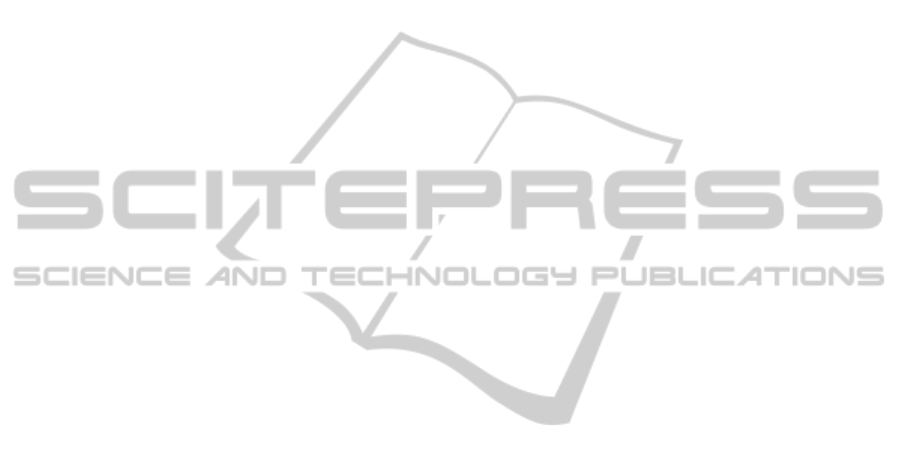
is an internationally recognized expert in the area of
psychometrics.
By now various versions of SCEQs have been
serving institutions of higher learning around the
globe for a long time (Abrami and Cohen, 1990).
Course evaluations are incorporated in the process
by which Universities seek to improve teaching and
learning, and to consider faculty for merit raises, pro-
motion and tenure. The UMBC questionnaireconsists
of seven sets of items. One set contains general ques-
tions that should be applicable to almost all courses.
The remaining sets are designed for lectures, discus-
sion, mathematics and science laboratories, seminars,
field experience, and self-paced courses. Six ques-
tions permit separate evaluation of as many as four in-
structors. The instructor has the option of administer-
ing whichever sets of questions are applicable. This
study focuses on general question 9 (G9) “How would
you grade the overall teaching effectiveness.”
UMBC has been using information collected
through SCEQs for a variety of purposes for about
forty years. UMBC Student Course Evaluation Ques-
tionnaires contain student ratings ranging from 1
(worst) to 5 (best) per each question asked. The
SCEQ administration protocol is described in writ-
ing, and the Instructor is charged with the responsi-
bility of conducting the survey. The quality of SCEQ
scores depends on students’ competence to evaluate
an instructor and may vary, but a student’s evaluation
is independent, for example, of the student’s GPA. In
other terms, ratings assigned by “F” students are as
important for instructor’s teaching evaluation as those
assigned by “A” students.
The ratings per question are averaged out, i.e., the
ratings per question are added up and the sum is di-
vided by the number of students who responded to the
question (see e.g. (Hardy et al., 1934) where mean
evaluations are discussed). This average is named
“Instructor Mean.”
Along with individual instructor statistics per
class/question, SCEQ provides additional statistical
indicators, among them “Org Mean” representing a
discipline. UMBC computed org means are actually
mean averages of the instructor’s means. The aver-
age scores for a class with one response are weighted
equally to a class with numerous responses when “av-
eraging the averages.” Instructor Means for classes
of different size contribute equally to the Org Mean.
Hence the input of large student groups (students in
large classes) to the computation of Org Mean is iden-
tical to that of small student groups (students in small
classes).
To make this point clear we consider a hypothet-
ical “Org” with just two instructors, A and B. While
A teaches a small class with one student, B teaches
a large class with one hundred students. The single
student in the small class rates the “overall teaching
effectiveness” (question G9 of the questionnaire, and
the main focus of this paper) of the instructor by 5,
while each of the one hundred students in the large
class responds to the same question with 3. Hence the
Instructor A mean is 5, the Instructor B mean is 3, and
the Department Mean is (5 + 100 × 3)/101 = 3.02.
However the Org Mean reported by on the UMBC
SCEQ forms for this hypothetical “Org” would be
(3+ 5)/2 = 4.
There are two major deficiencies of the org mean
computation currently in place at UMBC:
1. The current method reports instructor B rating 3
significantly below the Org Mean 4, while in fact
the Org Mean is only 3.02. The reporting distorts
reality.
2. As the example above shows, the opinion of a
single student in the small class carries the same
weight as that of the 100 students in the large
class. In other terms, the voice of one student in
the large class worth just 0.01 of the voice of the
one student in the small class.
There is no reason for discrimination based solely on
the class size.
The results reported in this paper provide means
computed in accordance with standard mathematical
definition of the arithmetic mean (see e.g. (Hardy
et al., 1934), (Hodges and Lehmann, 1964)). The
same way means are computed by the University of
Maryland College Park (UMCP). Each reference to
means computed by UMBC is specifically indicated
in the text below.
3 LEVEL MEANS
The UMBC website provides information about 82
different disciplines (“orgs”). We remove 11 “non
academic” orgs (with classes such as “Aerobics,”
“Walking/Jogging,” etc.), and focus on students rat-
ings for undergraduate classes (those are 100, 200,
300, and 100 level courses). We compute and graph
means for undergraduate courses covering 71 aca-
demic disciplines over 16 semesters (Spring 2005-
Fall 2012, total of 64 semesters, see Figure 1). The
graph shows that the means slowly climb up as levels
advance from 100 to 400.
We now partition 71 academic disciplines into 6
clusters:
1. Arts,
2. Engineering & Technology,
CSEDU2014-6thInternationalConferenceonComputerSupportedEducation
222