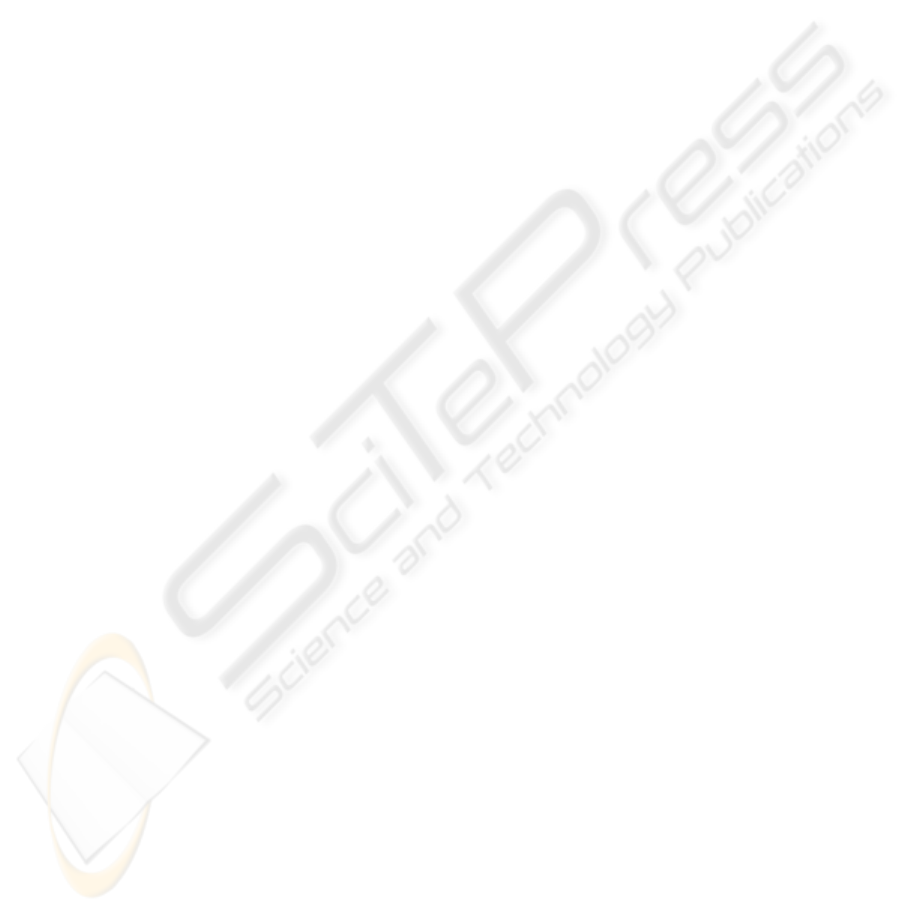
ACKNOWLEDGEMENTS
The authors thank the Swiss Agency of Promotion
and Innovation for the financial support of this work,
under the grant 6066.1 (e-Fulfillment).
REFERENCES
Bendixen, M., Bukasa, K.A., and Abratt, R., 2004. Brand
equity in the business-to-business market. Industrial
Marketing Management, 33, 371-380.
Canetta, L., Cheikhrouhou, N., Glardon, R., and Iliev, N.,
2004. Supporting electronic sales channels deployment
through the analysis of customer preferences. In IEEE
Conference on Systems, Man and Cybernetics, Den
Haag, 4123-4128.
Choi, B., Lee, C., Lee, H., and Subramani, M., 2004.
Effects of web retail service quality and product
categories on consumer behaviour. In 37th HICSS
(Hawaii International Conference on System
Sciences), 177-186.
Clemons, E.K., and Aron, R., 2002. Online distribution: a
taxonomy of channel structures determinants of
outcome and determinants of strategy. In 35th HICSS
2330-2339.
Fillis, I., Johansson, U., and Wagner, B., 2003. A
conceptualization of the opportunities and barriers to
e-business development in the smaller firm. Journal of
Small Business and Enterprise Development, 10(3),
336-344.
Gurunlian, J., and Zhongzhou, L., 2001. E-commerce and
Development Report. United Nations, New York and
Geneva.
