages 422–430.
Anisotropic Diffusion for Depth Estimation in Shape from Focus Systems
89