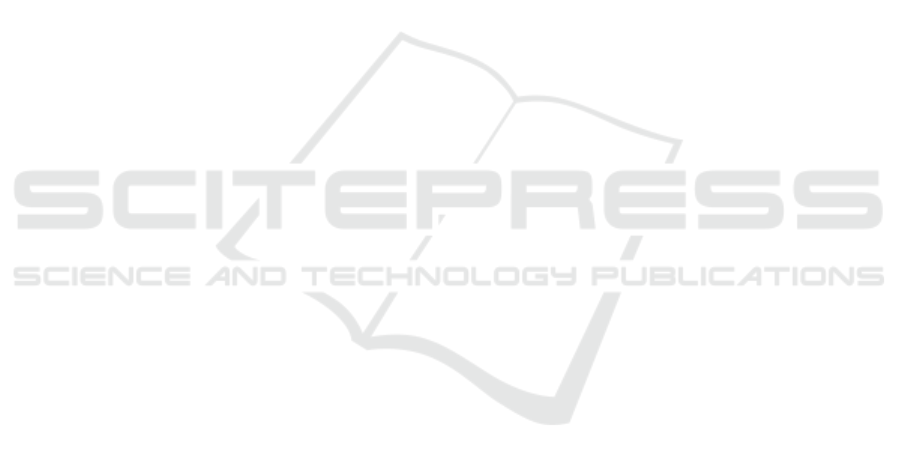
nih.gov/articlerender.fcgi?artid=2730469&tool=pmce
ntrez&rendertype=abstract.
Ito, T. et al., 2008. Two functional subsets of FOXP3+
regulatory T cells in human thymus and periphery.
Immunity, 28(6), pp.870–80. Available at:
http://www.pubmedcentral.nih.gov/articlerender.fcgi?a
rtid=2709453&tool=pmcentrez&rendertype=abstract.
Kitagawa, Y., Ohkura, N. & Sakaguchi, S., 2015.
Epigenetic control of thymic Treg-cell development.
European Journal of Immunology, 45(1), pp.11–16.
Klatzmann, D. & Abbas, A.K., 2015. The promise of low-
dose interleukin-2 therapy for autoimmune and
inflammatory diseases. Nature reviews. Immunology,
15(5), pp.283–94. Available at: http://
www.nature.com.gate1.inist.fr/nri/journal/v15/n5/full/
nri3823.html.
Lin, W. et al., 2007. Regulatory T cell development in the
absence of functional Foxp3. Nature immunology,
8(4), pp.359–368.
Liu, Z. et al., 2009. Treg suppress CTL responses upon
immunization with HSP gp96. European Journal of
Immunology, 39(11), pp.3110–3120.
Miyara, M., Ito, Y. & Sakaguchi, S., 2014. TREG-cell
therapies for autoimmune rheumatic diseases. Nature
reviews. Rheumatology, 10(9), pp.543–51. Available
at: http://www.ncbi.nlm.nih.gov/pubmed/24980140.
Mota, C. et al., 2014. Delta-like 1-Mediated Notch
Signaling Enhances the In Vitro Conversion of Human
Memory CD4 T Cells into FOXP3-Expressing
Regulatory T Cells. The Journal of Immunology,
193(12), pp.5854–62. Available at: http://
www.jimmunol.org/content/193/12/5854.full.
Nagalakshmi, U., Waern, K. & Snyder, M., 2010. RNA-
seq: A method for comprehensive transcriptome
analysis. Current Protocols in Molecular Biology,
(SUPPL. 89).
Nunes-Cabaço, H. et al., 2011. Differentiation of human
thymic regulatory T cells at the double positive stage.
European journal of immunology, 41, pp.3604–3614.
Available at: http://onlinelibrary.wiley.com/doi/
10.1002/eji.201141614/full.
Ohkura, N. et al., 2012. T Cell Receptor Stimulation-
Induced Epigenetic Changes and Foxp3 Expression
Are Independent and Complementary Events Required
for Treg Cell Development. Immunity, 37(5), pp.785–
799.
Pandiyan, P. et al., 2007. CD4+CD25+Foxp3+ regulatory
T cells induce cytokine deprivation-mediated
apoptosis of effector CD4+ T cells. Nature
immunology, 8(12), pp.1353–1362.
