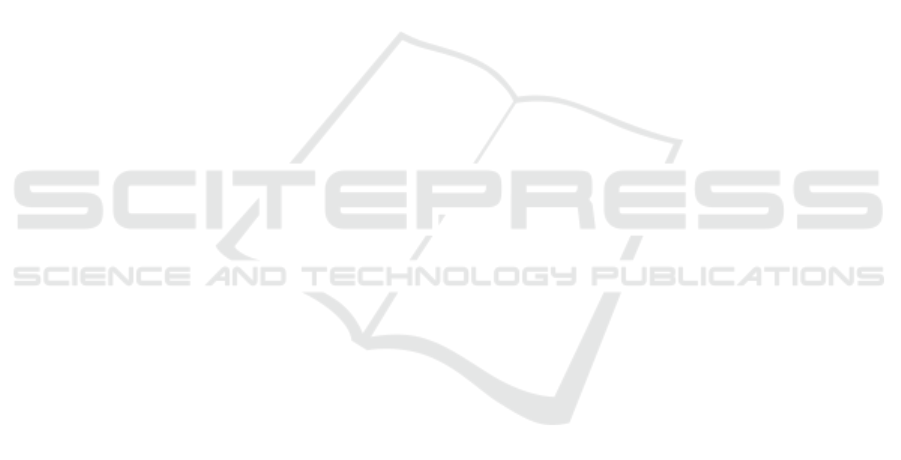
the two rule assessment modes, confirming the pro-
posed framework’s effectiveness. The results of this
study contribute to the field of healthcare analytics by
enhancing our understanding of patterns and associa-
tions within birth data and providing valuable insights
for public health decision-making.
Our contributions are as follows:
• We propose an Effective Rule Evaluation using
Ontology (EREO) framework for evaluating the
level of interestingness.
• We introduce an effective measure called Inte-
grated Rule Information Content using Ontology
(IRIC) for calculating the interestingness score.
Furthermore, the interesting rules are assessed by do-
main experts to ensure correctness, taking into con-
sideration the parameter of clinical relevance.
Overall, this research highlights the importance of
birth data analysis and proposes a novel framework,
EREO, for evaluating the interestingness of associa-
tion rules. By leveraging rare rules and incorporating
expert evaluation, the framework enables the identi-
fication of meaningful patterns in birth data, offering
potential benefits for public health policies, interven-
tions, and improved healthcare outcomes.
The remainder of the paper is structured as fol-
lows: Section 2 discusses the recent literature. Sec-
tion 3 presents the data and methodology for deriving
interesting inferences from US birth data. Section 4
showcases the results along with the interestingness
scores. Finally, Section 5 concludes the paper and
highlights future research directions.
2 LITERATURE REVIEW
The literature surrounding the analysis of birth data
and the evaluation of interesting patterns provides
valuable insights into the existing research and
methodologies in this field.
One area of focus in the literature is the ex-
ploration of association rule mining techniques for
discovering patterns in birth data. Various studies
have employed association rule mining algorithms to
identify associations between maternal characteris-
tics, birth outcomes, and demographic factors. These
studies have shed light on risk factors for adverse
birth outcomes, such as preterm birth and low birth
weight, as well as the impact of socioeconomic fac-
tors on birth outcomes (Bekkar et al., 2020; Griggs
et al., 2020; Robati et al., 2020).
Additionally, researchers have proposed different
measures of interestingness to quantify the signifi-
cance and relevance of discovered patterns. Measures
like support, confidence, and lift have been commonly
used to assess the interestingness of association rules
in birth data. These measures aid in prioritizing and
selecting the most meaningful and relevant patterns
for further analysis and interpretation.
In recent years, the integration of ontologies in
rule evaluation has gained attention in the literature.
Ontologies provide a structured and standardized rep-
resentation of domain knowledge, enabling the incor-
poration of domain expertise into the evaluation pro-
cess. OntoVPA is a commercially available Dialogue
Management System for Virtual Personal Assistants
(VPAs) that utilizes ontologies and ontology-based
rules (Wessel et al., 2019).
The approach for curating Gene Ontology (GO)
data by utilizing association rule mining and intro-
ducing a specificity measure called VICD. The results
demonstrate that VICD scores have stronger correla-
tions with specificity values and lead to more consis-
tent association rules (Shui and Cho, 2016).
(Mattiev and Kavsek, 2020), This study presents
a new method for constructing accurate and compact
classifiers by reducing the number of class associa-
tion rules. The proposed associative classifier selects
strong rules based on overall coverage and achieves
high classification accuracy while generating smaller
rules compared to traditional classifiers.
However, there remains a need for an effective
framework that combines robust rule evaluation tech-
niques, such as ontology-based measures, with ex-
pert evaluation to ensure the correctness and clinical
relevance of the interesting rules. This gap in the
literature highlights the importance of developing a
comprehensive approach for evaluating the interest-
ingness of association rules derived from birth data.
To address this gap, our study proposes the Effec-
tive Rule Evaluation using Ontology (EREO) frame-
work, which leverages the Integrated Rule Informa-
tion Content (IRIC) measure and domain expert eval-
uation. The IRIC measure accounts for the informa-
tion content embedded within the rules, providing a
quantitative indicator of interestingness. The involve-
ment of domain experts ensures the assessment of in-
teresting rules in terms of their clinical relevance and
alignment with current healthcare practices.
By reviewing the existing literature, we have iden-
tified the need for an integrated approach that com-
bines ontology-based measures, expert evaluation,
and the domain-specific nature of interestingness as-
sessment in the context of birth data analysis. Our
study aims to contribute to this field by introducing
the EREO framework and showcasing its effective-
ness in evaluating the interestingness of association
rules derived from US birth data.
EREO: An Effective Rule Evaluation Framework for Discovering Interesting Patterns in US Birth Data and Beyond
569