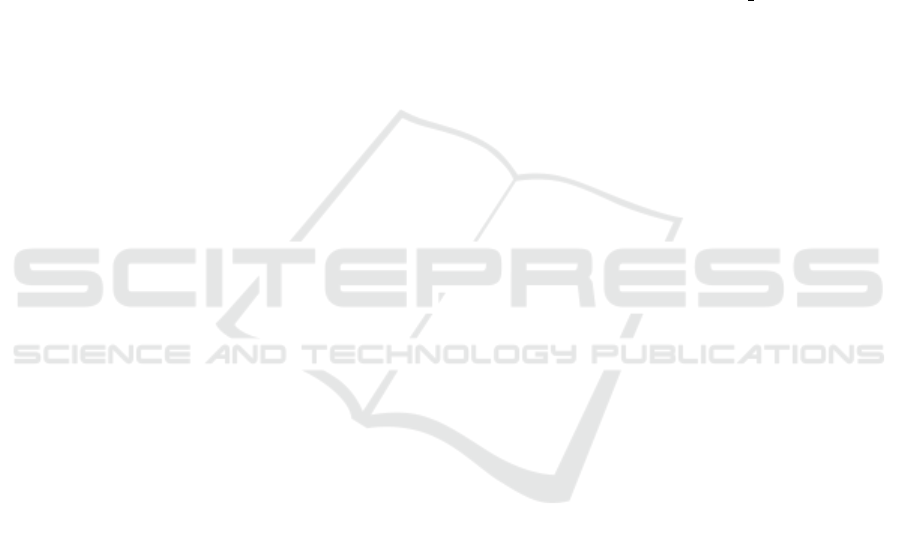
additional refinement in similarity measures. This
paper also proposed predicting the severity levels of
side effects through a multi-class classification ap-
proach. It classified drug interactions into minor (low-
frequency), moderate (medium-frequency), and ma-
jor (high-frequency) levels.
The authors aspire to develop more effective pre-
dictive models using Deep Learning methods, Re-
current Neural Network (RNNs) and their variations
which could significantly contribute to the evolution
of the reasearch work. Future work could also explore
the other existing research to perform comparison on
the same dataset for a more comprehensive evaluation
of model performance.
REFERENCES
Chen, H. and Li, J. (2018). Drugcom: Synergistic discovery
of drug combinations using tensor decomposition. In
2018 IEEE International Conference on Data Mining
(ICDM), pages 899–904. IEEE.
de Albuquerque, M. A., do Nascimento, E. R.,
de Oliveira Barros, K. N. N., and Barros, P.
S. N. (2022). Comparison between similarity coeffi-
cients with application in forest sciences. Research,
Society and Development, 11(2):e48511226046–
e48511226046.
Ferdousi, R., Safdari, R., and Omidi, Y. (2017). Compu-
tational prediction of drug-drug interactions based on
drugs functional similarities. Journal of biomedical
informatics, 70:54–64.
Galeano, D., Li, S., Gerstein, M., and Paccanaro, A. (2020).
Predicting the frequencies of drug side effects. Nature
communications, 11(1):4575.
Han, K., Cao, P., Wang, Y., Xie, F., Ma, J., Yu, M., Wang,
J., Xu, Y., Zhang, Y., and Wan, J. (2022). A review
of approaches for predicting drug–drug interactions
based on machine learning. Frontiers in Pharmacol-
ogy, 12:3966.
Huang, L., Luo, H., Li, S., Wu, F.-X., and Wang, J. (2021).
Drug–drug similarity measure and its applications.
Briefings in Bioinformatics, 22(4):bbaa265.
Huang, L.-C., Wu, X., and Chen, J. Y. (2013). Predicting
adverse drug reaction profiles by integrating protein
interaction networks with drug structures. Proteomics,
13(2):313–324.
Ibrahim, H., El Kerdawy, A. M., Abdo, A., and Eldin, A. S.
(2021). Similarity-based machine learning frame-
work for predicting safety signals of adverse drug–
drug interactions. Informatics in Medicine Unlocked,
26:100699.
Kumari, D., Yannam, P. K. R., Gohel, I. N., Naidu, M. V.
S. S., Arora, Y., Rajita, B., Panda, S., and Christo-
pher, J. (2023). Computational model for breast can-
cer diagnosis using hfse framework. Biomedical Sig-
nal Processing and Control, 86:105121.
LaBute, M. X., Zhang, X., Lenderman, J., Bennion,
B. J., Wong, S. E., and Lightstone, F. C. (2014).
Adverse drug reaction prediction using scores pro-
duced by large-scale drug-protein target docking on
high-performance computing machines. PloS one,
9(9):e106298.
Liu, M., Wu, Y., Chen, Y., Sun, J., Zhao, Z., Chen, X.-
w., Matheny, M. E., and Xu, H. (2012). Large-scale
prediction of adverse drug reactions using chemical,
biological, and phenotypic properties of drugs. Jour-
nal of the American Medical Informatics Association,
19(e1):e28–e35.
Luo, H., Chen, J., Shi, L., Mikailov, M., Zhu, H., Wang, K.,
He, L., and Yang, L. (2011). Drar-cpi: a server for
identifying drug repositioning potential and adverse
drug reactions via the chemical–protein interactome.
Nucleic acids research, 39(suppl 2):W492–W498.
Rajita, B., Tarigopula, P., Ramineni, P., Sharma, A., and
Panda, S. (2023). Application of evolutionary algo-
rithms in social networks: A comparative machine
learning perspective. New Generation Computing,
41(2):401–444.
Seo, S., Lee, T., Kim, M.-h., and Yoon, Y. (2020). Pre-
diction of side effects using comprehensive similarity
measures. BioMed research international, 2020.
Tatonetti, N. P., Ye, P. P., Daneshjou, R., and Altman,
R. B. (2012). Data-driven prediction of drug ef-
fects and interactions. Science translational medicine,
4(125):125ra31–125ra31.
Vilar, S., Uriarte, E., Santana, L., Lorberbaum, T., Hripc-
sak, G., Friedman, C., and Tatonetti, N. P. (2014).
Similarity-based modeling in large-scale prediction of
drug-drug interactions. Nature protocols, 9(9):2147–
2163.
Wu, L., Wen, Y., Leng, D., Zhang, Q., Dai, C., Wang, Z.,
Liu, Z., Yan, B., Zhang, Y., Wang, J., et al. (2022).
Machine learning methods, databases and tools for
drug combination prediction. Briefings in bioinfor-
matics, 23(1):bbab355.
Zhang, W., Zou, H., Luo, L., Liu, Q., Wu, W., and Xiao, W.
(2016). Predicting potential side effects of drugs by
recommender methods and ensemble learning. Neu-
rocomputing, 173:979–987.
Zheng, Y., Peng, H., Ghosh, S., Lan, C., and Li, J. (2019).
Inverse similarity and reliable negative samples for
drug side-effect prediction. BMC bioinformatics,
19(13):91–104.
A Study on Drug Similarity Measures for Predicting Drug-Drug Interactions and Severity Using Machine Learning Techniques
79