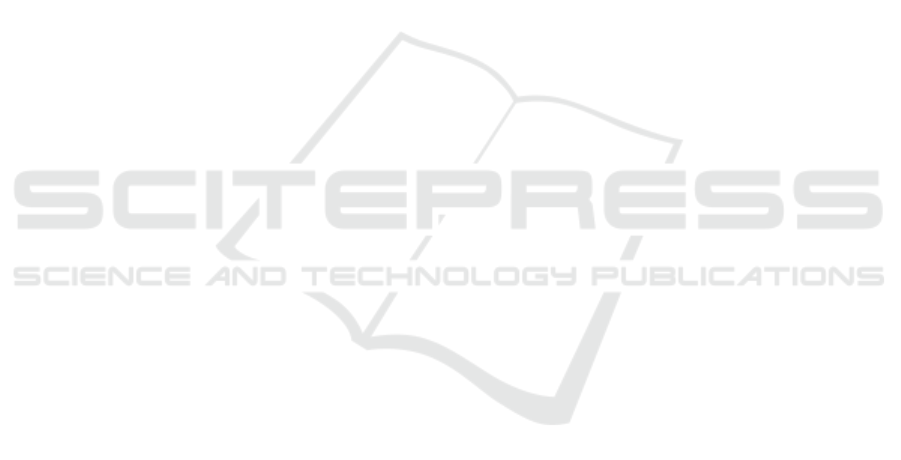
Bauder, R. and Khoshgoftaar, T. (2017). Medicare fraud de-
tection using machine learning methods. In 2017 16th
IEEE International Conference on Machine Learning
and Applications (ICMLA), 18-21 Dec. 2017, pages
858–65. IEEE Computer Society.
Blei, D. M., Ng, A. Y., and Jordan, M. I. (2003). La-
tent dirichlet allocation. J. Mach. Learn. Res.,
3(null):993–1022.
Brys, G., Hubert, M., and Struyf, A. (2004). A robust
measure of skewness. Journal of Computational and
Graphical Statistics, 13(4):996–1017.
Couffinhal, A. and Frankowski, A. (2017). Wasting with in-
tention: Fraud, abuse, corruption and other integrity
violations in the health sector, pages 265–301. OECD
Publishing.
Ekin, T., Ieva, F., Ruggeri, F., and Soyer, R. (2018). Statisti-
cal medical fraud assessment: Exposition to an emerg-
ing field. International Statistical Review, 86(3):379–
402.
Estivill-Castro, V. (2002). Why so many clustering algo-
rithms: A position paper. SIGKDD Explor. Newsl.,
4(1):65–75.
Gee, J. and Button, M. (2015). The financial cost of health-
care fraud. PKF Littlejohn LLP and University of
Portsmouth. Technical report.
Han, J., Kamber, M., and Pei, J. (2011). Data Mining: Con-
cepts and Techniques. Morgan Kaufmann.
Hu, Y., Murray, D. W., Shan, Y., Sutinen, A., Mendis, B.
S. U., and Tang, M. (2011). Prescriber-consumer
social network analysis for risk level re-estimation
based on an asymmetrical rating exchange model. In
Proceedings of the Ninth Australasian Data Mining
Conference, volume 121, pages 111–118. Australian
Computer Society, Inc.
Ivanovi
´
c, M. and Budimac, Z. (2014). An overview of on-
tologies and data resources in medical domains. Ex-
pert Systems with Applications, 41(11):5158–5166.
Ko, J. S., Chalfin, H., Trock, B. J., Feng, Z., Humphreys,
E., Park, S.-W., Carter, H. B., Frick, K. D., and Han,
M. (2015). Variability in medicare utilization and pay-
ment among urologists. Urology, 85:1045–1051.
Konopka, B. M. (2015). Biomedical ontologies — a re-
view. Biocybernetics and Biomedical Engineering,
35(2):75–86.
