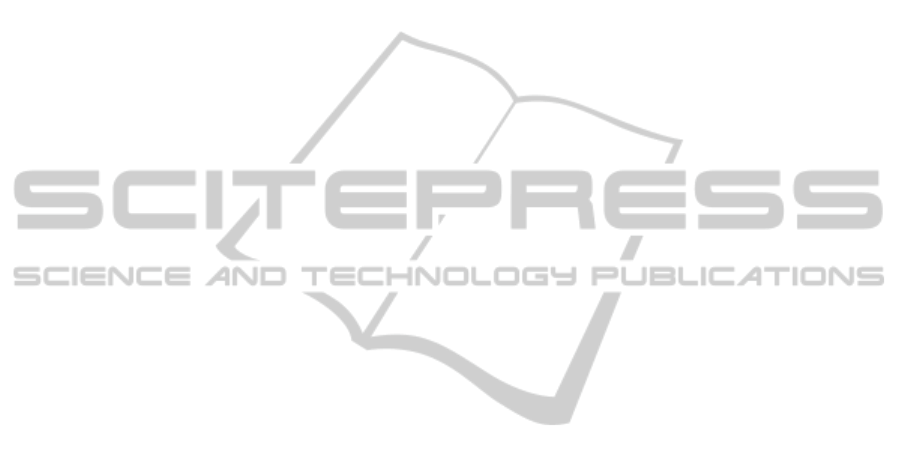
impedance tomography in a piglet model of lung
collapse, Journal of Applied Physiology, vol. 112, no.
11, pp. 225-236.
Braun, F., 2013. Systolic Time Intervals Measured by
Electrical Impedance Tomography (EIT), Swiss
Federal Institute of Technology, Zurich, Switzerland.
DOI: http://dx.doi.org/10.3929/ethz-a-009947722.
Brown, B.H., Leathard, A., Sinton, A. et al., 1992. Blood
flow imaging using electrical impedance tomography,
Clinical Physics and Physiological Measurement, vol.
13 (suppl A), pp. 175-179.
Deibele, J. M., Luepschen H. and S. Leonhardt, 2008.
Dynamic separation of pulmonary and cardiac
changes in electrical impedance tomography,
Physiological Measurement, vol. 29, no. 16, pp. S1-
S14.
Denai, M. A., Mahfouf, M., Mohamad-Samuri, S. et al.,
2010. Absolute Electrical Impedance Tomography
(aEIT) Guided Ventilation Therapy in Critical Care
Patients: Simulations and Future Trends, IEEE
Transactions on Information Technology in
Biomedicine, vol. 14, no. 13, pp. 641-649.
Fagerberg, A., Stenqvist, O. and Åneman, A., 2009.
Monitoring pulmonary perfusion by electrical
impedance tomography: an evaluation in a pig model,
Acta Anaesthesiologica Scandinavica, vol. 53, no. 12,
pp. 152-158.
Ferrario, D., Grychtol, B., Solà, J. et al., 2012. Towards
morphological thoracic EIT: Major signal sources
correspond to respective organ locations in CT, IEEE
Transactions on Biomedical Engineering, vol. 59, no.
111, pp. 3000-3008.
Frerichs, I., Hinz, J., Herrmann, P. et al., 2002. Regional
lung perfusion as determined by electrical impedance
tomography in comparison with electron beam CT
imaging, IEEE Transactions on Medical Imaging, vol.
21, no. 16, pp. 646-652.
Frerichs, I., Pulletz, S., Elke, G. et al., 2009. Assessment of
changes in distribution of lung perfusion by electrical
impedance tomography, Respiration, vol. 77, no. 13,
pp. 282-291.
Gabriel, S., Lau, R. W. and Gabriel, C., 1996. The
dielectric properties of biological tissues: III.
Parametric models for the dielectric spectrum of
tissues, Physics in Medicine and Biology, vol. 41, no.
111, pp. 2271–2293.
Grant, C. A., Pham, T., Hough, J. et al., 2011.
Measurement of ventilation and cardiac related
impedance changes with electrical impedance
tomography, Critical Care, vol. 15, no. 11, p. R37.
Grychtol, B., Lionheart, W. R. B., Bodenstein, M. et al.,
2012. Impact of Model Shape Mismatch on
Reconstruction Quality in Electrical Impedance
Tomography, IEEE Transactions on Medical Imaging,
vol. 31, no. 19, pp. 1754-1760.
Guha, S. K., Khan, M. R. and Tandon, S. N., 1973.
Electrical field distribution in the human body,
Physics in Medicine and Biology, vol. 18, no. 5, pp.
712-720.
Hellige, G., Hahn, G., 2011.
Cardiac-related impedance
changes obtained by electrical impedance
tomography: an acceptable parameter for assessment
of pulmonary perfusion?, Critical Care, vol. 15, p.
430.
Holder, D. S., 2005. Electrical impedance tomography:
methods, history and applications, Institute of Physics
Publishing. London.
Kim, D. W., Baker, L. E., Pearce, J. A. and Kim, W. K.,
1988. Origins of the impedance change in impedance
cardiography by a three-dimensional finite element
model, IEEE Transactions on Biomedical Engineering,
vol. 35, no. 112, pp. 993-1000.
Kunst, P. W. A., Vonk-Noordegraaf, A., Hoekstra, O. S. et
al., 1998. Ventilation and perfusion imaging by
electrical impedance tomography: a comparison with
radionuclide scanning, Physiological Measurement,
vol. 19, no. 14, pp. 481–490.
Leathard, A. D., Brown, B. H., Campbell, J. et al., 1994. A
comparison of ventilatory and cardiac related changes
in EIT images of normal human lungs and of lungs
with pulmonary emboli, Physiological Measurement,
vol. 15 (suppl 2A), pp. A137-A146.
Levick, J. R., 2010. An introduction to cardiovascular
physiology, Arnold. London, 5
th
edition.
Malmivuo, J. and Plonsey, R., 1995. 7: Volume source
and volume conductor, in Bioelectromagnetism:
Principles and Applications of Bioelectric and
Biomagnetic Fields, University Press. New York.
McArdle, F. J., Suggett, A. J., Brown, B. H. and Barber,
D. C., 1988. An assessment of dynamic images by
applied potential tomography for monitoring
pulmonary perfusion, Clinical Physics and
Physiological Measurement, vol. 9 (suppl A), pp. 87-
91.
Nguyen, D. T., Jin, C., Thiagalingam, A. and McEwan, A.
L., 2012. A review on electrical impedance
tomography for pulmonary perfusion imaging,
Physiological Measurement, vol. 33, no. 5, pp. 695–
706.
Smit, H. J., Vonk-Noordegraaf, A., Marcus, J. T. et al.,
2004. Determinants of pulmonary perfusion measured
by electrical impedance tomography, European
Journal of Applied Physiology, vol. 92, no. 1, pp. 45-
49.
Vonk-Noordegraaf, A., Kunst, P. W. A., Janse, A. et al.,
1998. Pulmonary perfusion measured by means of
electrical impedance tomography, Physiological
Measurement, vol. 19, no. 12, pp. 263–273.
Vonk-Noordegraaf, A., Janse, A., Marcus, J. T. et al.,
2000. Determination of stroke volume by means of
electrical impedance tomography, Physiological
Measurement, vol. 21, no. 12, pp. 285–293.
BIOSIGNALS2014-InternationalConferenceonBio-inspiredSystemsandSignalProcessing
34