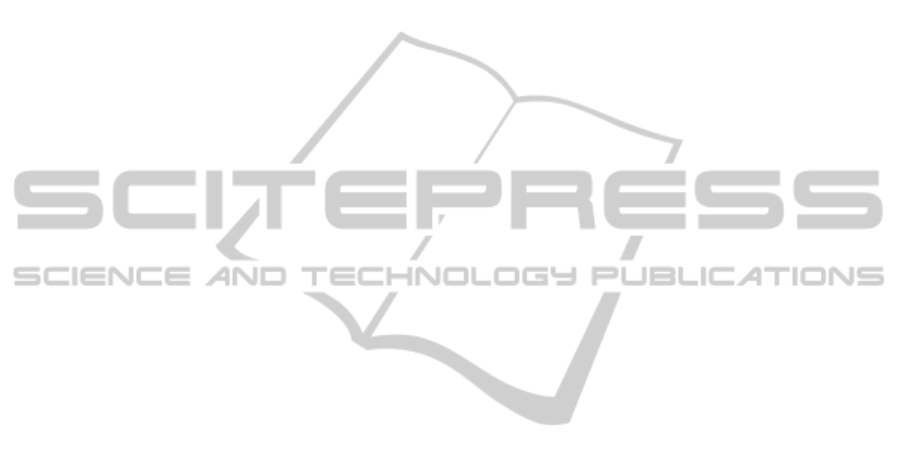
obtained. However, the same analysis for nega-
tive videos doesn’t show significant differences.
An increase in brain activity was recorded when
positive emotions are stimulated.
• The quantification of the brain activity has been
performed using GFP. As a result, an emotional
classification of the videos was made taking into
account the average number of peaks across the
subjects for each stimulus. As it was shown in
previous question, an increase in cerebral activity
occurs while the positive videos are displayed.
• Using the Zscore index obtained from the GFP, it
is possible to analyse frame by frame each video
in order to study the moments of the video where
the subject shows higher cerebral activity (key
frames). Zscore in theta and beta bands presents
different distribution of peaks, occurring at differ-
ent frames. The key frames detected for most of
the subjects were the same, showing similar pat-
terns. Celebration of goals and titles by players
and fans were the frames where the highest brain
activity was measured.
In conclusion, the football videos analysed in this
study provoked an increase in the cerebral activity in
relation to the viewing of the documentary. More-
over, during the visualization of positive videos the
subjects experimented on average an increase in cere-
bral activity higher than the experimented during the
visualization of negative videos.
In future research, observations and conclusions
of this work will be widely validated. The human be-
haviour in diverse audiovisual content will be evalu-
ated in order to understand better the emotions and
feelings processed in the brain.
ACKNOWLEDGEMENTS
This work has been possible by the collaboration
of Valencia C.F S.A.D. with i3bh/LabHuman re-
search group and partially by projects Consolider-C
(SEJ2006 14301/PSIC), “CIBER of Physiopathology
of Obesity and Nutrition, an initiative of ISCIII” and
Excellence Research Program PROMETEO (Gener-
alitat Valenciana. Conselleria de Educaci
´
on, 2008-
157).
REFERENCES
Brouwer, A.-M., Neerincx, M., Kallen, V., Van Der Leer,
L., and ten Brinke, M. (2011). Eeg alpha asymmetry,
heart rate variability and cortisol in response to virtual
reality induced stress. Journal of Cybertherapy and
Rehabilitation, 4(1):27–40.
Delorme, A. and Makeig, S. (2004). Eeglab: an open
source toolbox for analysis of single-trial eeg dynam-
ics. Journal of Neuroscience Methods, 134:9–21.
Klucharev, V., Smidts, A., and Fernandez, G. (2008). Brain
mechanisms of persuasion: How ”expert power” mod-
ulates memory and attitudes. Soc Cogn Affect Neu-
rosci, 3(4):353–366.
Lehmann, D. and Skrandies, W. (1980). Reference-free
identification of components of checkerboard-evoked
multichannel potential fields. Electroencephalogra-
phy and clinical neurophysiology, 48(6):609–621.
Mognon, A., Jovicich, J., Bruzzone, L., and Buiatti, M.
(2011). Adjust: An automatic eeg artifact detector
based on the joint use of spatial and temporal features.
Psychophysiology, 48(2):229–240.
Norhazman, H., Mohamad Zaini, N., Taib, M. N., Omar,
H. A., Jailani, R., and Lias, S. (2012). Behaviour of
eeg alpha asymmetry when stress is induced and bin-
aural is applied. In International Symposium on Com-
puter Applications and Industrial Electronics (ISCAIE
2012), pages 297–301, Kota Kinabalu, Malaysia.
Sanei, S. and Chambers, J. A. (2007). EEG Signal Process-
ing. Wiley.
Sulaiman, N., Taib, M. N., Aris, S. A. M., Hamid, N. H. A.,
Lias, S., and Murat, Z. H. (2010). Stress features
identification from eeg signals using eeg asymmetry
& spectral centroids techniques. In Biomedi-
cal Engineering and Sciences (IECBES), 2010 IEEE
EMBS Conference on, pages 417–421. IEEE.
Sulaiman, N., Taib, M. N., Lias, S., Murat, Z. H., Aris,
S. A. M., and Hamid, N. H. A. (2011). Novel meth-
ods for stress features identification using eeg signals.
International Journal of Simulation, Systems, Science
and Technology, 12(1):27–33.
Vecchiato, G., Astolfi, L., De Vico Fallani, F., Cincotti, F.,
Mattia, D., Salinari, S., Soranzo, R., and Babiloni, F.
(2010a). Changes in brain activity during the observa-
tion of tv commercials by using eeg, gsr and hr mea-
surements. Brain Topogr, 23(2):165–79.
Vecchiato, G., Astolfi, L., Tabarrini, A., Salinari, S., Mattia,
D., Cincotti, F., Bianchi, L., Sorrentino, D., Aloise, F.,
Soranzo, R., and Babiloni, F. (2010b). Eeg analysis
of the brain activity during the observation of com-
mercial, political, or public service announcements.
Comp. Int. and Neurosc.
BrainActivityQuantificationforSportAudiovisualContentVisualizationusingEEG
149