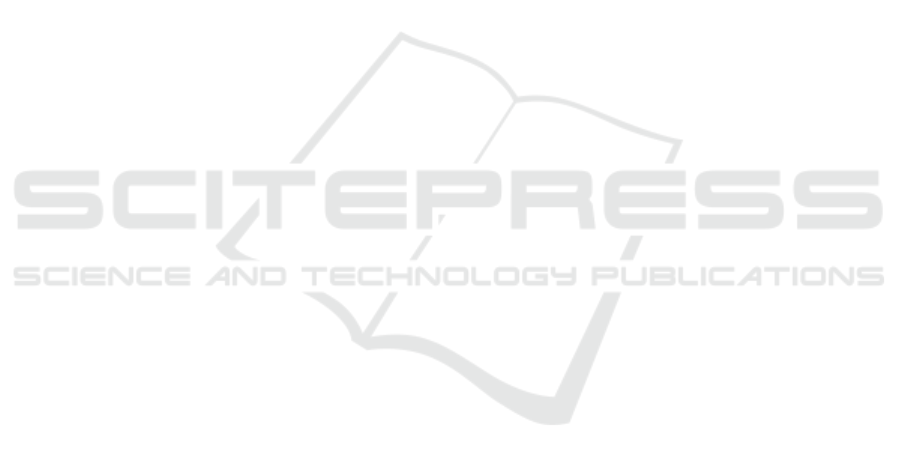
Bellatreche, L., Cuzzocrea, A., and Benkrid, S. (2010).
F&A: A methodology for effectively and efficiently
designing parallel relational data warehouses on het-
erogenous database clusters. In DAWAK 2010, Bil-
bao, Spain, August/September 2010, pages 89–104.
Springer.
Bonifati, A. and Cuzzocrea, A. (2007). Efficient fragmen-
tation of large XML documents. In DEXA 2007, Re-
gensburg, Germany, September 3-7, 2007, pages 539–
550. Springer.
Braun, P., Cuzzocrea, A., Keding, T. D., Leung, C. K., Paz-
dor, A. G. M., and Sayson, D. (2017). Game data
mining: Clustering and visualization of online game
data in cyber-physical worlds. In KES 2017, Mar-
seille, France, 6-8 September 2017, pages 2259–2268.
Elsevier.
Cannataro, M., Cuzzocrea, A., Mastroianni, C., Ortale, R.,
and Pugliese, A. (2002a). Modeling adaptive hyper-
media with an object-oriented approach and XML. In
WebDyn@WWW 2002, Honululu, HW, USA, May 7,
2002, pages 35–44. CEUR-WS.org.
Cannataro, M., Cuzzocrea, A., and Pugliese, A. (2002b).
XAHM: an adaptive hypermedia model based on
XML. In SEKE 2002, Ischia, Italy, July 15-19, 2002,
pages 627–634. ACM.
Ceci, M., Cuzzocrea, A., and Malerba, D. (2015). Ef-
fectively and efficiently supporting roll-up and drill-
down OLAP operations over continuous dimensions
via hierarchical clustering. J. Intell. Inf. Syst.,
44(3):309–333.
Cuzzocrea, A. (2005). Overcoming limitations of approx-
imate query answering in OLAP. In IDEAS 2005,
25-27 July 2005, Montreal, Canada, pages 200–209.
IEEE Computer Society.
Cuzzocrea, A. and Chakravarthy, S. (2010). Event-based
lossy compression for effective and efficient OLAP
over data streams. Data Knowl. Eng., 69(7):678–708.
Cuzzocrea, A., Darmont, J., and Mahboubi, H. (2009a).
Fragmenting very large XML data warehouses via k-
means clustering algorithm. Int. J. Bus. Intell. Data
Min., 4(3/4):301–328.
Cuzzocrea, A., Furfaro, F., Greco, S., Masciari, E., Mazzeo,
G. M., and Sacc
`
a, D. (2005). A distributed system
for answering range queries on sensor network data.
In PerCom 2005 Workshops, 8-12 March 2005, Kauai
Island, HI, USA, pages 369–373. IEEE Computer So-
ciety.
Cuzzocrea, A., Furfaro, F., Masciari, E., Sacc
`
a, D., and Sir-
angelo, C. (2004). Approximate query answering on
sensor network data streams. GeoSensor Networks,
49.
Cuzzocrea, A., Furfaro, F., and Sacc
`
a, D. (2003). Hand-
olap: A system for delivering OLAP services on hand-
held devices. In ISADS 2003, 9-11 April 2003, Pisa,
Italy, pages 80–87. IEEE Computer Society.
Cuzzocrea, A., Furfaro, F., and Sacc
`
a, D. (2009b). En-
abling OLAP in mobile environments via intelligent
data cube compression techniques. J. Intell. Inf. Syst.,
33(2):95–143.
Dodds, W. (2019). Disease now and potential future pan-
demics. The World’s Worst Problems, page 31–44.
Godio, A., Pace, F., and Vergnano, A. (2020). Seir model-
ing of the italian epidemic of sars-cov-2 using compu-
tational swarm intelligence. IJERPH, 17.
Harckbart, G. (2020). Heterogeneous siqr models with mass
testing and targeted quarantine and the spread of in-
fectious disease.
Hasaninasab, M. and Khansari, M. (2022). Efficient
COVID-19 testing via contextual model based com-
pressive sensing. Pattern Recognit., 122:108253.
Keeling, M. J. and Danon, L. (2009). Mathematical mod-
elling of infectious diseases. British Medical Bulletin,
92(1):33–42.
Kurtin, D. L., A.J., D., and Stagg, S. M. (2020). VTES:
a stochastic python-based tool to simulate viral trans-
mission [version 1]. F1000Research.
Li, S. and Yan, Y. (2022). Data-driven shock impact of
COVID-19 on the market financial system. Inf. Pro-
cess. Manag., 59(1):102768.
Mishra, B. K. and Singh, A. K. (2011). Two quarantine
models on the attack of malicious objects in computer
network. Hindawi Publishing Corporation.
Morens, D. M., Daszak, P., Markel, H., and Taubenberger,
J. K. (2020). Pandemic covid-19 joins history’s pan-
demic legion. mBio, 3(11).
Morris, K. J., Egan, S. D., Linsangan, J. L., Leung, C. K.,
Cuzzocrea, A., and Hoi, C. S. H. (2018). Token-based
adaptive time-series prediction by ensembling linear
and non-linear estimators: A machine learning ap-
proach for predictive analytics on big stock data. In
ICMLA 2018, Orlando, FL, USA, December 17-20,
2018, pages 1486–1491. IEEE.
Ronaghi, F., Salimibeni, M., Naderkhani, F., and Mo-
hammadi, A. (2022). COVID19-HPSMP: COVID-19
adopted hybrid and parallel deep information fusion
framework for stock price movement prediction. Ex-
pert Syst. Appl., 187:115879.
Yadav, A. and Vishwakarma, D. K. (2022). A language-
independent network to analyze the impact of
COVID-19 on the world via sentiment analysis. ACM
Trans. Internet Techn., 22(1):28:1–28:30.
Virus Spread Modeling and Simulation: A Behavioral Parameters Approach and Its Application to Covid-19
193