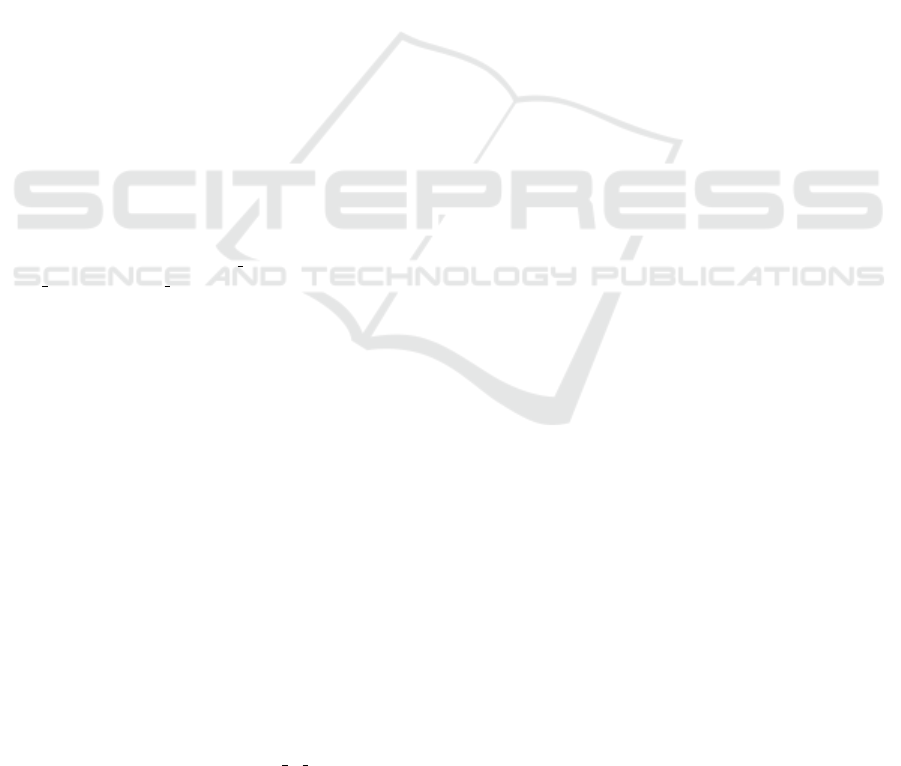
Glasgow. The CHART-ADAPT project we would like
to acknowledge the staff and patients of the Neuroin-
tensive care unit, Neurosciences Institute, Glasgow.
REFERENCES
A.Ferguson (2012). The evolution of confidentiality in the
united kingdom and the west. AMA Journal of Ethics,
14(9):738–742.
Aitken, M., Cunningham-Burley, S., and Pagliari, C.
(2016a). Moving from trust to trustworthiness: Ex-
periences of public engagement in the scottish health
informatics programme. Science and Public Policy,
43(5):713–723.
Aitken, M., de St Jorre, J., Pagliari, C., Jepson, R., and
Cunningham-Burley, S. (2016b). Public responses to
the sharing and linkage of health data for research pur-
poses: a systematic review and thematic synthesis of
qualitative studies. BMC Medical Ethics, 17(73).
Apache a (2016). Apache Spark. https://spark.apache.org/.
Accessed: Nov 2016.
Apache b (2016). Apache Hadoop.
https://hadoop.apache.org/. Accessed: Nov 2016.
BMA (2016). Secondary Uses of Data, Public Workshop.
https://www.bma.org.uk/collective-voice/policy-
and-research/ethics/secondary-uses-of-data/public-
workshop. Accessed: Nov 2016.
Caldicott (2016). Information: To share or not to
share? The Information Governance Review.
https://www.gov.uk/government/uploads/system/
uploads /attachment data/file/192572/2900774
InfoGovernance accv2.pdf. Accessed: Nov 2016.
CHART-ADAPT (2016). CHART-ADAPT.
http://www.chartadapt.org. Accessed: Nov 2016.