Koo, T. and Li, M. (2016). A guideline of selecting and
reporting intraclass correlation coefficients for relia-
bility research. Journal of Chiropractic Medicine, 15.
Kose, I., Gokturk, M., and Kilic, K. (2015). An inter-
active machine-learning-based electronic fraud and
abuse detection system in healthcare insurance. Ap-
plied Soft Computing, 36:283–299.
Krumholz, H. M. (2014). Big data and new knowledge
in medicine: The thinking, training, and tools needed
for a learning health system. Health Aff (Millwood),
33(7):1163–70.
Mendis, B. S. U., Murray, D. W., Sutinen, A., Tang, M.,
and Hu, Y. (2011). Enhancing the identification of
anomalous events in Medicare consumer data through
classifier combination. Presented at IWCD6 - 6th In-
ternational Workshop on Chance Discovery as part of
the International Joint Conference on Artificial Intel-
ligence 2011.
Ng, K. S., Shan, Y., Murray, D. W., Sutinen, A., Schwarz,
B., Jeacocke, D., and Farrugia, J. (2010). Detecting
non-compliant consumers in spatio-temporal health
data: A case study from Medicare Australia. In 2010
IEEE International Conference on Data Mining Work-
shops, pages 613–622.
Shan, Y., Jeacocke, D., Murray, D. W., and Sutinen, A.
(2008). Mining medical specialist billing patterns for
health service management. In AusDM ’08: Proceed-
ings of the 7th Australasian Data Mining Conference,
volume 87, pages 105–110. Australian Computer So-
ciety, Inc.
Shan, Y., Murray, D. W., and Sutinen, A. (2009). Discov-
ering inappropriate billings with local density based
outlier detection method. In AusDM ’09: Proceedings
of the Eighth Australasian Data Mining Conference,
volume 101, pages 93–98. Australian Computer Soci-
ety, Inc.
Sivarajah, U., Kamal, M. M., Irani, Z., and Weerakkody, V.
(2017). Critical analysis of big data challenges and
analytical methods. Journal of Business Research,
70:263–286.
Tan, P.-N., Steinbach, M., and Kumar, V. (2005). Introduc-
tion to data mining. New York, NY : Pearson Educa-
tion, Inc., New York, NY.
Tang, M. J., Mendis, B. S. U., Murray, D. W., Hu, Y.,
and Sutinen, A. (2010). Unsupervised fraud detection
in Medicare Australia. Conferences in Research and
Practice in Information Technology Series, 121:103–
110.
Templ, M., Gussenbauer, J., and Filzmoser, P. (2020). Eval-
uation of robust outlier detection methods for zero-
inflated complex data. Journal of Applied Statistics,
47(7):1144–1167.
Thornton, D., Van Capelleveen, G., Poel, M., Hillegersberg,
J., and Mueller, R. (2014). Outlier-based health insur-
ance fraud detection for U.S. Medicaid data. ICEIS
2014 - Proceedings of the 16th International Confer-
ence on Enterprise Information Systems, 2:684–694.
Webber, W., Moffat, A., and Zobel, J. (2010). A similarity
measure for indefinite rankings. ACM Trans. Inf. Syst.,
28(4). Article 20.
Weiss, S. M., Kulikowski, C. A., Galen, R. S., Olsen,
P. A., and Natarajan, R. (2015). Managing healthcare
costs by peer-group modeling. Applied Intelligence,
43(4):752–759.
Yamanishi, K., Takeuchi, J.-I., Williams, G., and Milne, P.
(2004). On-line unsupervised outlier detection using
finite mixtures with discounting learning algorithms.
Data Mining and Knowledge Discovery, 8(3):275–
300.
Yang, J., Xie, M., and Goh, T. (2011). Outlier identifica-
tion and robust parameter estimation in a zero-inflated
poisson model. Journal of Applied Statistics, 38:421–
430.
HEALTHINF 2023 - 16th International Conference on Health Informatics
40