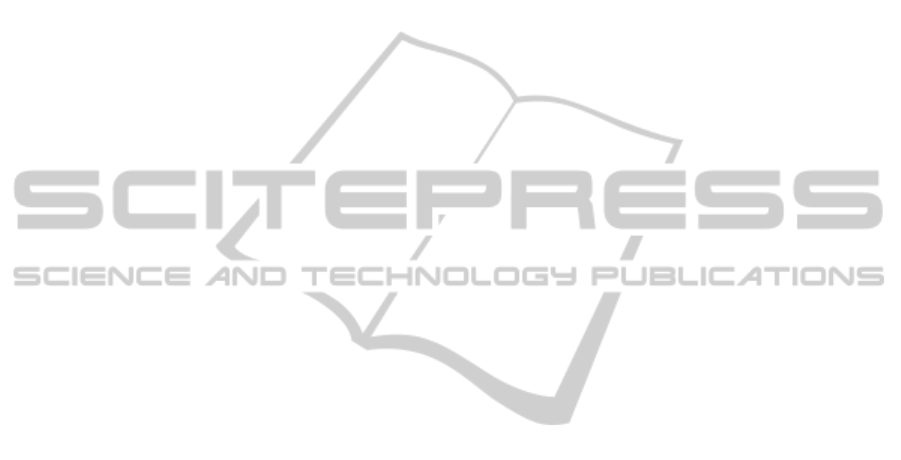
method is capable of detecting an individual PV
module with a loss of approximately 15% in power
output. This result also shows that a module where
the level of power outputs begins to decline can be
detected as potentially malfunctioning.
6 DISCUSSION
In this experiment, we only utilized the spatial
correlation information in comparing the power
output with the maximum power for the entire
modules and the power levels for each individual
module. However, there are various kinds of spatial
correlation information, such as correlations
between neighbouring module groups or between
individual modules. In particular, modules within
the same string tend to be highly correlated because
they mutually influence voltages within the same
current. The attainment of better malfunction-
detection performance by utilizing more complex
forms of spatial correlation information will be
tackled in future research.
The present experiment focused on a malfunction
where the level of power output suddenly decreases,
but there are other forms of malfunctioning, such as
gradual decreases in output power. In those
situations, it is not possible to detect the malfunction
until a certain level of decrease is reached. But once
it has been reached, our method can detect such
malfunctions.
In the present paper, we have focused only on
power, which is the representative value of output
within the solar energy data. However, we may
expect even faster and more accurate malfunction
detection performance by using other values, such as
voltage, current, and temperature. We will continue
to consider the utilization of such values for the
detection of malfunctioning PV modules.
Our method can be applied to mega-scale solar
systems by changing the unit of measurement from
the single module to a string of modules. In the
future, we are planning to apply the method to a
mega-scale solar system.
7 CONCLUSIONS
In this study, we have developed a method of
malfunction detection using a monitoring system of
the individual PV modules. The method makes it
possible to detect malfunctions in their early stages
in terms of slight declines in the levels of power
output from a PV module, which it has been very
difficult to be aware of previously. We confirmed
the effectiveness of the method through a detection
experiment for a simulated malfunction using actual
data from our monitoring system for individual PV
modules. In the future, we plan to explore the
application of our method to mega-scale solar
systems.
ACKNOWLEDGEMENTS
We would like to thank Prof. F. Kano in Oyama
National College of Technology for valuable
discussions.
REFERENCES
Tan, D. and Seng A. K., 2011. Handbook for solar
photovoltaic (PV) systems, Energy Market Authority.
Chouder, A. and Silvestre, S., 2010. Automatic
supervision and fault detection of PV systems based
on power losses analysis. In Energy Conversion and
Management, 51, pp. 1929-1937, Elsevier.
Polo, F. A. O., del Rosario, J. J. A. and Garcia, G. C.,
2010. Supervisory control and automatic failure detec-
tion in grid-connected photovoltaic systems. In N.
Garcia-Pedrajas et al. (Eds.): IEA/AIE 2010, Part I,
LNAI 6096, pp. 458-467, Springer.
Stettler, S., Toggweiler, P. and Remund, J., 2006. SPYCE:
satellite photovoltaic yield control and evaluation. In
21st European Photovoltaic Solar Energy Conference,
pp. 2613-2616.
Phoenix Contact GmbH & Co. KG, 2012. String
monitoring in photovoltaic systems, [online]
Available: http://www.phoenixcontact.com/global/
signal-level-matching/242_66017.htm.
Onamba Co., Ltd., 2010. Photovoltaic intelligent monitor-
ing system (in Japanese). [online] Available:
http://www.onamba.co.jp/pdf/monitor_catalog.pdf.
Tigo Energy, Inc., 2009. Tigo Energy MaxiManager soft-
ware data sheet. [online] Available: http://
www.tigoenergy.com/sites/default/files/attachments/
software.pdf.
Nosato, H., Kasai, Y., Takahashi, E. and Murakawa, M.,
2012. A very low-cost low-ffrequency PLC system
based on DS-CDMA for DC power lines. In 16th
IEEE International Symposium on Power Line
Communications and Its Applications (ISPLC2012).
pp. 398-403, IEEE.
Nosato, H., Kasai, Y., Iwata, M., Takahashi, E. and Mura-
kawa, M., 2013. The development of the individual
photovoltaic module monitoring system using power
line communication. In The Transactions of the
Institute of Electronics, Information and
Communication Engineers (in Japanese), J96-D (3) (in
press), IEICE.
SMARTGREENS2013-2ndInternationalConferenceonSmartGridsandGreenITSystems
184