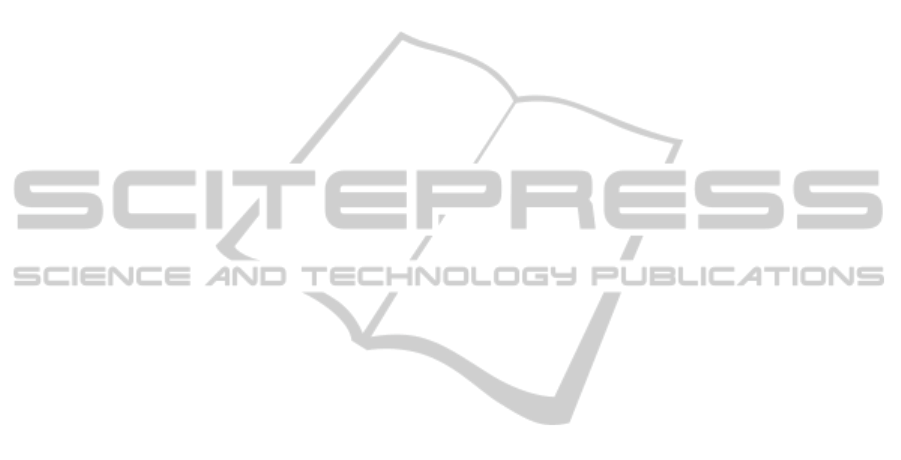
to each individual signal. Therefore, it is suggested
for further work a threshold obtained as a percentage
of the standard deviation of each signal.
DFA analysis presents higher values for both α
1
and α
2
DFA coefficients for the control group. Then,
this algorithm seems to be a good measure to reflect
neural degeneration.
MSE analysis presents higher values for the right
hand for the patients group, and higher values for the
right forearm for the control group. This algorithm
appears to be a good indicator of neural degeneration.
LZ, DFA and MSE analysis have then potential as
a quantitative test for upper and lower neural integrity
concerning ALS disease.
Classification results demonstrate to provide a
good distinction of both groups, being the combina-
tion of various algorithms with features proved to be
advantageous to improve both groups distinction ca-
pability.
REFERENCES
Almeida, M., Vig
´
ario, R., and Bioucas-Dias, J. (2011).
Phase locked matrix factorization. In Proc. of the EU-
SIPCO Conference, pages 1728–1732.
Camara, M. (2013). Coherence and phase locking dis-
ruption in electromyograms of patients with amy-
otrophic lateral sclerosis. Master’s thesis, Faculdade
de Ci
ˆ
encias e Tecnologia da Universidade Nova de
Lisboa.
Eskofier, B. M., Kraus, M., Worobets, J. T., Stefanyshyn,
D. J., and Nigg, B. M. (2012). Pattern classifica-
tion of kinematic and kinetic running data to distin-
guish gender, shod/barefoot and injury groups with
feature ranking. Computer methods in biomechanics
and biomedical engineering, 15(5):467–474.
Farmer, S. F., Gibbs, J., Halliday, D. M., Harrison, L. M.,
James, L. M., Mayston, M. J., and Stephens, J. A.
(2007). Changes in emg coherence between long and
short thumb abductor muscles during human develop-
ment. The Journal of physiology, 579(2):389–402.
Fisher, K. M., Zaaimi, B., Williams, T. L., Baker, S. N.,
and Baker, M. R. (2012). Beta-band intermuscular
coherence: a novel biomarker of upper motor neu-
ron dysfunction in motor neuron disease. Brain,
135(9):2849–2864.
Gomes, A. L. (2014). Human activity recognition with ac-
celerometry: Novel time and frequency features. Mas-
ter’s thesis, Faculdade de Ci
ˆ
encias e Tecnologia da
Universidade Nova de Lisboa.
Kaplanis, P. A., Pattichis, C. S., Zazula, D., et al.
(2010). Multiscale entropy-based approach to au-
tomated surface emg classification of neuromuscular
disorders. Medical & biological engineering & com-
puting, 48(8):773–781.
Kiernan, M. C., Vucic, S., Cheah, B. C., Turner, M. R.,
Eisen, A., Hardiman, O., Burrell, J. R., and Zoing,
M. C. (2011). Amyotrophic lateral sclerosis. The
Lancet, 377(9769):942–955.
Kim, K. S., Choi, H. H., Moon, C. S., and Mun, C. W.
(2011). Comparison of¡ i¿ k¡/i¿-nearest neighbor,
quadratic discriminant and linear discriminant analy-
sis in classification of electromyogram signals based
on the wrist-motion directions. Current Applied
Physics, 11(3):740–745.
Mitchell, J. D. and Borasio, G. D. (2007). Amyotrophic
lateral sclerosis. The lancet, 369(9578):2031–2041.
Pedregosa, F., Varoquaux, G., Gramfort, A., Michel, V.,
Thirion, B., Grisel, O., Blondel, M., Prettenhofer,
P., Weiss, R., Dubourg, V., Vanderplas, J., Passos,
A., Cournapeau, D., Brucher, M., Perrot, M., and
Duchesnay, E. (2011). Scikit-learn: Machine learning
in Python. Journal of Machine Learning Research,
12:2825–2830.
Phinyomark, A., Phukpattaranont, P., Limsakul, C., and
Phothisonothai, M. (2011). Electromyography (emg)
signal classification based on detrended fluctuation
analysis. Fluctuation and Noise Letters, 10(03):281–
301.
Poosapadi Arjunan, S. and Kumar, D. K. (2012). Compu-
tation of fractal features based on the fractal analysis
of surface electromyogram to estimate force of con-
traction of different muscles. Computer Methods in
Biomechanics and Biomedical Engineering, (ahead-
of-print):1–7.
Roy, M.-H. and Larocque, D. (2012). Robustness of ran-
dom forests for regression. Journal of Nonparametric
Statistics, 24(4):993–1006.
Talebinejad, M., Chan, A. D., and Miri, A. (2011). A
lempel–ziv complexity measure for muscle fatigue es-
timation. Journal of Electromyography and Kinesiol-
ogy, 21(2):236–241.
Wang, L.-M., Li, X.-L., Cao, C.-H., and Yuan, S.-M.
(2006). Combining decision tree and naive bayes for
classification. Knowledge-Based Systems, 19(7):511–
515.
West, B. J. (1994). Fractal physiology, volume 2. Oxford
University Press.
Zhang, X., Chen, X., Barkhaus, P. E., and Zhou, P. (2013).
Multiscale entropy analysis of different spontaneous
motor unit discharge patterns. Journal of Biomedical
and Health Informatics, 17(2).
Zhang, X., Chen, X., Li, Y., Lantz, V., Wang, K., and Yang,
J. (2011). A framework for hand gesture recognition
based on accelerometer and emg sensors. Systems,
Man and Cybernetics, Part A: Systems and Humans,
IEEE Transactions on, 41(6):1064–1076.
BIOSIGNALS2015-InternationalConferenceonBio-inspiredSystemsandSignalProcessing
234