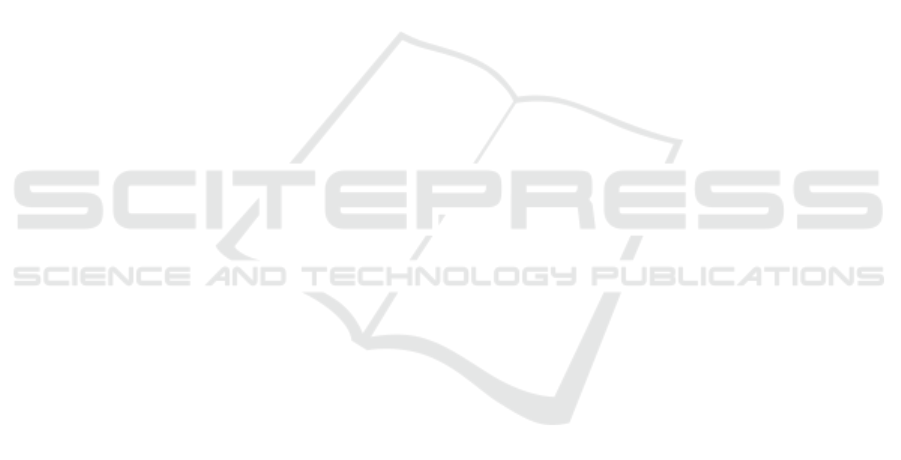
A., Rueckert, D., and Glocker, B. (2017). Unsuper-
vised domain adaptation in brain lesion segmentation
with adversarial networks. Lecture Notes in Computer
Science (including subseries Lecture Notes in Artifi-
cial Intelligence and Lecture Notes in Bioinformatics),
10265 LNCS:597–609.
Lee, D., Hofmann, M., Steinke, F., Altun, Y., Cahill,
N. D., and Sch
¨
olkopf, B. (2009). Learning sim-
ilarity measure for multi-modal 3D image registra-
tion. 2009 IEEE Computer Society Conference on
Computer Vision and Pattern Recognition Workshops,
CVPR Workshops 2009, pages 186–193.
Marcus, D. S., Wang, T. H., Parker, J., Csernansky, J. G.,
Morris, J. C., and Buckner, R. L. (2007). Open access
series of imaging studies (OASIS): Cross-sectional
MRI data in young, middle aged, nondemented, and
demented older adults. Journal of Cognitive Neuro-
science, 19:1498–1507.
Mattes, D., Haynor, D., Vesselle, H., Lewellen, T., and Eu-
bank, W. (2001). Non-rigid multimodality image reg-
istration. In Medical Imaging 2001: Image Process-
ing. SPIE Publications, pages 1609–1620.
Miao, S., Wang, Z. J., Zheng, Y., and Liao, R. (2016). Real-
time 2D/3D registration via CNN regression. Pro-
ceedings - International Symposium on Biomedical
Imaging, 2016-June:1430–1434.
Murphy, K., Ginneken, B. V., Reinhardt, J. M., Member,
S., Kabus, S., Ding, K., Deng, X., Cao, K., Du, K.,
Christensen, G. E., Garcia, V., Vercauteren, T., Ay-
ache, N., Commowick, O., Malandain, G., Glocker,
B., Paragios, N., Navab, N., Gorbunova, V., Sporring,
J., Bruijne, M. D., Han, X., Heinrich, M. P., Schn-
abel, J. A., Jenkinson, M., Lorenz, C., Modat, M.,
Mcclelland, J. R., Ourselin, S., Muenzing, S. E. A.,
Viergever, M. A., Nigris, D. D., Collins, D. L., Arbel,
T., Peroni, M., Li, R., Sharp, G. C., Schmidt-richberg,
A., Ehrhardt, J., Werner, R., Smeets, D., Loeckx, D.,
Song, G., Tustison, N., Avants, B., Gee, J. C., Star-
ing, M., Klein, S., Stoel, B. C., Urschler, M., Werl-
berger, M., Vandemeulebroucke, J., Rit, S., Sarrut, D.,
and Pluim, J. P. W. (2011). Evaluation of Registration
Methods on Thoracic CT : The EMPIRE10 Challenge.
IEEE transactions on medical imaging, 30(11):1901–
1920.
Scherer, D., Andreas, M., and Behnke, S. (2010). Evalua-
tion of Pooling Operations in Convolutional Architec-
tures for Object Recognition. In International Confer-
ence on Artifical Neural Networks, number September
2010.
Simonovsky, M., Guti
´
errez-Becker, B., Mateus, D., Navab,
N., and Komodakis, N. (2016). A Deep Metric for
Multimodal Registration. In Medical Image Com-
puting and Computer-assisted Intervention – MICCAI
2016, pages 1–10.
Smriti, R., Steredney, D., Schmalbrock, P., and Clymer,
B. D. (2005). Image Registration Using Rigid Reg-
istration and Maximisation of Mutual Information. In
13th Annual Medicine Meets Virtual Reality Confer-
ence, pages 26–29.
Song, G. and Tustison, N. J. (2010). Lung CT image reg-
istration using diffeomorphic transformation models.
Medical Image Analysis for the Clinic, pages 23–32.
Srivastava, N., Hinton, G., Krizhevsky, A., Sutskever, I.,
and Salakhutdinov, R. (2014). Dropout: A Simple
Way to Prevent Neural Networks from Overfitting.
Journal of Machine Learning Research, 15:1929–
1958.
Wu, G., Kim, M., Wang, Q., Brent, M., and Shen, D.
(2016). Scalable High Performance Image Registra-
tion Framework by Unsupervised Deep Feature Rep-
resentations Learning. IEEE Transactions on Biomed-
ical Engineering, 63(7):1505–1516.
Zhu, J.-Y., Park, T., Isola, P., and Efros, A. A. (2017).
Unpaired Image-to-Image Translation using Cycle-
Consistent Adversarial Networks.
Learning Rigid Image Registration - Utilizing Convolutional Neural Networks for Medical Image Registration
99