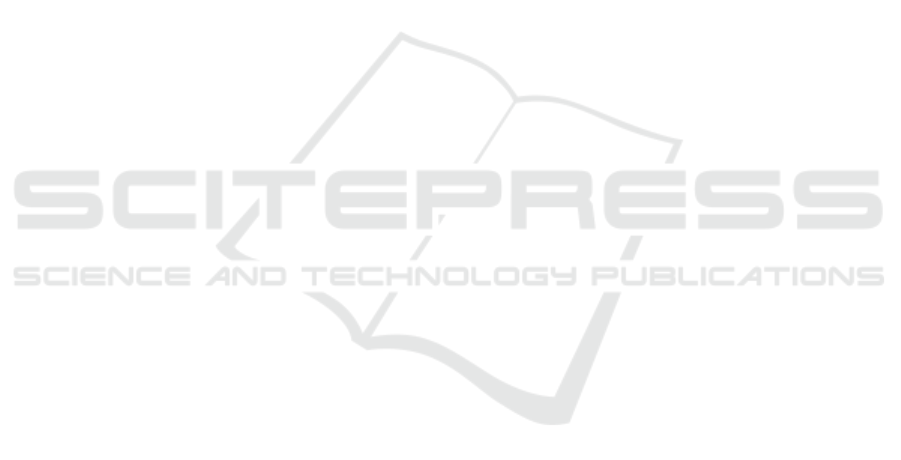
McKeown, G., Valstar, M. F., Cowie, R., and Pantic, M.
(2010). The semaine corpus of emotionally colou-
red character interactions. In Multimedia and Expo
(ICME), 2010 IEEE International Conference on, pa-
ges 1079–1084. IEEE.
Pantic, M., Valstar, M., Rademaker, R., and Maat, L.
(2005). Web-based database for facial expression ana-
lysis. In Multimedia and Expo, 2005. ICME 2005.
IEEE International Conference on, pages 5–pp. IEEE.
Qu, F., Wang, S.-J., Yan, W.-J., and Fu, X. (2016).
Cas(me)2: A database of spontaneous macro-
expressions and micro-expressions. In International
Conference on Human-Computer Interaction, pages
48–59. Springer.
Ringeval, F., Sonderegger, A., Sauer, J., and Lalanne, D.
(2013). Introducing the RECOLA Multimodal Corpus
of Remote Collaborative and Affective Interactions. In
Proceedings of EmoSPACE 2013, held in conjunction
with FG 2013, Shanghai, China. IEEE.
Russell, J. A. and Pratt, G. (1980). A description of the
affective quality attributed to environments. Journal
of personality and social psychology, 38(2):311.
Savran, A., Aly
¨
uz, N., Dibeklio
˘
glu, H., C¸ eliktutan, O.,
G
¨
okberk, B., Sankur, B., and Akarun, L. (2008). Bos-
phorus database for 3d face analysis. In European
Workshop on Biometrics and Identity Management,
pages 47–56. Springer.
Savran, A., Ciftci, K., Chanel, G., Mota, J., Hong Viet, L.,
Sankur, B., Akarun, L., Caplier, A., and Rombaut, M.
(2006). Emotion detection in the loop from brain sig-
nals and facial images.
Schmidt, K. L., Ambadar, Z., Cohn, J. F., and Reed, L. I.
(2006). Movement differences between deliberate and
spontaneous facial expressions: Zygomaticus major
action in smiling. Journal of Nonverbal Behavior,
30(1):37–52.
Schmidt, K. L. and Cohn, J. F. (2001). Dynamics of facial
expression: Normative characteristics and individual
differences. In ICME. Citeseer.
Sneddon, I., McRorie, M., McKeown, G., and Hanratty, J.
(2012). The belfast induced natural emotion database.
Affective Computing, IEEE Transactions on, 3(1):32–
41.
Soleymani, M., Lichtenauer, J., Pun, T., and Pantic, M.
(2012). A multimodal database for affect recogni-
tion and implicit tagging. Affective Computing, IEEE
Transactions on, 3(1):42–55.
Stratou, G., Ghosh, A., Debevec, P., and Morency, L.-P.