Hunter, L.M., Kasouf, C.J., Celuch, K.J., and Curry, K.A.,
2004. A classification of business-to-business buying
decisions: risk importance and probability as a
framework for e-business benefits. Industrial
Marketing Management, 33(2), 145-154.
Iliev, N., Alard, R., Canetta, L., Cheikhrouhou, N., 2004.
Evaluating Electronic Enabled Transaction Costs´
Efficiency. In International Conference on Global
Production Management, Indonesia, 21-31.
Kuppens, R., 2006. Keeping up with innovation. ELO
Innovation Challenges in Logistics, Helsinki.
http://akseli.tekes.fi/opencms/opencms/OhjelmaPortaa
li/ohjelmat/ELO/fi/Dokumenttiarkisto/Viestinta_ja_akt
ivointi/Seminaarit/ELO_loppuseminaari/20060315_Ci
sco_SCM_Helsinki_rkuppens.pdf (view 25.10.2006)
Levin, A.M., Levin, I.P., and Heath C.E., 2003. Product
category dependent consumer preferences for online
and offline shopping features and their influence on
multi-channel retail alliances. Journal of Electronic
Commerce Research, 4(3), 85-93.
Liang, T.P., and Huang, J.S., 1998. An empirical study on
consumer acceptance of products in electronic
markets: a transaction cost model. Decision Support
Systems, 24, 29-43.
Lim, N., 2003. Consumers’ perceived risk: sources versus
consequences. Electronic Commerce Research and
Applications, 2, 216-228.
Liu, J.Y., Tarn, J.M., and Wen, H.J., 2004. Adopting e-
commerce web site operation modes - the current stage
of large US firms. Information Management and
Computer Security, 12(1), 73-90.
Liu, X., and Wei, K.K., 2003. An empirical study of
product differences in consumers’ e-commerce
adoption behaviour.
Electronic Commerce Research
and Applications, 2, 229-239.
Lowengart, O., and Tractinsky, N., 2001. Differential
effects of product category on shoppers’ selection of
web-based stores: a probabilistic modeling approach.
Journal of Electronic Commerce Research, 2(4), 142-
156.
Mudambi, S., 2002. Branding importance in business-to-
business market – three buyer clusters. Industrial
Marketing Management, 31, 525-533.
Peterson, R.A., Balasubramanian, S., Bronnenberg, B.J.,
1997. Exploring the implications of the Internet for
consumer marketing. Journal of the Academy of
Marketing Science, 25(4), 329-346.
Phau, I., Poon, S.M., 2000. Factors influencing the types
of products and services purchased over the Internet.
Internet Research: Electronic Networking
Applications and Policy, 10(2), 102-113.
Scupola, A., 2002. Adoption issues of business-to-
business Internet commerce in European SMEs. In
35th HICSS, 2119-2128.
Selhofer, H., 2004. The European e-Business Report.
European Commission Enterprise Directorate General,
Bonn.
Stansfield, M., and Grant, K., 2003. An investigation into
issues influencing the use of the internet and electronic
commerce among small-medium size enterprises.
Journal of Electronic Commerce Research, 4(1), 15-
26.
Subramaniam, C., and Shaw, M.J., 2002. A study on the
value and impact of B2B e-commerce: the case of
web-based procurement. International Journal of
Electronic Commerce, 6(4), 41-72.
Teng, J.T.C., Grover, V., and Güttler, W., 2002.
Information technology innovations: general diffusion
patterns and its relationships to innovation
characteristics. IEEE Transactions on Engineering
Management, 49(1), 13-27.
Van den Poel, D., and Leunis, J., 1999. Consumer
acceptance of the Internet as a channel of distribution.
Journal of Business Research, 45, 249-256.
Vijayasarathy, L.R., 2002. Product characteristics and
internet shopping intention. Internet Research:
Electronic Networking Applications and Policy, 12(5),
411-426.
Zhou, L., Chiang, W.K., and Zhang D., 2004. Discovering
rules for predicting customers’ attitude toward internet
retailers. Journal of Electronic Commerce Research,
5(4), 228-238.
WEBIST 2007 - International Conference on Web Information Systems and Technologies
106