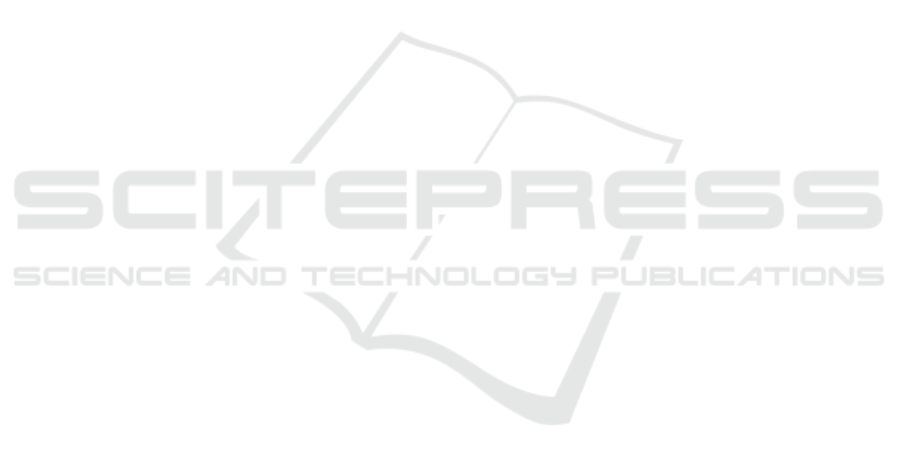
REFERENCES
Amme, J., Pleßmann, G., B
¨
uhler, J., H
¨
ulk, L., K
¨
otter, E.,
and Schwaegerl, P. (2018). The ego grid model: An
open-source and open-data based synthetic medium-
voltage grid model for distribution power supply sys-
tems. In Journal of Physics: Conference Series, vol-
ume 977, page 012007. IOP Publishing.
Balduin, S., Tr
¨
oschel, M., and Lehnhoff, S. (2019). Towards
domain-specific surrogate models for smart grid co-
simulation. Energy Informatics, 2(1):1–19.
Balduin, S., Westermann, T., and Puiutta, E. (2020). Eval-
uating different machine learning techniques as sur-
rogate for low voltage grids. Energy Informatics,
3(1):1–12.
Benesty, J., Chen, J., Huang, Y., and Cohen, I. (2009).
Pearson correlation coefficient. In Noise reduction in
speech processing, pages 37–40. Springer.
Blank, M. (2015). Reliability Assessment of Coalitions for
the Provision of Ancillary Services. PhD thesis, Uni-
versit
¨
at Oldenburg.
Blank, M., Breithaupt, T., Bremer, J., Dammasch, A.,
Garske, S., Klingenberg, T., Koch, S., L
¨
unsdorf, O.,
Niesse, A., Scherfke, S., Hofmann, L., and Sonnen-
schein, M. (2015). Smart nord final report. Technical
report, Uni Hannover.
Bremer, J. and Lehnhoff, S. (2018). Unfolding ensemble
training sets for improved support vector decoders in
energy management. In ICAART (2), pages 322–329.
Cai, D., Shi, D., and Chen, J. (2013). Probabilistic load flow
computation with polynomial normal transformation
and latin hypercube sampling. IET Generation, Trans-
mission & Distribution, 7(5):474–482.
Chen, P., Chen, Z., and Bak-Jensen, B. (2008). Probabilistic
load flow: A review. In 2008 Third International Con-
ference on Electric Utility Deregulation and Restruc-
turing and Power Technologies, pages 1586–1591.
IEEE.
Dean, A. and Voss, D. (1999). Design and analysis of ex-
periments. Springer.
Diao, R., Wang, Z., Shi, D., Chang, Q., Duan, J., and
Zhang, X. (2019). Autonomous voltage control for
grid operation using deep reinforcement learning. In
2019 IEEE Power & Energy Society General Meeting
(PESGM), pages 1–5. IEEE.