Povoleri, G.A.M. et al., 2013. Thymic versus induced
regulatory T cells-who regulates the regulators?
Frontiers in Immunology, 4(JUN), pp.1–22.
Ralainirina, N. et al., 2007. Control of NK cell functions
by CD4+CD25+ regulatory T cells. J Leukoc Biol,
81(1), pp.144–153. Available at: http://
www.jleukbio.org/cgi/content/abstract/81/1/144%5Cn
http://www.jleukbio.org/cgi/reprint/81/1/144.pdf.
Relland, L.M. et al., 2012. The TCR repertoires of
regulatory and conventional T cells specific for the
same foreign antigen are distinct. Journal of
immunology, 189, pp.3566–74. Available at:
http://www.pubmedcentral.nih.gov/articlerender.fcgi?a
rtid=3538134&tool=pmcentrez&rendertype=abstract.
Roadmap Epigenomics Consortium, et al., 2015.
Integrative analysis of 111 reference human
epigenomes. Nature, 518(7539), pp.317–330.
Available at:
http://www.ncbi.nlm.nih.gov/pubmed/25693563.
Saeys, Y., Gassen, S. Van & Lambrecht, B.N., 2016.
Computational flow cytometry: helping to make sense
of high-dimensional immunology data. Nature
Reviews Immunology. Available at: http://
www.nature.com/doifinder/10.1038/nri.2016.56.
Shumway, M., Cochrane, G. & Sugawara, H., 2009.
Archiving next generation sequencing data. Nucleic
Acids Research, 38(SUPPL.1), pp.2009–2010.
Silva, S.L. et al., 2016. Human naive regulatory T-cells
feature high steady-state turnover and are maintained
by IL-7. Oncotarget.
Spence, A. et al., 2015. Targeting Treg signaling for the
treatment of autoimmune diseases. Current Opinion in
Immunology, 37, pp.11–20.
Spits, H., 2002. Development of αβ t cells in the human
thymus. Nature Reviews Immunology, 2(10), pp.760–
772. Available at: http://www.nature.com/doifinder/
10.1038/nri913.
Starr, T.K., Jameson, S.C. & Hogquist, K.A., 2003.
Positive and negative selection of T cells. Annu Rev
Immunol, 21, pp.139–176. Available at: http://
www.ncbi.nlm.nih.gov/entrez/query.fcgi?cmd=Retriev
e&db=PubMed&dopt=Citation&list_uids=12414722.
Tang, Q. & Bluestone, J.A., 2013. Regulatory T-cell
therapy in transplantation: Moving to the clinic. Cold
Spring Harbor Perspectives in Medicine, 3(11).
Tarakhovsky, A., 2010. Tools and landscapes of
epigenetics. Nature immunology, 11(7), pp.565–568.
Available at: http://dx.doi.org/10.1038/ni0710-565.
Wang, R.-F., 2006. Regulatory T cells and toll-like
receptors in cancer therapy. Cancer research, 66(10),
pp.4987–90. Available at: http://www.ncbi.nlm.
nih.gov/ pubmed/16707417.
Wang, Z., Gerstein, M. & Snyder, M., 2009. RNA-Seq: a
revolutionary tool for transcriptomics. Nature reviews.
Genetics, 10(1), pp.57–63. Available at: http://
www.ncbi.nlm.nih.gov/pubmed/19015660.
Wong, J. et al., 2007. Adaptation of TCR repertoires to
self-peptides in regulatory and nonregulatory CD4+ T
cells. Journal of immunology, 178(11), pp.7032–7041.
Available at: papers2://publication/uuid/C9A56955-
F35C-4DA9-BAE2-4C050A1104EC.
Yu, A. et al., 2009. A Low Interleukin-2 Receptor
Signaling Threshold Supports the Development and
Homeostasis of T Regulatory Cells. Immunity, 30(2),
pp.204–217. Available at: http://dx.doi.org/10.1016/
j.immuni.2008.11.014.
Regulatory T cell Development in the Human Thymus - A Comprehensive Approach Combining Genome-wide Analysis and Single-cell
Protein Expression by Computational Flow Cytometry
9