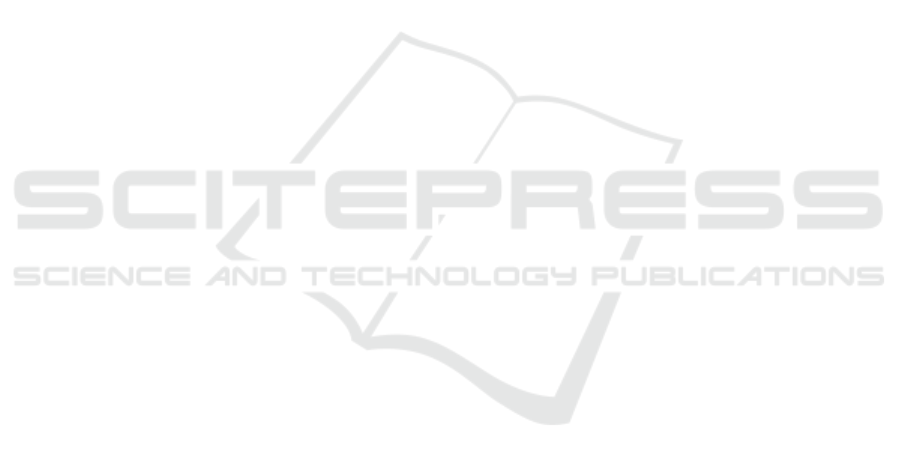
Biber, P. and Straßer, W. (2003). The normal distribu-
tions transform: A new approach to laser scan match-
ing. In Proceedings 2003 IEEE/RSJ International
Conference on Intelligent Robots and Systems (IROS
2003)(Cat. No. 03CH37453), volume 3, pages 2743–
2748. IEEE.
Chetverikov, D., Svirko, D., Stepanov, D., and Krsek, P.
(2002). The trimmed iterative closest point algorithm.
In 2002 International Conference on Pattern Recogni-
tion, volume 3, pages 545–548. IEEE.
Das, A., Servos, J., and Waslander, S. L. (2013). 3d scan
registration using the normal distributions transform
with ground segmentation and point cloud clustering.
In 2013 IEEE international conference on robotics
and automation, pages 2207–2212. IEEE.
Droeschel, D., St
¨
uckler, J., and Behnke, S. (2014). Local
multi-resolution representation for 6d motion estima-
tion and mapping with a continuously rotating 3d laser
scanner. In 2014 IEEE International Conference on
Robotics and Automation (ICRA), pages 5221–5226.
IEEE.
Frei, H., Burri, M., Rems, F., and Risse, E.-A. (2022). A
robust navigation filter fusing delayed measurements
from multiple sensors and its application to spacecraft
rendezvous. Advances of Space Research, Special Is-
sue on Space Environment Management and Space
Sustainability.
Hertzberg, C., Wagner, R., Frese, U., and Schr
¨
oder, L.
(2013). Integrating generic sensor fusion algorithms
with sound state representations through encapsula-
tion of manifolds. Information Fusion, 14(1):57–77.
Hong, H. and Lee, B. H. (2017). Probabilistic normal distri-
butions transform representation for accurate 3d point
cloud registration. In 2017 IEEE/RSJ International
Conference on Intelligent Robots and Systems (IROS),
pages 3333–3338. IEEE.
Koide, K., Yokozuka, M., Oishi, S., and Banno, A. (2021).
Voxelized gicp for fast and accurate 3d point cloud
registration. In 2021 IEEE International Conference
on Robotics and Automation (ICRA), pages 11054–
11059. IEEE.
Lim, H., Hwang, S., Shin, S., and Myung, H. (2020). Nor-
mal distributions transform is enough: Real-time 3d
scan matching for pose correction of mobile robot un-
der large odometry uncertainties. In 2020 20th Inter-
national Conference on Control, Automation and Sys-
tems (ICCAS), pages 1155–1161. IEEE.
Magnusson, M. (2009). The three-dimensional normal-
distributions transform: an efficient representation
for registration, surface analysis, and loop detection.
PhD thesis,
¨
Orebro universitet. Pages 58–65.
Magnusson, M., Nuchter, A., Lorken, C., Lilienthal, A. J.,
and Hertzberg, J. (2009). Evaluation of 3d registration
reliability and speed-a comparison of icp and ndt. In
2009 IEEE International Conference on Robotics and
Automation, pages 3907–3912. IEEE.
N
¨
uchter, A., Elseberg, J., Schneider, P., and Paulus, D.
(2010). Study of parameterizations for the rigid
body transformations of the scan registration prob-
lem. Computer Vision and Image Understanding,
114(8):963–980.
N
¨
uchter, A. and Lingemann, K. (2016). Robotic 3D scan
repository. [Online]. Available: http://kos.informatik.
uni-osnabrueck.de/3Dscans/.
Olive, D. (2022). Prediction and statistical learning. page
344, preprint M-02-006. [Online]. Available: http://
lagrange.math.siu.edu/Olive/slearnbk.htm.
Pang, S., Kent, D., Cai, X., Al-Qassab, H., Morris, D., and
Radha, H. (2018). 3d scan registration based localiza-
tion for autonomous vehicles-a comparison of ndt and
icp under realistic conditions. In 2018 IEEE 88th ve-
hicular technology conference (VTC-Fall), pages 1–5.
IEEE.
Pyrak, M. and Anderson, J. (2022). Performance of
northrop grumman’s mission extension vehicle (mev)
rpo imagers at geo. In Autonomous Systems: Sen-
sors, Processing and Security for Ground, Air, Sea
and Space Vehicles and Infrastructure 2022, volume
12115, pages 64–82. SPIE.
Schulz, C., Hanten, R., and Zell, A. (2018). Efficient map
representations for multi-dimensional normal distri-
butions transforms. In 2018 IEEE/RSJ International
Conference on Intelligent Robots and Systems (IROS),
pages 2679–2686. IEEE.
Segal, A., Haehnel, D., and Thrun, S. (2009). Generalized-
icp. In Robotics: science and systems, volume 2, page
435. Seattle, WA.
Stoyanov, T., Magnusson, M., and Lilienthal, A. J. (2012).
Point set registration through minimization of the l 2
distance between 3d-ndt models. In 2012 IEEE In-
ternational Conference on Robotics and Automation,
pages 5196–5201. IEEE.
Takeuchi, E. and Tsubouchi, T. (2006). A 3-d scan matching
using improved 3-d normal distributions transform for
mobile robotic mapping. In 2006 IEEE/RSJ Interna-
tional Conference on Intelligent Robots and Systems,
pages 3068–3073. IEEE.
Ulas¸, C. and Temeltas¸, H. (2013). 3d multi-layered nor-
mal distribution transform for fast and long range scan
matching. Journal of Intelligent & Robotic Systems,
71(1):85–108.
VISAPP 2023 - 18th International Conference on Computer Vision Theory and Applications
930