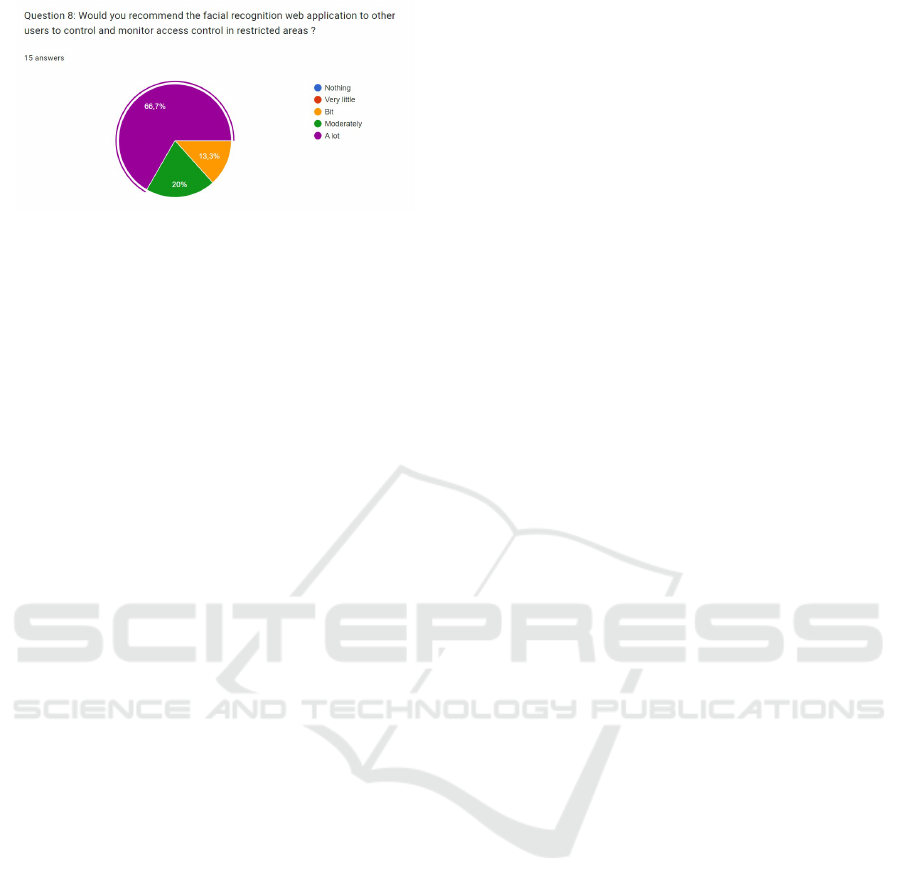
Figure 7: Question 8 pie chart.
6 CONCLUSIONS
The facial recognition system based on neural net-
works in IP cameras for access control of people
in SMEs offers an effective and secure solution to
strengthen security in facilities. Through the accurate
and rapid identification of individuals, this system im-
proves access control efficiency and reduces the risks
associated with unauthorized entry. However, it is im-
portant to note that the successful implementation of
this system requires a proper needs assessment and
careful selection of IP cameras and face database.
In addition, supplementing the system with addi-
tional security measures and performing regular mon-
itoring and maintenance to ensure its proper func-
tioning over time is a way to enhance the final pro-
posed solution, as the development of this project
does. Therefore, 66.7% of the people who partici-
pated in the validation are willing to recommend the
facial recognition system for access control.
7 RECOMMENDATIONS
Before implementing the system, it is important to
evaluate the needs and problems that may be encoun-
tered in the sector to which the solution is to be pro-
vided. On the one hand, it is essential to select high
quality cameras, with adequate resolution and image
capture capacity to ensure accurate identification; it is
also important to ensure optimal integration with the
facial recognition software, as well as its use.
On the other hand, it is essential to perform regular
monitoring and maintenance of this system to ensure
its proper functioning, which involves verifying the
calibration of the cameras, performing software up-
dates, monitoring the database of faces and reviewing
the access logs to detect any anomaly or attempted
security breach in the sector in which this project was
oriented. Finally, it is necessary that future research
be based on this project since it can be oriented not
only to the SME sector, but to any sector where the
most successful solution is a new facial recognition
system, also the sample size can be larger, in order to
increase the metrics that benefit the development of
the project.
REFERENCES
Abou Loume, G., Abana, A. B., Tonye, E., and Kabiena,
Y. (2022). Facial recognition in the opening of a door
using deep learning and a cloud service. International
Journal of Intelligent Systems and Applications in En-
gineering, 10(3):40–45.
Almabdy, S. and Elrefaei, L. (2019). Deep convolutional
neural network-based approaches for face recognition.
Applied Sciences, 9(20):4397.
Araujo, A., P
´
erez, J., and Rodriguez, W. (2018). Apli-
caci
´
on de una red neuronal convolucional para el re-
conocimiento de personas a trav
´
es de la voz. In
Proc. Sexta Conferencia Nacional de Computaci
´
on,
Inform
´
atica y Sistemas, pages 77–81.
Azure, M. (2022). Azure SQL Database: servicio de base
de datos en la nube administrado — Microsoft Azure
— azure.microsoft.com. https://azure.microsoft.com/
es-es/services/sql-database/campaign/#pricing.
Carlos-Roca, L. R., Torres, I. H., and Tena, C. F. (2018).
Facial recognition application for border control. In
2018 International Joint Conference on Neural Net-
works (IJCNN), pages 1–7. IEEE.
Dur
´
an Su
´
arez, J. (2017). Redes neuronales convolucionales
en r: Reconocimiento de caracteres escritos a mano.
Elmahmudi, A. and Ugail, H. (2019). Deep face recogni-
tion using imperfect facial data. Future Generation
Computer Systems, 99:213–225.
Hussain, S. A. and Al Balushi, A. S. A. (2020). A real time
face emotion classification and recognition using deep
learning model. In Journal of physics: Conference
series, volume 1432, page 012087. IOP Publishing.
Hussain, T., Hussain, D., Hussain, I., AlSalman, H., Hus-
sain, S., Ullah, S. S., and Al-Hadhrami, S. (2022). In-
ternet of things with deep learning-based face recog-
nition approach for authentication in control medical
systems. Computational and Mathematical Methods
in Medicine, 2022.
IBM (2022). ¿Qu
´
e son las redes neuronales convolu-
cionales? — IBM — ibm.com. https://www.ibm.com/
es-es/topics/convolutional-neural-networks.
Joshi, A., Kale, S., Chandel, S., and Pal, D. K. (2015). Lik-
ert scale: Explored and explained. British journal of
applied science & technology, 7(4):396.
Junquera-S
´
anchez, J., Cilleruelo, C., De-Marcos, L., and
Martinez-Herr
´
aiz, J.-J. (2021). Access control be-
yond authentication. Security and Communication
Networks, 2021:1–11.
Kocacinar, B., Tas, B., Akbulut, F. P., Catal, C., and Mishra,
D. (2022). A real-time cnn-based lightweight mo-
bile masked face recognition system. Ieee Access,
10:63496–63507.
Access Control Using Facial Recognition with Neural Networks for Restricted Zones
317