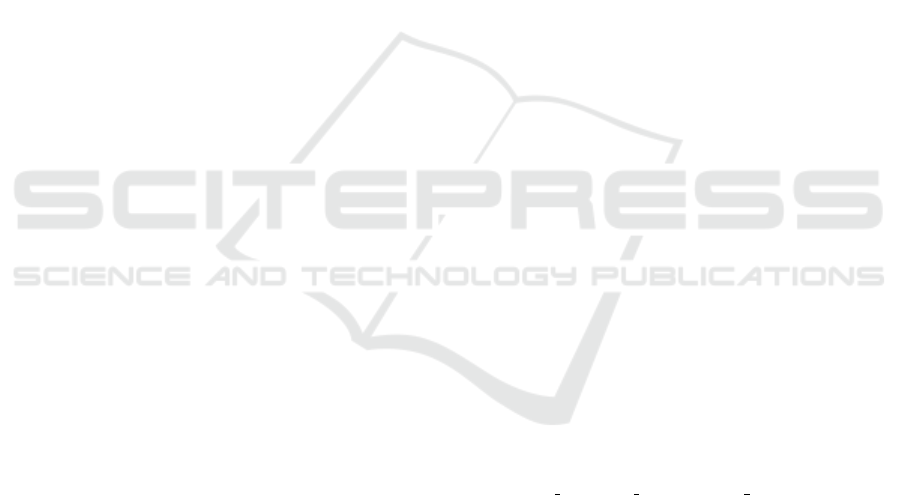
only qualitative conclusions to be drawn regarding the
properties of the electric vehicle market as a whole, as
well as the properties of the trend stability of each of
the time series that were considered. The quantitative
characteristics obtained by this method are averaged
over the entire series. Therefore, to obtain differen-
tiated characteristics of the identified memory, it is
promising to research these time series using fractal
analysis methods, which are based on the sequential
R/S analysis algorithm (Perepelitsa and Maksyshko,
2012).
The use of phase analysis in a two-dimensional
phase spase made it possible to identify the pres-
ence or absence of cyclicity in dynamics at some time
intervals, to evaluate the characteristics of attractors
(quasi-cycles) and their features for each agent in the
Chinese electric automobiles market. The results ob-
tained are the basis for further research in the direc-
tion of a more detailed research of the identified fea-
tures in the dynamics due to decomposition the phase
portrait into quasicycles, determining their character-
istics, analyzing the dynamics of the overall dimen-
sions of quasicycles and their centers of attraction.
The construction of recurrence plots in ρ-
dimensional phase spase and their topological anal-
ysis made it possible to confirm the presence of at-
tractor drift for all agents in the Chinese electric auto-
mobiles market. A gradual change in the parameters
of the behavior of agents was also revealed.
Quantitative analysis of recurrence plots based
on the calculation of measures of complexity of
their structure (in particular, the percent recurrence
(%REC) and the percent determinism (%DET) also
made it possible to confirm the fractal (deterministic)
nature of the nature of the dynamics of sales of elec-
tric vehicles in the Chinese market. It should be noted
that at the moment the input data of the research are
characterized by a short time series. This, obviously,
affects both the possibilities, and the features, and the
results of using the applied methods. However, their
application – each separately and in combination –
provides an opportunity to gain new knowledge about
the characteristics of the dynamics in a new market
that is rapidly developing and has prospects in the
global economy – the market for electric vehicles.
The results of the research can be used to select
relevant forecasting methods and their parameters.
REFERENCES
(2022). Car Sales in China. https://www.chinamobil.ru/eng/
sales/sales.
BYD North America (2022). Bus: BYD Motors - BYD
USA. https://en.byd.com/bus/bus-byd-motors.
CAAM (2022). China Association of Automobile Manu-
facturers (CAAM). http://en.caam.org.cn/.
Chery (2022). Introduction. https://www.
cheryinternational.com/pc/aboutchery/introduction/.
Derbentsev, V., Velykoivanenko, H., and Datsenko, N.
(2019). Machine learning approach for fore-
casting cryptocurrencies time series. Neiro-
Nechitki Tekhnolohii Modelyuvannya v Ekonomitsi,
2019(8):65–93.
Dhakal, T. and Min, K.-S. (2021). Macro Study of
Global Electric Vehicle Expansion. Foresight and STI
Governance, 15(1):67–73. https://doi.org/10.17323/
2500-2597.2021.1.67.73.
Dingab, S. and Li, R. (2021). Forecasting the sales and
stock of electric vehicles using a novel self-adaptive
optimized grey model. Engineering Applications of
Artificial Intelligence, 100:104148. https://doi.org/10.
1016/j.engappai.2020.104148.
Eckmann, J.-P., Kamphorst, S. O., and Ruelle, D. (1987).
Recurrence Plots of Dynamical Systems. Euro-
physics Letters, 4(9):973–977. https://doi.org/10.
1209/0295-5075/4/9/004.
Ensslen, A., Will, C., and Jochem, P. (2019). Simulating
Electric Vehicle Diffusion and Charging Activities in
France and Germany. World Electr. Veh. J., 10(4):73.
https://doi.org/10.3390/wevj10040073.
Faggini, M. (2014). Chaotic time series analysis in
economics: Balance and perspectives. Chaos:
An Interdisciplinary Journal of Nonlinear Science,
24(4):042101. https://doi.org/10.1063/1.4903797.
Geely (2022). Overview : Geely Global. http://global.geely.
com/overview.
Hegger, R. and Kantz, H. (1999). Practical implementation
of nonlinear time series methods: The TISEAN pack-
age. Chaos: An Interdisciplinary Journal of Nonlin-
ear Science, 9(2):413–435. https://doi.org/10.1063/1.
166424.
Jin, L. and He, H. (2019). Comparison of the electric car
market in China and the United States. Working paper
2019-10, International Council on Clean Transporta-
tion. https://theicct.org/wp-content/uploads/2021/06/
ICCT
US-China EV-mkt-comp 20190523.pdf.
Jochem, P., J., J., Vilchez, G., Ensslen, A., Sch
¨
auble, J.,
and Fichtner, W. (2018). Methods for forecasting the
market penetration of electric drivetrains in the pas-
senger car market. Transport Reviews, 38(3):322–348.
https://doi.org/10.1080/01441647.2017.1326538.
Kennel, M. B., Brown, R., and Abarbanel, H. D. I.
(1992). Determining embedding dimension for phase-
space reconstruction using a geometrical construction.
Phys. Rev. A, 45(6):3403. https://doi.org/10.1103/
PhysRevA.45.3403.
Kmytiuk, T. and Majore, G. (2021). Time series forecasting
of agricultural product prices using Elman and Jordan
recurrent neural networks. Neiro-Nechitki Tekhnolohii
Modelyuvannya v Ekonomitsi, 2021(10):67–85.
Maksyshko, N., Vasylieva, O., Kozin, I., and Perepelitsa,
V. (2020). Comparative analysis of the attractive-
M3E2 2022 - International Conference on Monitoring, Modeling Management of Emergent Economy
194