DeepMind a (2016). DeepMind Acute Kidney In-
jury. Royal Free London. Google DeepMind:
Q&A. https://www.royalfree.nhs.uk/news-
media/news/google-deepmind-qa/. Accessed:
Nov 2016.
DeepMind b (2016). DeepMind Moorfields Eye
Hospital. Moorfields announces research partner-
ship. http://www.moorfields.nhs.uk/news/moorfields-
announces-research-partnership. Accessed: Nov
2016.
Fillmore, C., Braye, C., and Kawamoto, K. (2013). System-
atic review of clinical decision support interventions
with potential for inpatient cost reduction. BMC Med
Inform Decis Mak, 13(135).
Focus Groups, Stevenson, F., Lloyd, N., Harrington, L., and
Wallace, P. (2013). Use of electronic patient records
for research: views of patients and staff in general
practice. Fam Pract, 30(2):227–23.
GDPR (2016). GDPR: Regulation (EU)
2016/679. http://ec.europa.eu/justice/data-
protection/reform/files/regulation oj en.pdf. Ac-
cessed: Nov 2016.
IBM a (2016). IBM Big Data. Extracting busi-
ness value from the 4 V’s of big data.
http://www.ibmbigdatahub.com/infographic/extracting
-business-value-4-vs-big-data. Accessed: Nov 2016.
IBM b (2016). The 4 V’s of big data.
http://www.ibmbigdatahub.com/infographic/four-
vs-big-data. Accessed: Nov 2016.
IBM c (2016). IBM’s Watson supercomputer to speed up
cancer care. http://www.bbc.co.uk/news/technology-
32607688. Accessed: Nov 2016.
Jaspers, M., Smeulers, M., Vermeulen, H., and Peute, L.
(2011). Effects of clinical decision-support systems
on practitioner performance and patient outcomes: a
synthesis of high-quality systematic review findings.
J Am Med Inform Assoc, 18(3):327–34.
Kaye, J., Whitley, E., Lund, D., Morrison, M., Teare, H.,
and Melham, K. (2015). Dynamic consent: a patient
interface for twenty-first century research networks.
Eur J Hum Genet, 23(2):141–6.
Kinsella, J., Hawthorne, C., Shaw, M., Piper, I., Healthcare,
P., Aridhia, and L.Moss (2017). Public perception of
the collection and use of critical care patient data be-
yond treatment: a pilot study. In Proceedings of the
Society of Critical Care Medicine Congress (SCCM).
SCCM.
Kitchen, R. and McArdle, G. (2016). What makes big data,
big data? exploring the ontological characteristics of
26 datasets. Big Data & Society, Jan-June 2016(3):1–
10.
New Scientist (2016). Revealed: Google AI
has access to huge haul of NHS pa-
tient data. New Scientist 2016 Apr 29.
https://www.newscientist.com/article/\2086454-
revealed-google-\ai-has-access-to-\huge-haul-of-
nhs-patient-data/. Accessed: Nov 2016.
Presser, L., Hruskova, M., Rowbottom, H., and Kancir, J.
(2015). Care.data and access to uk health records:
patient privacy and public trust. Technology Science,
2015081103(Aug 11).
Riordan, F., Papoutsi, C., Reed, J., Marston, C., Bell, D.,
and Majeed, A. (2015). Patient and public attitudes
towards informed consent models and levels of aware-
ness of electronic health records in the uk. Int J Med
Inform, 84(4):237–347.
Scala (2016). Scala Programming Language.
http://www.scala-lang.org/. Accessed: Nov 2016.
van Staa, T.-P., Goldacre, B., Buchan, I., and Smeeth, L.
(2016). Big health data: the need to earn public trust.
BMJ, 354:i3636.
Williams, H., Spencer, K., Sanders, K., Lund, D., Whitley,
E., Kaye, J., and Dixon, W. (2015). Dynamic consent:
A possible solution to improve patient confidence and
trust in how electronic patient records are used in med-
ical research. JMIR Med Inform, 3(1).
HEALTHINF 2017 - 10th International Conference on Health Informatics
468