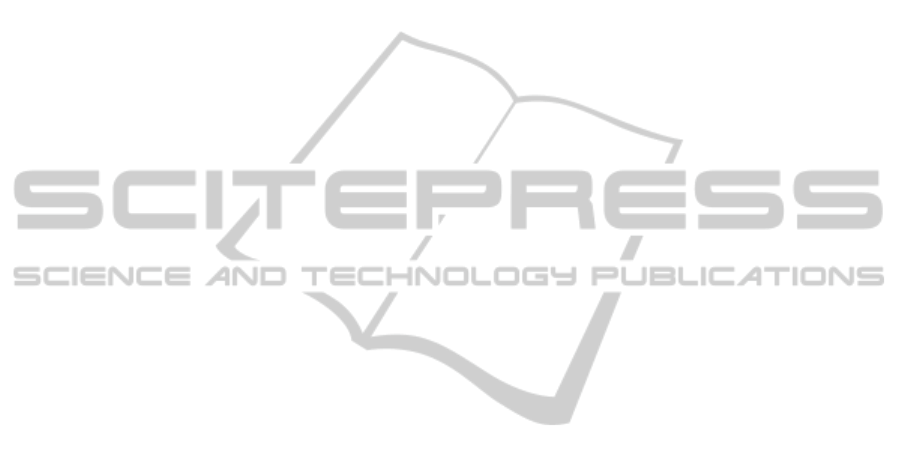
select distinct ?s1 where {
?s1 a <#policy_artifact> ?s2 a <#person> .
?s3 a <#human_activity> ?s4 a <#planning> .
?s1 <#related_to> ?s3 .
?s3 <#has_participant> ?s2 .
?s4 <#has_participant> ?s2 .
?s4 <#produces> ?s1
}
A description logics reasoner could then determine
that this query matches the semantic graph shown in
figure 1 because “Socializing” is described in the
ontology to be a subclass of “human activity”.
Consider adding a query constraint specifying
that the persons involved in both the planning and
the activities of the intervention were the focus of
the problem type addressed by the intervention.
Although the problem type “need for engaging the
staff” exists in the graph, it applies to persons
involved in the planning of the intervention but not
in the activities of the intervention. Thus, the query
would no longer match with the semantic graph.
3 FUTURE DIRECTIONS
Future work on this knowledge base will focus on
developing effective applications in areas that are
most likely to be beneficial to the envisaged users.
Natural language generation can be used to generate
accurate representations of the semantic graphs in
any language that is handled by the generator, as
shown in figure 2. Knowledge mining techniques
can be used to extract common semantic motifs from
the knowledge base on what kinds of interventions
are most effective in what kinds of social contexts.
Obtaining feedback from potential users of the
knowledge base will be critical in guiding the
development of these applications. For this purpose,
we hope to establish a “community of practice”
through the Platinum Concept Network in Japan and
other entities around the world, such as the United
Nations HABITAT program (www.unhabitat.org).
Feedback from these test users will also help to
identify what modifications to the knowledge base
schema are needed. Under the theme of “social
entrepreneurship”, a new class of NPOs is emerging
that shares some of the flexibility for experimental
trial-and-error provided by venture capitalists
(Tanimoto 2008). These NPOs may be valuable
sources for knowledge on what works in addressing
specific social issues in specific social contexts.
System development is focused in the human-
computer interface. We are testing different methods
for accessing the knowledge base, one of which is
based on semantic similarity calculated between
cities using semantic attributes from DBpedia (Guo
and Kraines 2010). We are also using knowledge
mining and natural language processing techniques
to further assist researchers and social entrepreneurs
to create semantic graphs that accurately express the
knowledge that they want to share.
REFERENCES
Abrahamse, W., Wokje, A., Steg, L., Vlek, C., and
Rothengatter, T. 2005. A review of intervention
studies aimed at household energy conservation.
Journal of Environ Psychology 25(3): pp. 273-291.
Cattan, M., White, M., Bond, J. and Learmouth, A. 2005.
Preventing social isolation and loneliness among older
people: a systematic review of health promotion
interventions. Ageing & Society 25: pp. 41-67.
Dwyer, W. O., Leeming, F.C., Cobern, M.K., Porter, B.E.,
and Jackson, J. M. 1993. Critical review of behavioral
interventions to preserve the environment: Research
since 1980. Environ and Behavior 25: pp. 485-505.
Greenberg, D. H. and Shroder. M. 2004. Digest of Social
Experiments Third Edition. The Urban Institute Press.
Guo, W. and Kraines S.B., 2008. Explicit scientific
knowledge comparison based on semantic description
matching. ASIST 2008, Columbus, Ohio.
Guo W., S. B. Kraines. 2010. Enriching city entities in the
EKOSS failure cases knowledge base with linked open
data. Proc 6th Intl Conf on Next Gen Web Services
Practices, Nov 23-25, 2010, Gwalior, India, pp: 58-63
Hogan, B. E., Linden, W., and Najarian, B. 2002. Social
support interventions Do they work? Clinical
Psychology Review 22: pp. 381-440.
Kraines, S. B. and Guo, W., 2011. A system for ontology-
based sharing of expert knowledge in sustainability
science. Data Science Journal, 9: 107-123.
McKenzie-Mohr, D. 1996. Promoting a Sustainable
Future: An Introduction to Community-based Social
Marketing. http://nrtee-trnee.ca/wp-content/uploads/
2011/08/promoting-sustainable-future.pdf
Orr, L. L. 1998. Social experimentation: evaluating public
programs with experimental methods. Office of the
Assistant Secretary for Planning and Evaluation, U.S.
Department of Health and Human Services.
Platinum Concept Network. 2012. www.platinum-
network.jp (Japanese only)
Tanimoto, K. 2008. A Conceptual Framework of Social
Entrepreneurship and Social Innovation Cluster: A
Preliminary Study. Hitotsubashi Journal of Commerce
and Management, 42(1): pp. 1-16.
Takeuchi, K. and Komiyama, H., 2006. Sustainability
science: building a new discipline. Sust Sci, 1(1): 1-6.
Urban Reformation Program for Realization of Bright
Low Carbon Society. 2012. http://low-carbon.k.u-
tokyo.ac.jp/en/index.html
DevelopmentofaKnowledgeBaseforSocialExperimentation
167