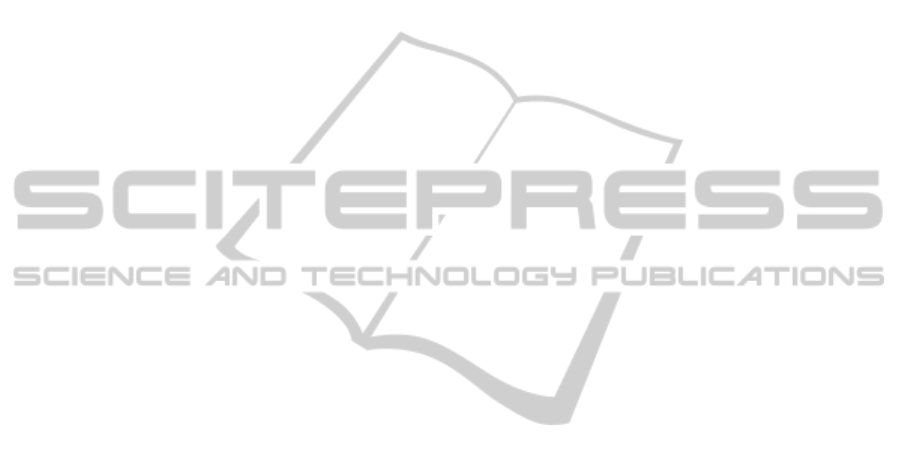
3.2 VDF Model and the Knowledge
Spiral
The VDF model can be used as a tool in process
innovation to increase the body of knowledge in the
organisation in line with the steps outlined in the
knowledge spiral:
• Socialisation (Tacit to Tacit) – VMEA allows
employees from several departments to
disseminate their knowledge to the others using
brainstorming sessions
• Externalisation (Tacit to Explicit) – The
VMEA then documents this knowledge into a
form which can be used. The VMEA uses a
structured method to calculate the greatest
causes of problems in a process and this is
documented and fed into the DOE and FEA.
• Combination (Explicit to Explicit) – In the
VDF process knowledge is combined from a
variety of explicit processes. The DOE uses
the results of the VMEA to concentrate on the
factors that are the greatest cause of process
problems and to determine the effect on the
process of altering these factors at different
levels. The FEA uses the knowledge obtained
from the VMEA and DOE to fine tune the
process and to produce process behaviour
predictions. The FEA results will be compared
and evaluated against the results of the
experimental DOE and the predictions will be
validated. Process factors that were undetected
by the DOE will be found through the FEA
analysis, a complete body of knowledge of the
process will be produced.
• Internalisation (explicit to tacit) – The results
from the DOE and FEA are disseminated to the
original brainstorming group in a final VMEA
using the findings of the experiments and
analysis and through discussion. This
internalises the knowledge within the minds of
the individuals so they can use it in their work
3.3 The VDF Model in Operation –
Case Study
The engineering company in this case study is a
medical company which presented itself with a
product failure due to the unknown causes during
the fabrication process.
Due to confidentiality issues, the company cannot be
named, as well as their product and fabrication
process. The names will be kept confidential but the
procedure will be explained in detail. To investigate
the process and the root cause of the product’s
failure the proposed approach was the VDF model.
The investigation started off with a VMEA
brainstorming session which allowed employees
from several departments (technicians, design
engineers, managerial team, quality department etc)
to disseminate their knowledge to one another,
approach that encompasses the tacit- to- tacit aspect
of the ‘Knowledge Spiral’ model.
Then the knowledge in the minds of the team
involved in the process was transformed into explicit
knowledge through the VMEA document which
outlines the tacit to explicit feature of the
Knowledge Spiral model.
Using the VMEA structured method and the
ranking algorithms proposed by Johansson et al.
(2006), the greatest causes of variation in the process
that affected the failure of the product were
identified and documented as shown in Table 1
below. A Variation Risk Priority Number (VRPN)
was calculated which computed the effect of each
process factor on the failure of the product and
identified the process factor that needed to be
investigated further. The highest the total VRPN
number - the greater the influence of that factor on
the product failure.
Initially it was thought that Factor 1 was the
greatest cause of variation on the product but from
Table 1 it can be seen that the calculated highest
VRPN total number (1730396) corresponded to the
Sub-KPC Factor 7. It was concluded that the Factor
7 process characteristic, by its variation, had the
greatest influence on the product failure.
However the method above only provides an
indication of the factors with the greatest effect on
process variation that could ultimately affect the
product failure but it cannot show how these factors
actually impact on the process itself. Therefore
more in depth explicit analyses are needed.
The VDF model then adds a combination of two
engineering methods - DOE and FEA, which
enhances the company’s knowledge and through its
explicit to explicit approach outlined in the
knowledge spiral in Section 2 of this paper the
model creates efficient practical paths to innovation.
The engineering knowledge captured through the
VMEA brain storming session and the data provided
by the VMEA table above acted as feeds for the
remaining two explicit elements of the VDF model:
the DOE and the FEA.
The DOE was performed on the process stage
named Factor 7 to determine the process optimum
running parameters and the most suitable
KMIS 2010 - International Conference on Knowledge Management and Information Sharing
344