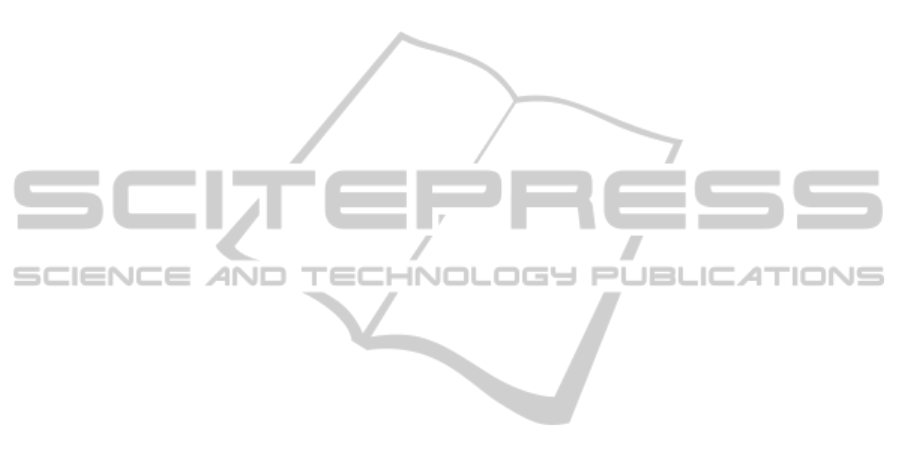
39. Reisch T., Ebner-Priemer U. W., Tschacher, W., Bohus, M., Linehan, M. M.: Sequences of
Emotions in Patients with Borderline Personality Disorder. Acta Psychiatrica Scandinavica
118 (2008) 42–48.
40. Ebner-Priemer, U. W., Kuo, J., Schlotz, W., Kleindienst, N., Rosenthal, M. Z., Detterer, L.,
Linehan, M. M., Bohus, M.: Distress and Affective Dysregulation in Patients with Borderline
Personality Disorder: A Psychophysiological Ambulatory Monitoring Study. The Journal of
Nervous and Mental Disease 196 (2008) 314–320
41. Glaser, J.-P., van Os, J., Mengelers, R., Myin-Germeys, I.: A Momentary Assessment Study
of the Reputed Emotional Phenotype Associated with Borderline Personality Disorder. Psy-
chological Medicine 38 (2008) 1231–1239
42. Glaser, J.-P., van Os, J., Thewissen, V., Myin-Germeys, I.: Psychotic Reactivity in Borderline
Personality Disorder. Acta Psychiatrica Scandinavica 121 (2010) 125–134
43. Husky, M. M., Gindre, C., Mazure, C. M., Brebant, C., Nolen-Hoeksema, S., Sanacora, G.,
Swendsen, J.: Computerized Ambulatory Monitoring in Mood Disorders: Feasibility, Com-
pliance, and Reactivity. Psychiatry Research 178 (2010) 440–442
44. Links, P. S., Eynan, R., Heisel, M. J., Barr, A., Korzekwa, M., McMain, S., Ball, J. S.: Af-
fective Instability and Suicidal Ideation and Behavior in Patients with Borderline Personality
Disorder. Journal of Personality Disorders 21 (2007) 72–86
45. Links, P. S., Eynan, R., Heisel, M., Nisenbaum, R.: Elements of Affective Instability As-
sociated with Suicidal Behavior in Patients with Borderline Personality Disorder. Canadian
Journal of Psychiatry 53 (2008) 112–116
46. Nisenbaum, R., Links, P.S., Eynan, R., Heisel, M.J.: Variability and Predictors of Nega-
tive Mood Intensity in Patients with Borderline Personality Disorder and Recurrent Suicidal
Behavior: Multilevel Analyses Applied to Experience Sampling Methodology. Journal of
Abnormal Psychology 119 (2010) 433–439
47. Mokros, H. B.: Communication and Psychiatric Diagnosis: Tales of Depressive Moods from
Two Contexts. Health Communication 5 (1993) 113–127
48. Myin-Germeys, I., Peeters, F., Havermans, R., Nicolson, N. A., deVries, M. W., Delespaul,
P., van Os, J.: Emotional Reactivity to Daily Life Stress in Psychosis and Affective Disorder:
An Experience Sampling Study. Acta Psychiatrica Scandinavica 107 (2003) 124–131
49. Havermans, R., Nicolson, N. A., deVries, M. W.: Daily Hassles, Uplifts, and Time Use in
Individuals with Bipolar Disorder in Remission. The Journal of Nervous and Mental Disease
195 745–751
50. Havermans, R., Nicolson, N. A., Berkhof, J., deVries, M. W.: Mood Reactivity to Daily
Events in Patients with Remitted Bipolar Disorder. Psychiatry Research 179 47–52
51. Peeters, F., Nicolson, N. A., Berkhof, J., Delespaul, P., deVries, M.: Effects of Daily Events
on Mood States in Major Depressive Disorder. Journal of Abnormal Psychiatry 112 (2003)
203–211
52. Peeters, F., Nicolson, N. A., Berkhof, J.: Cortisol Responses to Daily Events in Major De-
pressive Disorder. Psychosomatic Medicine 65(2003) 836–841
53. Peeters, F., Berkhof, J., Delespaul, P., Rottenberg, J., Nicolson, N. A.: Diurnal Mood Varia-
tion in Major Depressive Disorder. Emotion 6 (2006) 383–391
54. Peeters, F., Berkhof, J., Rottenberg, J., Nicolson, N. A.: Ambulatory Emotional Reactivity to
Negative Daily Life Events Predicts Remission from Major Depressive Disorder. Behavior
Research and Therapy 48 (2010) 754–760
55. Putnam, K. M., McSweeney, L. B.: Depressive Symptoms and Baseline Prefrontal EEG Al-
pha Activity: A Study Utilizing Ecological Momentary Assessment. Biological Psychiatry
77 (2008) 237-240
56. Stetler, C., Dickerson, S. S., Miller, G. E.: Uncoupling of Social Zeitgebers and Diurnal
Cortisol Secretion in Clinical Depression. Psychoneuroendocrinology 29 (2004) 1250–1259
15