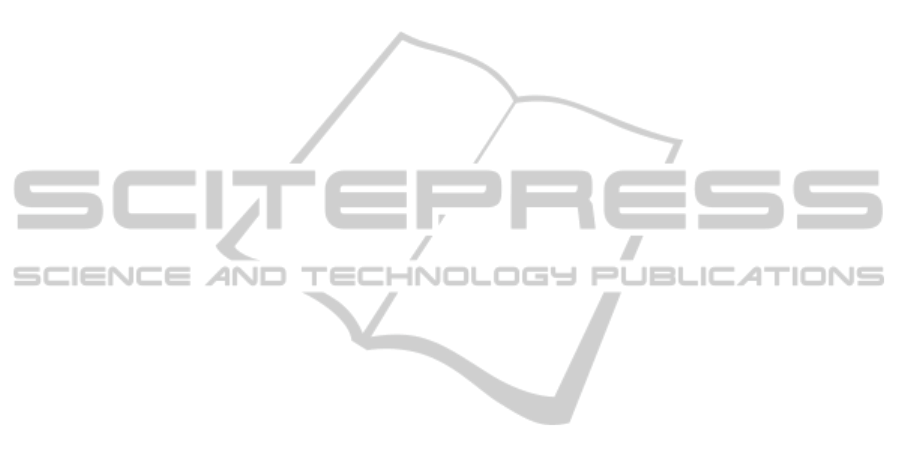
Cook, D., Schmitter-Edgecombe, M., Crandall, A.,
Sanders, C., and Thomas, B. (2009). Collecting and
disseminating smart home sensor data in the casas
project. In Proceedings of the CHI Workshop on De-
veloping Shared Home Behavior Datasets to Advance
HCI and Ubiquitous Computing Research.
Dadlani, P., Gritti, T., Shan, C., de Ruyter, B., and
Markopoulos, P. (2014). Sopresent: An awareness
system for connecting remote households. Ambient
Intelligence, page 67.
Davis, K., Hu, J., Feijs, L., and Owusu, E. B. (2015). So-
cial hue: A subtle awareness system for connecting
the elderly and their caregivers. In Pervasive Com-
puting and Communications Workshops (PERCOM
Workshops), 2015 IEEE International Conference on.
IEEE. In press.
De Greef, P. and Ijsselsteijn, W. A. (2001). Social pres-
ence in a home tele-application. CyberPsychology &
Behavior, 4(2):307–315.
Halmos, P. (2013). Solitude and privacy: A study of social
isolation, its causes and therapy. Routledge.
Kaye, J., Levitt, M. K., Nevins, J., Golden, J., and Schmidt,
V. (2005). Communicating intimacy one bit at a time.
In CHI’05 extended abstracts on Human factors in
computing systems, pages 1529–1532. ACM.
Landabaso, M. and Letter, L. D. (2013). Guide to social
innovation. page 71.
Markopoulos, P. and Mackay, W. (2009). Awareness sys-
tems: Advances in theory, methodology and design.
Springer Science & Business Media.
Metaxas, G., Metin, B., Schneider, J., Markopoulos, P., and
De Ruyter, B. (2007). Daily activities diarist: sup-
porting aging in place with semantically enriched nar-
ratives. In Human-Computer Interaction–INTERACT
2007, pages 390–403. Springer.
Mynatt, E. D., Rowan, J., Craighill, S., and Jacobs, A.
(2001). Digital family portraits: supporting peace of
mind for extended family members. In Proceedings of
the SIGCHI conference on Human factors in comput-
ing systems, pages 333–340. ACM.
Rashidi, P. and Mihailidis, A. (2013). A survey on ambient-
assisted living tools for older adults. IEEE journal of
biomedical and health informatics, 17(3):579–590.
Ravi, N., Dandekar, N., Mysore, P., and Littman, M. L.
(2005). Activity recognition from accelerometer data.
In AAAI, volume 5, pages 1541–1546.
Reisberg, B., Finkel, S., Overall, J., Schmidt-Gollas, N.,
Kanowski, S., Lehfeld, H., Hulla, F., Sclan, S. G.,
Wilms, H.-U., Heininger, K., et al. (2001). The
alzheimer’s disease activities of daily living interna-
tional scale (adl-is). International Psychogeriatrics,
13(02):163–181.
Rodriguez-Martin, D., Sama, A., Perez-Lopez, C., Catala,
A., Cabestany, J., and Rodriguez-Molinero, A. (2013).
Svm-based posture identification with a single waist-
located triaxial accelerometer. Expert Systems with
Applications, 40(18):7203–7211.
Romero, N., Markopoulos, P., Van Baren, J., De Ruyter, B.,
Ijsselsteijn, W., and Farshchian, B. (2007). Connect-
ing the family with awareness systems. Personal and
Ubiquitous Computing, 11(4):299–312.
Sheldon, J. H. et al. (1948). The social medicine of old age.
report of an enquiry in wolverhampton. The Social
Medicine of Old Age. Report of an Enquiry in Wolver-
hampton.
Stafford, M., McMunn, A., Zaninotto, P., and Nazroo, J.
(2011). Positive and negative exchanges in social re-
lationships as predictors of depression: evidence from
the english longitudinal study of aging. Journal of Ag-
ing and Health.
Steptoe, A., Shankar, A., Demakakos, P., and Wardle, J.
(2013). Social isolation, loneliness, and all-cause mor-
tality in older men and women. Proceedings of the
National Academy of Sciences, 110(15):5797–5801.
Troje, N. F. (2002). Decomposing biological motion: A
framework for analysis and synthesis of human gait
patterns. Journal of vision, 2(5):2.
Umberson, D. and Montez, J. K. (2010). Social relation-
ships and health a flashpoint for health policy. Journal
of health and social behavior, 51(1 suppl):S54–S66.
Ustev, Y. E., Durmaz Incel, O., and Ersoy, C. (2013).
User, device and orientation independent human ac-
tivity recognition on mobile phones: challenges and
a proposal. In Proceedings of the 2013 ACM confer-
ence on Pervasive and ubiquitous computing adjunct
publication, pages 1427–1436. ACM.
Van Bel, D. T., IJsselsteijn, W. A., and de Kort, Y. A.
(2008). Interpersonal connectedness: conceptualiza-
tion and directions for a measurement instrument. In
CHI’08 extended abstracts on Human factors in com-
puting systems, pages 3129–3134. ACM.
PerceptionofHumanActivities-AMeanstoSupportConnectednessBetweentheElderlyandTheirCaregivers
199