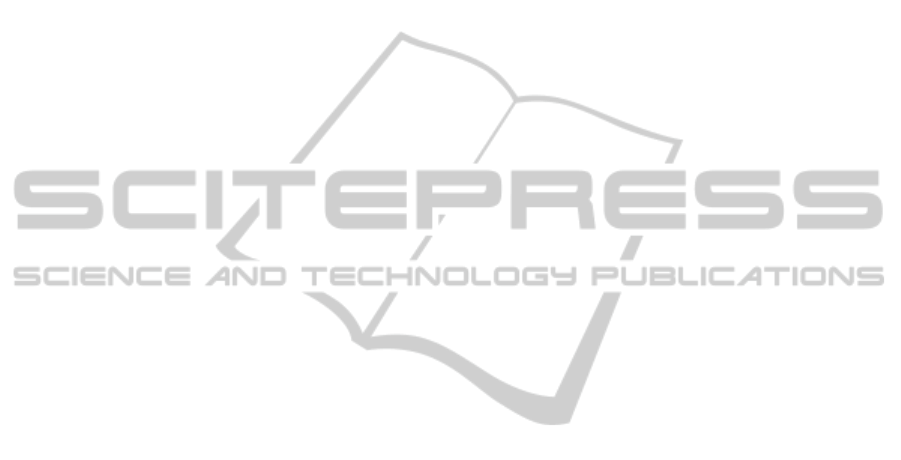
with calculating ROSI is the lack of data concerning
information security (Levy, 2005). Security breaches
and incidents are still seen as a reason for
embarrassment and few companies are willing or
able to provide actual costs associated with security
breaches (Sonnenreich, 2006). However, whilst the
data itself can be improved in terms of accuracy, it is
in fact the reproducible nature of the calculation that
is important, this reproducible nature arises from a
consistent methodology of calculating and reporting
the cost (Sonnenreich, 2006).
There are multiple factors and numerous
alternatives to consider when such a widely used
methodology as multi-criteria decision-making
(MCDM) is used. MCDM is a collection of
methodologies used to compare, select or rank
multiple alternatives that typically involve
incommensurate attributes (Levy, 2005). MCDM
can fall in to two main categories; 1) decision
making under certainty and 2) decision making
under uncertainty. However, in practice, it is
extremely unlikely to have complete information
about the future (Shah et al., 2007). The values used
in ROSI calculations are subject to uncertainty,
primarily down to the fact that you cannot predict,
with a high level of certainty, the losses caused
when an event may or may not happen
(Sonnenreich, 2006). Consequently, decision-
making under uncertainty is the logical method to
use in order to allow for realistic solutions.
Though there are previous applications of
decision analysis to ROSI problems, the inclusion of
multi-attribute utility theory (MAUT) hasn’t been
applied to its fullest extent. The cases of MAUT
being used are restricted to a small number of
attributes, such as cost and availability (Ioannidis et
al., 2010), (Beautement et al., 2008), or cost,
investment and availability (Beres, Pym and Shiu,
2010) for example. The limited use of MAUT
doesn’t show the full extent to which decision
analysis can be used to support ROSI calculations.
2 RELATED WORK
2.1 Return on Security Investment
Performing an ROSI calculation is a method of
evaluating a security investment prior to making a
decision; it compares the cost of implementing and
procuring a solution to the losses avoided. It is the
misconception of what ROSI demonstrates, in terms
of not necessarily representing a profit, which has
led to its misuse.
Relatively recently, in 2002, Gordon and Loeb
adopted a static optimisation model where the
optimal ratio of investment in Information Security
can be calculated under different assumptions of
expected loss. This model relies on restrictive
assumptions to calculate the optimal ratio and has
sparked much debate regarding whether the
relationship between Information Security
Investment and vulnerability is always a monotonic
function. In 2006, Hausken proposed that
vulnerability be represented as a function, showing
the optimal ratio cannot be supported.
An important issue is that the inputs for ROSI
may be highly subjective, and consequently,
companies that use the same method for calculation
can arrive at extremely varied results due to different
choices made about the inclusion or exclusion of
costs (Sonnenreich, 2006). In addition, estimating
losses from future events brings uncertainty in to the
values used. Even when actuarial tables or insurance
data are used, these values may not be accurate due
to the “ostrich effect” (Sonnenreich, 2006)
experienced when an incident occurs. Another way
to collet data is to base values on competitors
experiences. This could be to investigate losses
incurred by competitors during incidents, or an
uptake in sales within an organisation once a
competitor has experienced an attack. These values
of losses or gains can be added on to the ROSI
analysis (Korostoff, 2003) or values based on
previous experience can also be used. Finally, the
variable most complex to define and evaluate is the
mitigated risk. One method to consider is the use of
past data, determining the expected losses due to
security breaches prior, and subsequent to,
implementing a solution (Arora, Frank and Telang,
2008). The avoided risk or expected benefit is then
the difference between the baseline loss and residual
risk. When using this approach, it must be
recognised that rare events may preclude the use of
past data, when such events occurred sufficiently
long ago that the same conditions no longer apply.
ROSI calculations, as explained above are based
heavily on estimations or perceptions of values –
this makes ROSI more of an approximation and less
accurate. It is the ability to manipulate these
approximations to justify decisions (ENISA, 2012)
that call for ROSI to be improved, in terms of
reproducibility, repeatability and predictability. The
biased perceptions of a decision maker should not
cause differing outputs of the calculations. ROSI
equations should be objective and numerical but due
to insufficient definition or subjective variables, the
calculations become imprecise and subjective
DeterminingtheValueofInformationSecurityInvestments-ADecisionSupportSystem
427