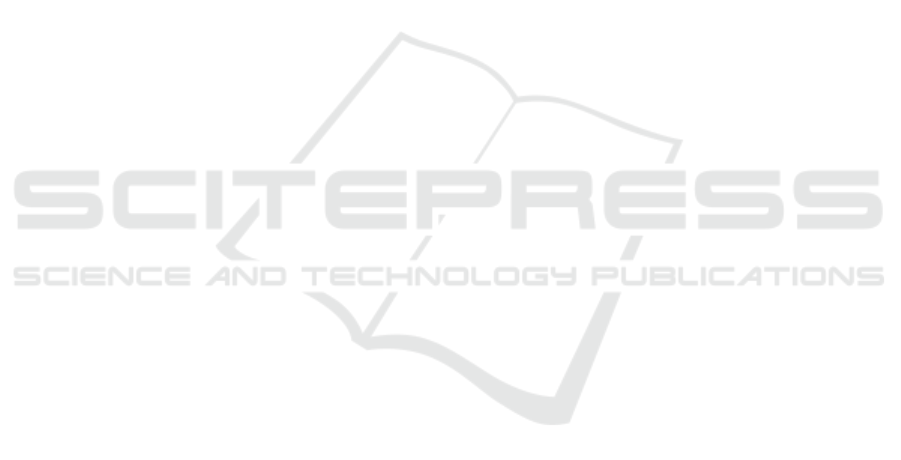
a generalized approach on guiding context learning in
real world applications so adapting to different tasks
would be more efficient.
ACKNOWLEDGEMENTS
The work is supported by NSF via the Partnerships for
Innovation Program (Award #1827505) and the CISE-
MSI Program (Award #1737533), AFOSR Dynamic
Data Driven Applications Systems (Award #FA9550-
21-1-0082), and ODNI via the Intelligence Com-
munity Center for Academic Excellence (IC CAE)
at Rutgers University (Awards #HHM402-19-1-0003
and #HHM402-18-1-0007).
REFERENCES
Ahmetovic, D., Manduchi, R., Coughlan, J. M., and Ma-
scetti, S. (2015). Zebra crossing spotter: Automatic
population of spatial databases for increased safety of
blind travelers. In Proceedings of the International
ACM SIGACCESS Conference on Computers and Ac-
cessibility, pages 251–258.
Cai, Z., Fan, Q., Feris, R. S., and Vasconcelos, N. (2016). A
unified multi-scale deep convolutional neural network
for fast object detection. In Proceedings of the Euro-
pean Conference on Computer Vision, pages 354–370.
Springer.
Chacra, D. A. and Zelek, J. (2022). The topology and lan-
guage of relationships in the visual genome dataset.
In Proceedings of the IEEE/CVF Conference on Com-
puter Vision and Pattern Recognition, pages 4860–
4868.
Chen, C., Liu, M.-Y., Tuzel, O., and Xiao, J. (2017). R-cnn
for small object detection. In Lai, S.-H., Lepetit, V.,
Nishino, K., and Sato, Y., editors, Computer Vision –
ACCV 2016, pages 214–230, Cham. Springer Interna-
tional Publishing.
Chen, Z.-M., Wei, X.-S., Wang, P., and Guo, Y. (2019).
Multi-label image recognition with graph convolu-
tional networks. In Proceedings of the IEEE/CVF
Conference on Computer Vision and Pattern Recog-
nition, pages 5177–5186.
Cheng, M., Zhang, Y., Su, Y.,
´
Alvarez, J. M., and Kong,
H. (2018). Curb detection for road and sidewalk de-
tection. IEEE Transactions on Vehicular Technology,
67:10330–10342.
Clementini, E., Felice, P. D., and Oosterom, P. v. (1993). A
small set of formal topological relationships suitable
for end-user interaction. In International Symposium
on Spatial Databases, pages 277–295. Springer.
Cordts, M., Omran, M., Ramos, S., Rehfeld, T., Enzweiler,
M., Benenson, R., Franke, U., Roth, S., and Schiele,
B. (2016). The cityscapes dataset for semantic urban
scene understanding. In Proceedings of the IEEE/CVF
Conference on Computer Vision and Pattern Recogni-
tion.
Deng, J., Dong, W., Socher, R., Li, L.-J., Li, K., and Fei-
Fei, L. (2009). Imagenet: A large-scale hierarchical
image database. In 2009 IEEE conference on com-
puter vision and pattern recognition, pages 248–255.
Ieee.
Du, Y., Duan, G., and Ai, H. (2012). Context-based text de-
tection in natural scenes. In Proceedings of the IEEE
International Conference on Image Processing, pages
1857–1860. IEEE.
Dvornik, N., Mairal, J., and Schmid, C. (2018). Modeling
visual context is key to augmenting object detection
datasets. In Proceedings of the European Conference
on Computer Vision, pages 364–380.
Egenhofer, M. J. and Franzosa, R. D. (1991). Point-set topo-
logical spatial relations. International Journal of Ge-
ographical Information System, 5(2):161–174.
Fang, Y., Kuan, K., Lin, J., Tan, C., and Chandrasekhar, V.
(2017). Object detection meets knowledge graphs. In
Proceedings of the International Joint Conferences on
Artificial Intelligence.
He, K., Zhang, X., Ren, S., and Sun, J. (2016). Deep resid-
ual learning for image recognition. In Proceedings
of the IEEE/CVF Conference on Computer Vision and
Pattern Recognition, pages 770–778.
Johnson, J., Gupta, A., and Fei-Fei, L. (2018). Image
generation from scene graphs. In Proceedings of the
IEEE/CVF Conference on Computer Vision and Pat-
tern Recognition, pages 1219–1228.
Kipf, T. N. and Welling, M. (2016). Semi-supervised clas-
sification with graph convolutional networks. arXiv
preprint arXiv:1609.02907.
Lee, C.-W., Fang, W., Yeh, C.-K., and Wang, Y.-C. F.
(2018). Multi-label zero-shot learning with structured
knowledge graphs. In Proceedings of the IEEE/CVF
Conference on Computer Vision and Pattern Recogni-
tion, pages 1576–1585.
Leng, J., Ren, Y., Jiang, W., Sun, X., and Wang, Y. (2021).
Realize your surroundings: Exploiting context infor-
mation for small object detection. Neurocomputing,
433:287–299.
Li, Q., Qiao, M., Bian, W., and Tao, D. (2016). Condi-
tional graphical lasso for multi-label image classifi-
cation. In Proceedings of the IEEE/CVF Conference
on Computer Vision and Pattern Recognition, pages
2977–2986.
Li, X., Zhao, F., and Guo, Y. (2014). Multi-label image
classification with a probabilistic label enhancement
model. In Proceedings of the Conference on Uncer-
tainty in Artificial Intelligence, volume 1, pages 1–10.
Lim, J.-S., Astrid, M., Yoon, H.-J., and Lee, S.-I. (2021).
Small object detection using context and attention. In
Proceedings of the International Conference on Arti-
ficial Intelligence in Information and Communication,
pages 181–186.
Lin, T.-Y., Doll
´
ar, P., Girshick, R., He, K., Hariharan, B.,
and Belongie, S. (2017). Feature pyramid networks
for object detection. In Proceedings of the IEEE/CVF
A General Context Learning and Reasoning Framework for Object Detection in Urban Scenes
101