(2011). Effect of illumination on automatic expres-
sion recognition: a novel 3d relightable facial data-
base. In Automatic Face & Gesture Recognition and
Workshops (FG 2011), 2011 IEEE International Con-
ference on, pages 611–618. IEEE.
Tcherkassof, A., Dupr
´
e, D., Meillon, B., Mandran, N., Du-
bois, M., and Adam, J.-M. (2013). Dynemo: A vi-
deo database of natural facial expressions of emoti-
ons. The International Journal of Multimedia & Its
Applications, 5(5):61–80.
Toole, A. J., Harms, J., Snow, S. L., Hurst, D. R., Pappas,
M. R., Ayyad, J. H., and Abdi, H. (2005). A video
database of moving faces and people. Pattern Analy-
sis and Machine Intelligence, IEEE Transactions on,
27(5):812–816.
Valstar, M. and Pantic, M. (2010). Induced disgust, happi-
ness and surprise: an addition to the mmi facial ex-
pression database. In Proc. 3rd Intern. Workshop on
EMOTION (satellite of LREC): Corpora for Research
on Emotion and Affect, page 65.
Valstar, M., Schuller, B., Smith, K., Eyben, F., Jiang, B.,
Bilakhia, S., Schnieder, S., Cowie, R., and Pantic, M.
(2013). Avec 2013: the continuous audio/visual emo-
tion and depression recognition challenge. In Procee-
dings of the 3rd ACM international workshop on Au-
dio/visual emotion challenge, pages 3–10. ACM.
Valstar, M. F., Gunes, H., and Pantic, M. (2007). How to
distinguish posed from spontaneous smiles using ge-
ometric features. In Proceedings of the 9th internati-
onal conference on Multimodal interfaces, pages 38–
45. ACM.
Van Der Schalk, J., Hawk, S. T., Fischer, A. H., and Doosje,
B. (2011). Moving faces, looking places: validation of
the amsterdam dynamic facial expression set (adfes).
Emotion, 11(4):907.
Wang, S., Liu, Z., Lv, S., Lv, Y., Wu, G., Peng, P., Chen, F.,
and Wang, X. (2010). A natural visible and infrared
facial expression database for expression recognition
and emotion inference. Multimedia, IEEE Transacti-
ons on, 12(7):682–691.
Yin, L., Chen, X., Sun, Y., Worm, T., and Reale, M. (2008).
A high-resolution 3d dynamic facial expression data-
base. In Automatic Face & Gesture Recognition, 2008.
FG’08. 8th IEEE International Conference On, pages
1–6. IEEE.
Yin, L., Wei, X., Sun, Y., Wang, J., and Rosato, M. J.
(2006). A 3d facial expression database for facial be-
havior research. In Automatic face and gesture recog-
nition, 2006. FGR 2006. 7th international conference
on, pages 211–216. IEEE.
Zafeiriou, S., Papaioannou, A., Kotsia, I., Nicolaou, M. A.,
Zhao, G., Antonakos, E., Snape, P., Trigeorgis, G.,
and Zafeiriou, S. (2016). Facial affect in-the-wild: A
survey and a new database. In International Confe-
rence on Computer Vision.
Zara, A., Maffiolo, V., Martin, J. C., and Devillers, L.
(2007). Collection and annotation of a corpus of
human-human multimodal interactions: Emotion and
others anthropomorphic characteristics. In Affective
computing and intelligent interaction, pages 464–475.
Springer.
Zeng, Z., Pantic, M., Roisman, G., Huang, T. S., et al.
(2009). A survey of affect recognition methods: Au-
dio, visual, and spontaneous expressions. Pattern
Analysis and Machine Intelligence, IEEE Transacti-
ons on, 31(1):39–58.
Zhalehpour, S., Onder, O., Akhtar, Z., and Erdem, C. E.
(2016). Baum-1: A spontaneous audio-visual face da-
tabase of affective and mental states. IEEE Transacti-
ons on Affective Computing.
Zhang, L., Walter, S., Ma, X., Werner, P., Al-Hamadi, A.,
Traue, H. C., and Gruss, S. (2016). biovid emo db: A
A Survey on Databases for Facial Expression Analysis
83