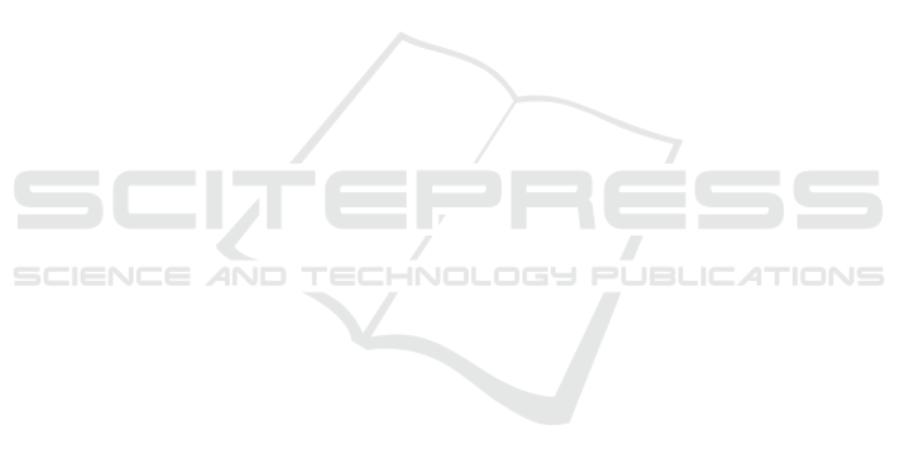
lenges toward responsible AI. Information Fusion,
58:82–115.
Borlea, I.-D., Precup, R.-E., Borlea, A.-B., and Iercan, D.
(2021). A unified form of fuzzy C-means and K-
means algorithms and its partitional implementation.
Knowledge-Based Systems, 214:106731.
Chakraborty, D., Alam, A., Chaudhuri, S., Bas¸a
˘
gao
˘
glu, H.,
Sulbaran, T., and Langar, S. (2021). Scenario-based
prediction of climate change impacts on building cool-
ing energy consumption with explainable artificial in-
telligence. Applied Energy, 291:116807.
Chen, T. and Guestrin, C. (2016). XGBoost: A scalable
tree boosting system. In Proceedings of the 22nd
ACM SIGKDD International Conference on Knowl-
edge Discovery and Data Mining, pages 785–794.
Du, Z., Fan, B., Jin, X., and Chi, J. (2014). Fault detection
and diagnosis for buildings and HVAC systems using
combined neural networks and subtractive clustering
analysis. Building and Environment, 73:1–11.
Du, Z. and Jin, X. (2008). Multiple faults diagnosis for
sensors in air handling unit using Fisher discrimi-
nant analysis. Energy Conversion and Management,
49(12):3654–3665.
Fan, C., Xiao, F., Yan, C., Liu, C., Li, Z., and Wang, J.
(2019). A novel methodology to explain and evalu-
ate data-driven building energy performance models
based on interpretable machine learning. Applied En-
ergy, 235:1551–1560.
Gao, Y. and Ruan, Y. (2021). Interpretable deep learn-
ing model for building energy consumption prediction
based on attention mechanism. Energy and Buildings,
252:111379.
Gunning, D. (2017). Explainable artificial intelligence
(XAI). Technical report, Defense Advanced
Research Projects Agency. [Online] Avail-
able https://www.darpa.mil/program/explainable-
artificial-intelligence, Accessed January 27, 2022.
Han, H., Gu, B., Hong, Y., and Kang, J. (2011). Automated
FDD of multiple-simultaneous faults (MSF) and the
application to building chillers. Energy and Buildings,
43(9):2524–2532.
Houz
´
e,
´
E., Dessalles, J.-L., Diaconescu, A., Menga, D., and
Schumann, M. (2021). A decentralized explanatory
system for intelligent cyber-physical systems. In Lec-
ture Notes in Networks and Systems, pages 719–738.
Springer International Publishing.
Hripcsak, G. and Rothschild, A. S. (2005). Agreement,
the F-measure, and reliability in information retrieval.
Journal of the American Medical Informatics Associ-
ation, 12(3):296–298.
Li, G., Yao, Q., Fan, C., Zhou, C., Wu, G., Zhou, Z., and
Fang, X. (2021a). An explainable one-dimensional
convolutional neural networks based fault diagnosis
method for building heating, ventilation and air con-
ditioning systems. Building and Environment, page
108057.
Li, G., Yao, Q., Fan, C., Zhou, C., Wu, G., Zhou, Z., and
Fang, X. (2021b). An explainable one-dimensional
convolutional neural networks based fault diagno-
sis method for building heating, ventilation and air
conditioning systems. Building and Environment,
203:108057.
Liao, H., Cai, W., Cheng, F., Dubey, S., and Rajesh, P. B.
(2021). An online data-driven fault diagnosis method
for air handling units by rule and convolutional neural
networks. Sensors, 21(13):4358.
Lundberg, S. M. and Lee, S.-I. (2017). A unified approach
to interpreting model predictions. Advances in Neural
Information Processing Systems, 30.
Machlev, R., Heistrene, L., Perl, M., Levy, K. Y., Belikov,
J., Mannor, S., and Levron, Y. (2022). Explainable
artificial intelligence (XAI) techniques for energy and
power systems: review, challenges and opportunities.
Energy and AI, 9:100169.
Machlev, R., Perl, M., Belikov, J., Levy, K. Y., and Lev-
ron, Y. (2021). Measuring explainability and trustwor-
thiness of power quality disturbances classifiers using
explainable artificial intelligence (XAI). IEEE Trans-
actions on Industrial Informatics.
Madhikermi, M., Malhi, A. K., and Fr
¨
amling, K. (2019).
Explainable artificial intelligence based heat recycler
fault detection in air handling unit. In Lecture Notes
in Computer Science, pages 110–125. Springer Inter-
national Publishing.
Miller, C. (2019). What’s in the box?! Towards explainable
machine learning applied to non-residential building
smart meter classification. Energy and Buildings,
199:523–536.
Mirnaghi, M. S. and Haghighat, F. (2020). Fault detection
and diagnosis of large-scale HVAC systems in build-
ings using data-driven methods: A comprehensive re-
view. Energy and Buildings, page 110492.
Srinivasan, S., Arjunan, P., Jin, B., Sangiovanni-Vincentelli,
A. L., Sultan, Z., and Poolla, K. (2021). Explainable
AI for chiller fault-detection systems: Gaining human
trust. Computer, 54(10):60–68.
Tsoka, T., Ye, X., Chen, Y., Gong, D., and Xia, X.
(2021). Building energy performance certificate la-
belling classification based on explainable artificial in-
telligence. In Neural Computing for Advanced Appli-
cations, pages 181–196. Springer Singapore.
Upadhyay, P. K. and Nagpal, C. (2020). Wavelet based per-
formance analysis of SVM and RBF kernel for classi-
fying stress conditions of sleep EEG. SCIENCE AND
TECHNOLOGY, 23(3):292–310.
Wang, S. and Xiao, F. (2004). AHU sensor fault diagnosis
using principal component analysis method. Energy
and Buildings, 36(2):147–160.
Wenninger, S., Kaymakci, C., and Wiethe, C. (2022). Ex-
plainable long-term building energy consumption pre-
diction using QLattice. Applied Energy, 308:118300.
Xiao, F. and Wang, S. (2009). Progress and methodolo-
gies of lifecycle commissioning of HVAC systems to
enhance building sustainability. Renewable and Sus-
tainable Energy Reviews, 13(5):1144–1149.
Explainable AI based Fault Detection and Diagnosis System for Air Handling Units
279