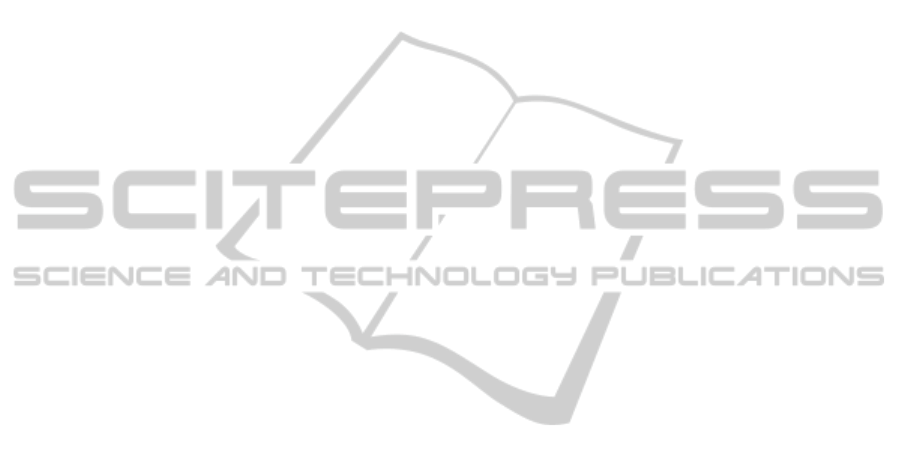
primary communication (Social) aspect. On the
surface, this would lead us to say that inherently,
mobile devices are visual instruments. However, this
perhaps leads to wondering about whether other
learning modes can be facilitated. For instance, aural
learners might be more responsive to audio.
Another trend we observed was that the personal
dimension within the Learner aspect was not
addressed. The fact that mobile devices are
considered personal devices means that we have the
opportunity to provide experiences that are highly
configured to meet the needs of the individual. In
addition, writing and speaking/listening found
limited support. Considering that these dimensions
are related to interactive communication skills, this
perhaps means that m-learning approaches are ripe
for addressing these skills.
Our primary goal in developing the Augmented
FRAME evaluation approach was to assist in
understanding the current state of m-learning. We
have found other evaluation frameworks to be
difficult to use for quickly sizing up approaches as
well as for looking at a big picture view of the field.
In order to further validate our approach, we intend
to expand the number of approaches that we
catalogue. In doing so, we hope to identify whether
some of the initial trends we have observed are true
of the field.
4 CONCLUSIONS
Mobile learning has received an influx of energy
with the release of mobile technology that has
offered a significant bump in utility. Features such
as GPS, cameras, accelerometers, magnetometers,
and other capabilities believed to be only wishful
thinking during the first generation of PDA in the
early 2000’s are now commonplace. As more
educational institutions move towards using m-
learning, effective tools that assist educators in
evaluating and selecting appropriate m-learning
strategies are needed. In this paper, we described the
Augmented FRAME evaluation framework based on
the FRAME evaluation model by Koole (2009).
In order to further validate our approach, we will
be focusing on building a larger catalogue of m-
learning techniques, with the intention of studying
trends as well as determining whether the
dimensions we have identified are sufficient.
Ultimately, our work is focused on using the
framework to inform policy makers about methods
to use by providing information about potential
learning outcomes that are relevant for m-learning.
REFERENCES
Cabrera, J. S., Frutos, H. M., Stoica, A. G., Avouris, N.,
Dimitriadis, Y., Fiotakis, G., & Liveri, K.D. (2005).
Mystery in the museum: collaborative learning
activities using handheld devices. Proc. of the 2005
ACM Mobile HCI Conference.
Corbell, J. R. & Valdes-Corbell, M. E. (2007). Are you
ready for mobile learning? EDUCAUSE Quarterly
Magazine, Vol 30, No 2.
Hagen, P., Robertson, T., Kan, M., & Sadler, K. (2005).
Emerging research methods for understanding mobile
technology use. Proc. of OZCHI 2005.
Huizenga, J., Admiraal, W., Akkerman, S., & ten Dam, G.
(2009). Mobile game-based learning in secondary
education: engagement, motivation, and learning in a
mobile city game. Journal of Computer Assisted
Learning, 25, 332-344.
Koole, M. L. (2009). A model for framing mobile
learning. In M. Ally (Ed.), Empowering Learners and
Educators with Mobile Learning. Athabasca, AB:
Athabasca University Press, 25-47.
Norris, C. & Soloway, E. (2004). Envisioning the
handheld-centric classroom. Journal of Educational
Computing Research, 30(4), 281-294.
Reckles, D. (2007). Lessons in wireless for K-12 schools.
Aruba Networks Whitepaper.
Schwabe, G. & Goth, C. (2005). Mobile learning with a
mobile game: design and motivational effects. Journal
of Computer Assisted Learning, 21, 204-216.
Sharples, M., Corlett, D., & Westmancott, O. (2002). The
design and implementation of a mobile learning
resource. Personal and Ubiquitous Computing, 6, 220-
234, Springer-Verlag.
Shen, R., Wang M., & Pan, X. (2008). Increasing
interactivity in blended classrooms through a cutting-
edge mobile learning system. British Journal of
Educational Technology, 39(6), 1073-1086.
Sung, Y.-T., Chang, K.-E., Lee, Y.-H., & Yu, W.-C.
(2008). Effects of a Mobile Electronic Guidebook on
Visitors’ Attention and Visiting Behaviors.
Educational Technology & Society, 11 (2), 67-80.
Tatar, D., Roschelle, J., Vahey, P. & Penuel, W. R. (2003).
Handhelds go to school: Lessons Learned. IEEE
Computer, September, 30-37.
Traxler, J. (2007). Defining, Discussing and Evaluating
Mobile Learning: the moving finger writes and having
writ… The Int’l Review of Research in Open and
Distance Learning, Vol 8, No 2, ISSN: 1492-3831.
van t’ Hooft, M. & McNeal T. (2010). Mobile Phones for
Mobile Learning: The Geo-Historian Project. Proc. of
the American Educational Research Assoc. Conf.
Zurita, G. & Nussbaum, M. (2004). Computer supported
collaborative learning using wirelessly interconnected
handheld computers. Computers & Education, 42,
289-314, Elsevier Publishers.
AN EVALUATION FRAMEWORK FOR M-LEARNING
333