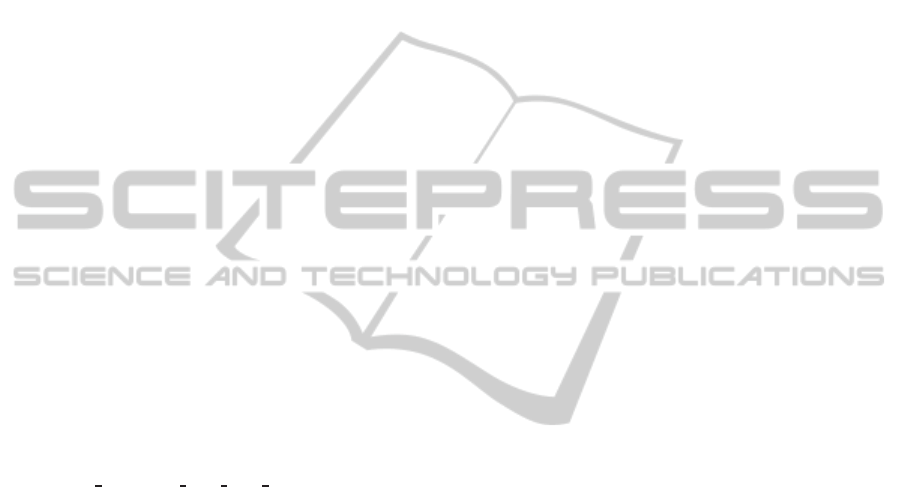
frequency of the band pass filter should be set at
around 2.0Hz.
Discriminant analysis was conducted using the
features of ERP, in order to evaluate the significance
of these, and some significant accuracy was obtained.
To generate more significant features to measure the
viewer’s state of emotion while they are viewing im-
ages of facial expressions, some additional biosignals
such as eye movements using EOGs should be con-
sidered. They will be subject of our further study.
ACKNOWLEDGEMENT
This research was partially supported by the Japan
Society for the Promotion of Science (JSPS), Grant-
in-Aid for Scientific Research (B-26282046:2014-
2016).
REFERENCES
Brainard, D. H. (1997). The Psychophysics Toolbox. Spa-
tial Vision, 10:433–436.
Ekman, P. and Friesen, W. V. (1975). Unmasking the Face:
A guide to recognizing emotions from facial clues.
Prentice-Hall, New Jersey, USA.
Guitton, C. (2010). Emotions estimation from eeg
recordings. Master’s thesis, Imperial College,
Londo, UK. http://www.academia.edu/1203149/
Emotions
Estimation from EEG Recordings.
Huang, J., Fan, J., He, W., Yu, S., Yeow, G., Sun, G., Shen,
M., Chen, W., and Wang, W. (2009). Could inten-
sity ratings of Matsumoto and Ekman’s JACFEE pic-
tures delineate basic emotions? A principal compo-
nent analysis in Chinese university students. Person-
ality and Individual Differences, 46:331–335.
Kirchner, H. and Thorpe, S. J. (2006). Ultra-rapid object de-
tection with saccadic eye movements: Visual process-
ing speed revisited. Vision Research, 46:1762–1776.
Lin, Y.-P., Wang, C.-H., Jung, T.-P., Wu, T.-L., and Jeng, S.-
K. (2010). EEG-Based emotion recognition in music
listening. IEEE Transaction on biomedical Engineer-
ing, 57(7):1798–1806.
Matsumoto, D. and Ekman, P. (1988). Japanese and Cau-
casian facial expression of emotion (JACFEE) and
neutral faces (JACNeuF). San Fransisco State Uni-
versity, San Francisco, CA, USA.
Nittono, H. (2005). Event-related potential guidebook
for psychological research. Kitaohji-shobo, Kyoto,
Japan.
Petrantonakis, P. C. and Hadjileontiadis, L. J. (2010). Emo-
tion recognition from EEG using higher order cross-
ings. IEEE Transaction on information technology in
biomedine, 14(2):186–197.
Rugg, M. D. (1997). Cognitive Neuroscience. Psychology
Press, UK.
Russell, J. A., Weiss, A., and Mendelsohn, G. A. (1989).
Affect grid: A single-item scale of pleasure and
arosal. Journal of Personality and Social Psychology,
57(3):493–502.
Shibui, S. and Shigemasu, K. (2005). A model of
two-dimensional placement of the facial expressions
of emotion. The Japanese Journal of Psychology,
76(2):113–121.
Takehara, T. and Suzuki, N. (2001). Robustness of the two-
dimensional structure of recognition of facial expres-
sion: evidence under differentt intensities of emotion-
ality. Perception and Motor Skills, 93:739–753.
Thorpe, S., Fize, D., and Marlot, C. (1996). Speed of pro-
cessing in the human visual system. Nature, 381:520–
522.
VanRullen, R. and Thorpe, S. J. (2001). The time
course of visual processing: From early perception to
decision-making. Journal of Cognitive Neuroscience,
13(4):454–461.
Yasuda, M., Dou, Z., and Nakayama, M. (2014). EEG fea-
tures of recognizing facial expressions. Technical Re-
port HCS2014-41, IEICE Technical report.
FeaturesofEvent-relatedPotentialsUsedtoRecognizeClustersofFacialExpressions
171