Gerster, J., Lehnhoff, S., Sarstedt, M., Hofmann, L., and
Veith, E. M. (2021). Comparison of random sampling
and heuristic optimization-based methods for deter-
mining the flexibility potential at vertical system in-
terconnections. In 2021 IEEE PES Innovative Smart
Grid Technologies Europe (ISGT Europe), pages 1–6.
IEEE.
Guha, N., Wang, Z., Wytock, M., and Majumdar, A. (2019).
Machine learning for ac optimal power flow. arXiv
preprint arXiv:1910.08842.
Hasan, F., Kargarian, A., and Mohammadi, A. (2020). A
survey on applications of machine learning for opti-
mal power flow. In 2020 IEEE Texas Power and En-
ergy Conference (TPEC), pages 1–6.
Huang, Y., Chen, S., Chen, Z., Hu, W., and Huang, Q.
(2020). Improved probabilistic load flow method
based on d-vine copulas and latin hypercube sampling
in distribution network with multiple wind genera-
tors. IET Generation, Transmission & Distribution,
14(5):893–899.
H
¨
ulk, L., Wienholt, L., Cußmann, I., M
¨
uller, U. P., Matke,
C., and K
¨
otter, E. (2017). Allocation of annual elec-
tricity consumption and power generation capacities
across multiple voltage levels in a high spatial reso-
lution. International Journal of Sustainable Energy
Planning and Management, 13:79–92.
Lei, X., Yang, Z., Yu, J., Zhao, J., Gao, Q., and Yu, H.
(2020). Data-driven optimal power flow: A physics-
informed machine learning approach. IEEE Transac-
tions on Power Systems, 36(1):346–354.
Pan, X., Zhao, T., and Chen, M. (2019). Deepopf: Deep
neural network for dc optimal power flow. In 2019
IEEE International Conference on Communications,
Control, and Computing Technologies for Smart Grids
(SmartGridComm), pages 1–6. IEEE.
Powell, L. (2004). Power system load flow analysis. Mc-
Graw Hill professional.
Sklar, A. (1959). Fonctions d
´
e repartition
`
a n dimension
et leurs marges. Paris: Publications de l’Institut de
statistique de l’Universit
´
e de Paris, 8:229–231.
Spalthoff, C., Sarajlic, D., Kittl, C., Drauz, S., Kneiske, T.,
Rehtanz, C., and Braun, M. (2019). Simbench: Open
source time series of power load, storage and genera-
tion for the simulation of electrical distribution grids.
In International ETG-Congress 2019; ETG Sympo-
sium, pages 1–6. VDE.
Thayer, B. L. and Overbye, T. J. (2020). Deep reinforce-
ment learning for electric transmission voltage con-
trol. In 2020 IEEE Electric Power and Energy Con-
ference (EPEC), pages 1–8. IEEE.
Thurner, L., Scheidler, A., Sch
¨
afer, F., Menke, J., Dol-
lichon, J., Meier, F., Meinecke, S., and Braun, M.
(2018). pandapower — an open-source python tool for
convenient modeling, analysis, and optimization of
electric power systems. IEEE Transactions on Power
Systems, 33(6):6510–6521.
Whittaker, J. (2009). Graphical models in applied multi-
variate statistics. Wiley Publishing.
Wiese, F., Schlecht, I., Bunke, W.-D., Gerbaulet, C., Hirth,
L., Jahn, M., Kunz, F., Lorenz, C., M
¨
uhlenpfordt, J.,
Reimann, J., and Schill, W.-P. (2019). Open power
system data – frictionless data for electricity system
modelling. Applied Energy, 236:401–409.
Zamzam, A. S. and Baker, K. (2020). Learning optimal so-
lutions for extremely fast ac optimal power flow. In
2020 IEEE International Conference on Communica-
tions, Control, and Computing Technologies for Smart
Grids (SmartGridComm), pages 1–6. IEEE.
SIMULTECH 2022 - 12th International Conference on Simulation and Modeling Methodologies, Technologies and Applications
326